SqueezeNext: Hardware-Aware Neural Network Design
2018 IEEE/CVF Conference on Computer Vision and Pattern Recognition Workshops (CVPRW)(2018)
摘要
One of the main barriers for deploying neural networks on embedded systems has been large memory and power consumption of existing neural networks. In this work, we introduce SqueezeNext, a new family of neural network architectures whose design was guided by considering previous architectures such as SqueezeNet, as well as by simulation results on a neural network accelerator. This new network is able to match AlexNet's accuracy on the ImageNet benchmark with $112\times$ fewer parameters, and one of its deeper variants is able to achieve VGG-19 accuracy with only 4.4 Million parameters, ($31\times$ smaller than VGG-19). SqueezeNext also achieves better top-5 classification accuracy with $1.3\times$ fewer parameters as compared to MobileNet, but avoids using depthwise-separable convolutions that are inefficient on some mobile processor platforms. This wide range of accuracy gives the user the ability to make speed-accuracy tradeoffs, depending on the available resources on the target hardware. Using hardware simulation results for power and inference speed on an embedded system has guided us to design variations of the baseline model that are $2.59\times$/$8.26\times$ faster and $2.25\times$/$7.5\times$ more energy efficient as compared to SqueezeNet/AlexNet without any accuracy degradation.
更多查看译文
关键词
neural network architectures,AlexNet,VGG-19,neural network accelerator,neural networks,hardware-aware neural network design,embedded system,hardware simulation results,speed-accuracy tradeoffs,SqueezeNext
AI 理解论文
溯源树
样例
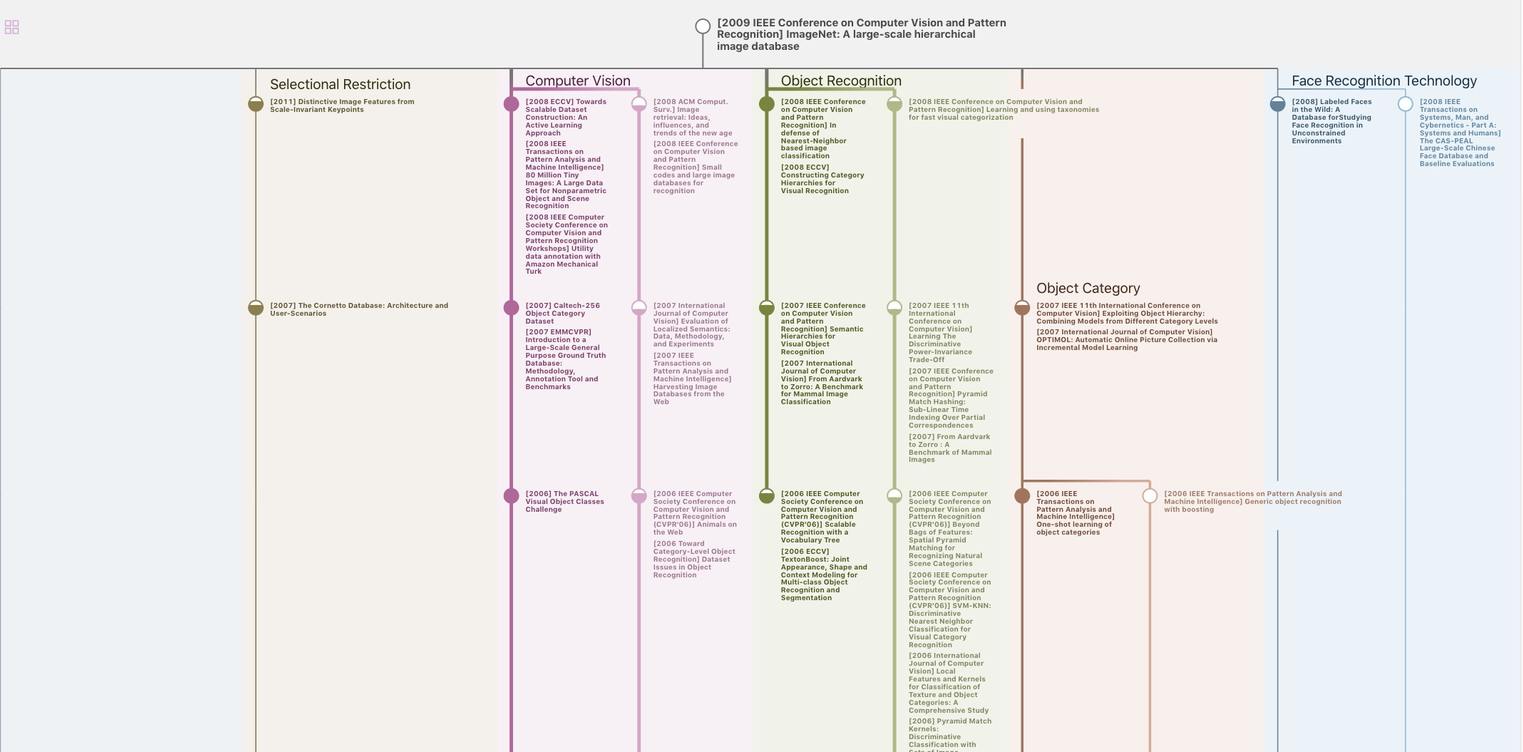
生成溯源树,研究论文发展脉络
Chat Paper
正在生成论文摘要