Semi-Supervised Algorithms for Approximately Optimal and Accurate Clustering
ICALP(2018)
摘要
We study k-means clustering in a semi-supervised setting. Given an oracle that returns whether two given points belong to the same cluster in a fixed optimal clustering, we investigate the following question: how many oracle queries are sufficient to efficiently recover a clustering that, with probability at least (1 - δ), simultaneously has a cost of at most (1 + ϵ) times the optimal cost and an accuracy of at least (1 - ϵ)? We show how to achieve such a clustering on n points with O((k^2 log n) · m(Q, ϵ^4, δ / (klog n))) oracle queries, when the k clusters can be learned with an ϵ' error and a failure probability δ' using m(Q, ϵ',δ') labeled samples in the supervised setting, where Q is the set of candidate cluster centers. We show that m(Q, ϵ', δ') is small both for k-means instances in Euclidean space and for those in finite metric spaces. We further show that, for the Euclidean k-means instances, we can avoid the dependency on n in the query complexity at the expense of an increased dependency on k: specifically, we give a slightly more involved algorithm that uses O(k^4/(ϵ^2 δ) + (k^9/ϵ^4) log(1/δ) + k · m(ℝ^r, ϵ^4/k, δ)) oracle queries. We also show that the number of queries needed for (1 - ϵ)-accuracy in Euclidean k-means must linearly depend on the dimension of the underlying Euclidean space, and for finite metric space k-means, we show that it must at least be logarithmic in the number of candidate centers. This shows that our query complexities capture the right dependencies on the respective parameters.
更多查看译文
AI 理解论文
溯源树
样例
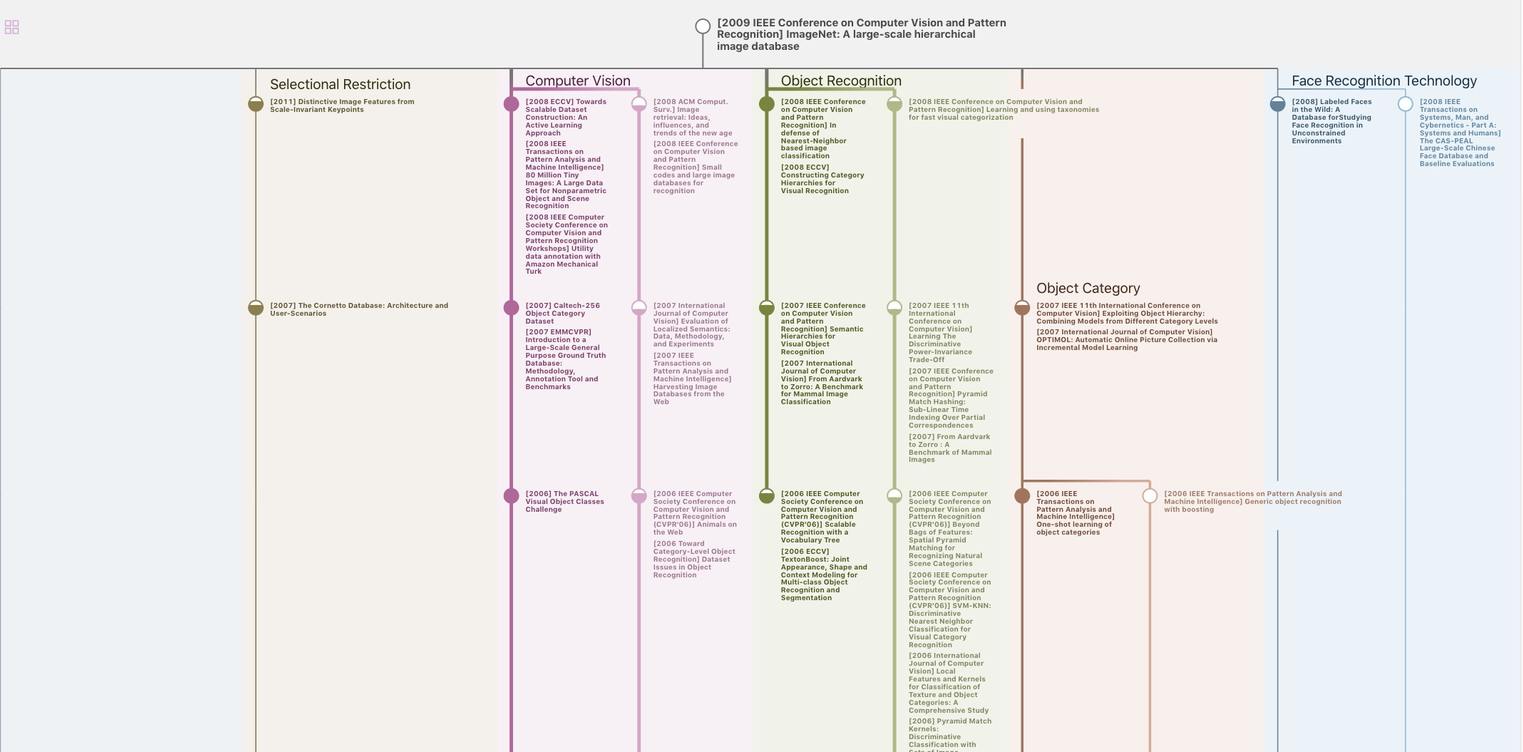
生成溯源树,研究论文发展脉络
Chat Paper
正在生成论文摘要