Tight Query Complexity Lower Bounds for PCA via Finite Sample Deformed Wigner Law
STOC '18: Symposium on Theory of Computing Los Angeles CA USA June, 2018๏ผ2018๏ผ
ๆ่ฆ
We prove a query complexity lower bound for approximating the top r dimensional eigenspace of a matrix. We consider an oracle model where, given a symmetric matrix ๐โโ^d ร d, an algorithm ๐ ๐
๐ is allowed to make ๐ณ exact queries of the form ๐^(i) = ๐๐^(i) for i in {1,...,๐ณ}, where ๐^(i) is drawn from a distribution which depends arbitrarily on the past queries and measurements {๐^(j),๐^(i)}_1 โค j โค i-1. We show that for every ๐๐๐โ (0,1/2], there exists a distribution over matrices ๐ for which 1) gap_r(๐) = ฮฉ(๐๐๐) (where gap_r(๐) is the normalized gap between the r and r+1-st largest-magnitude eigenvector of ๐), and 2) any algorithm ๐ ๐
๐ which takes fewer than constรr log d/โ(๐๐๐) queries fails (with overwhelming probability) to identity a matrix ๐ตโโ^d ร r with orthonormal columns for which โจ๐ต, ๐๐ตโฉโฅ (1 - constร๐๐๐)โ_i=1^r ฮป_i(๐). Our bound requires only that d is a small polynomial in 1/๐๐๐ and r, and matches the upper bounds of Musco and Musco '15. Moreover, it establishes a strict separation between convex optimization and randomized, "strict-saddle" non-convex optimization of which PCA is a canonical example: in the former, first-order methods can have dimension-free iteration complexity, whereas in PCA, the iteration complexity of gradient-based methods must necessarily grow with the dimension.
ๆดๅคๆฅ็่ฏๆ
ๅ
ณ้ฎ่ฏ
Lower Bounds,Query Complexity,PCA,Optimization,Random Matrix Theory
AI ็่งฃ่ฎบๆ
ๆบฏๆบๆ
ๆ ทไพ
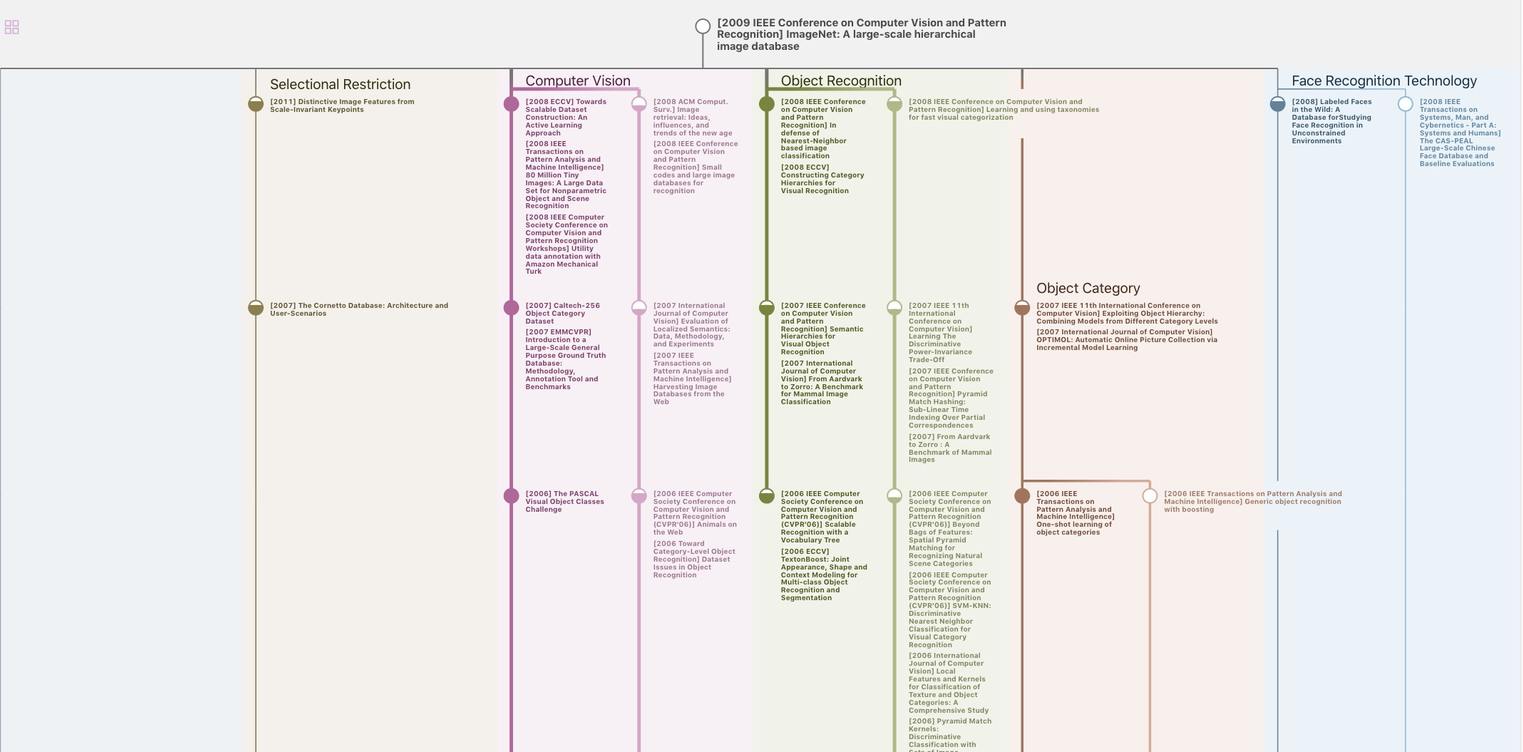
็ๆๆบฏๆบๆ ๏ผ็ ็ฉถ่ฎบๆๅๅฑ่็ป
Chat Paper
ๆญฃๅจ็ๆ่ฎบๆๆ่ฆ