Understanding Image Impressiveness Inspired By Instantaneous Human Perceptual Cues
AAAI'18/IAAI'18/EAAI'18: Proceedings of the Thirty-Second AAAI Conference on Artificial Intelligence and Thirtieth Innovative Applications of Artificial Intelligence Conference and Eighth AAAI Symposium on Educational Advances in Artificial Intelligence(2018)
摘要
With the explosion of visual information nowadays, millions of digital images are available to the users. How to efficiently explore a large set of images and retrieve useful information thus becomes extremely important. Unfortunately only some of the images can impress the user at first glance. Others that make little sense in human perception are often discarded, while still costing valuable time and space. Therefore, it is significant to identify these two kinds of images for relieving the load of online repositories and accelerating information retrieval process. However, most of the existing image properties, e.g., memorability and popularity, are based on repeated human interactions, which limit the research and application of evaluating image quality in terms of instantaneous impression. In this paper, we propose a novel image property, called impressiveness, that measures how images impress people with a short-term contact. This is based on an impression-driven model inspired by a number of important human perceptual cues. To achieve this, we first collect three datasets in various domains, which are labeled according to the instantaneous sensation of the annotators. Then we investigate the impressiveness property via six established human perceptual cues as well as the corresponding features from pixel to semantic levels. Sequentially, we verify the consistency of the impressiveness which can be quantitatively measured by multiple visual representations, and evaluate their latent relationships. Finally, we apply the proposed impressiveness property to rank the images for an efficient image recommendation system.
更多查看译文
AI 理解论文
溯源树
样例
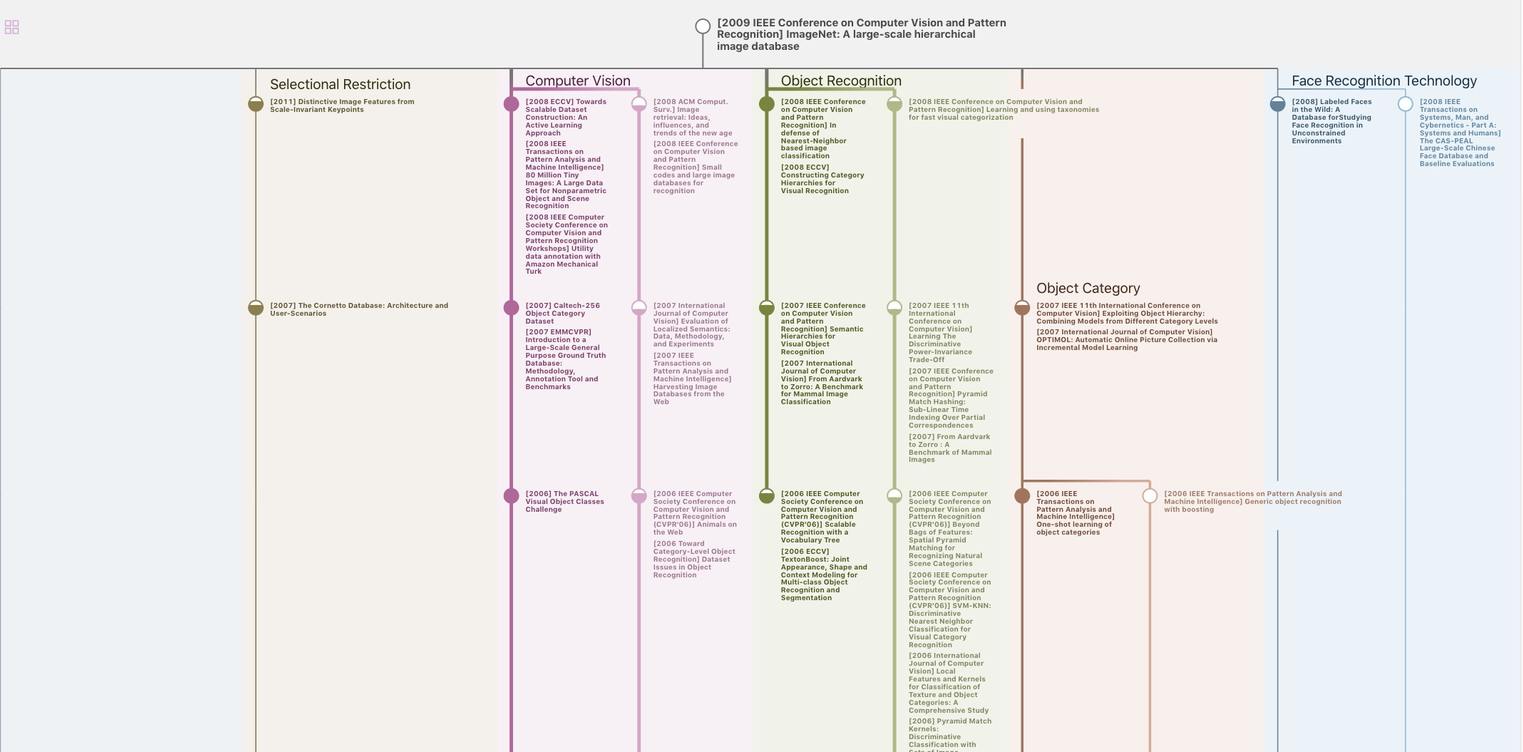
生成溯源树,研究论文发展脉络
Chat Paper
正在生成论文摘要