Abnormality detection using deep neural networks with robust quasi-norm autoencoding and semi-supervised learning
2018 IEEE 15TH INTERNATIONAL SYMPOSIUM ON BIOMEDICAL IMAGING (ISBI 2018)(2018)
摘要
Abnormality detection in biomedical images is a one-class classification problem, where methods learn a statistical model to characterize the inlier class using training data solely from the inlier class. Typical methods (i) need well-curated training data and (ii) have formulations that are unable to utilize expert feedback through (a small amount of) labeled outliers. In contrast, we propose a novel deep neural network framework that (i) is robust to corruption and outliers in the training data, which are inevitable in real-world deployment, and (ii) can leverage expert feedback through high-quality labeled data. We introduce an autoencoder formulation that (i) gives robustness through a non-convex loss and a heavy-tailed distribution model on the residuals and (ii) enables semi-supervised learning with labeled outliers. Results on three large medical datasets show that our method outperforms the state of the art in abnormality-detection accuracy.
更多查看译文
关键词
Abnormality detection, deep neural networks, robust learning, quasi-norm loss, semi-supervision
AI 理解论文
溯源树
样例
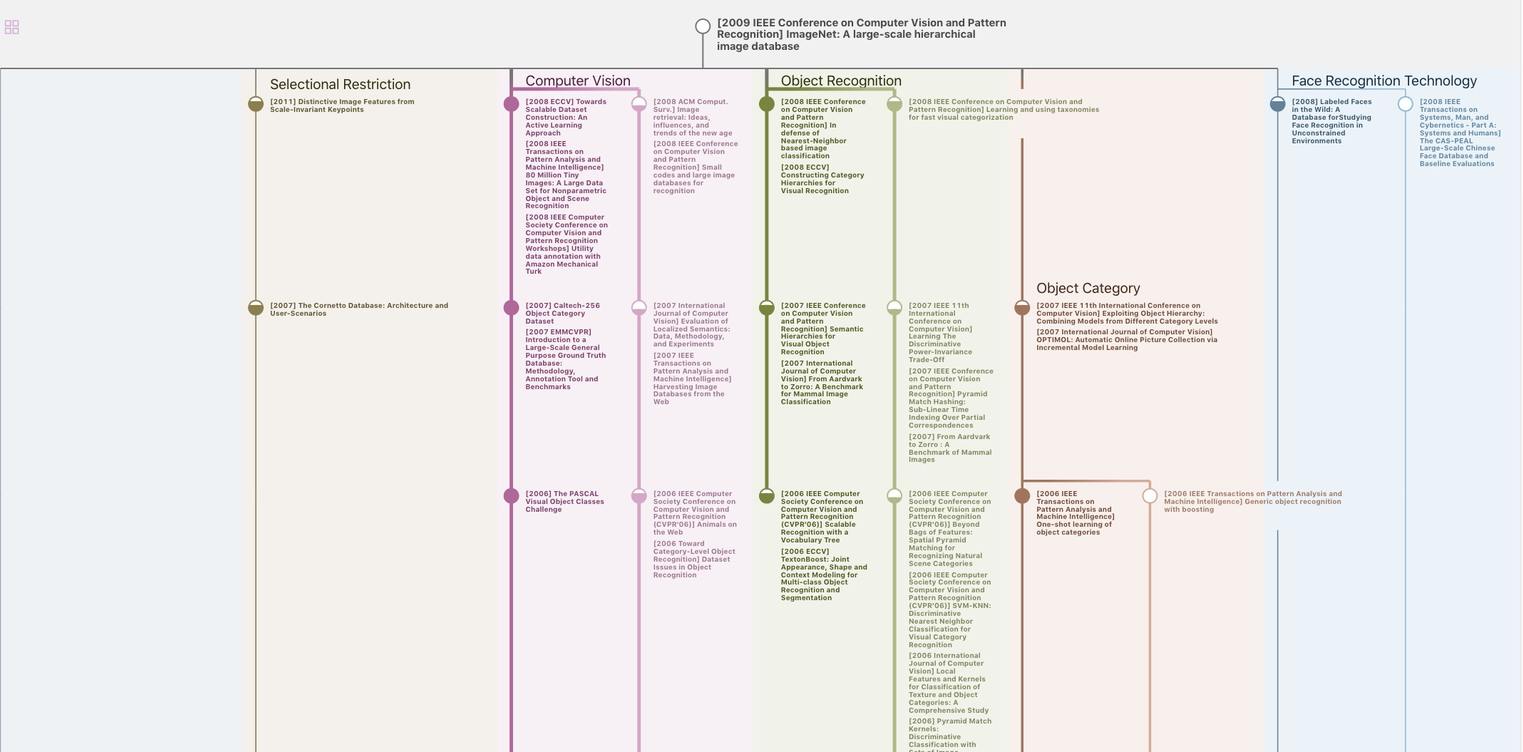
生成溯源树,研究论文发展脉络
Chat Paper
正在生成论文摘要