Radiomics for pretreatment prediction of pathological response to neoadjuvant therapy using magnetic resonance imaging: Influence of feature selection
2018 IEEE 15th International Symposium on Biomedical Imaging (ISBI 2018)(2018)
摘要
Texture features from breast MRI have shown promising results in the early prediction of pathological complete response (pCR) to neoadjuvant chemotherapy (NAC). However, feature selection (FS) is of key importance to discard attributes that can be source of noise, thus decreasing the classifier performance. In this study, we extracted 27 3D texture features from the dynamic contrast enhanced-MRI, and we created four feature subsets using different FS algorithms: a) F-Score measure, b) a genetic algorithm (GA) and c) two versions of an ant colony optimization (ACO) algorithm. All subsets were fed into a Bayesian classifier, and their performances were compared. Using GA and ACO, the area under the ROC curve (AUC) increased by 25% and 8% with respect to the subsets containing all texture features and the F-score subset, respectively. With GA, AUC was 0.84, sensitivity=82.7% and specificity=74%. FS can strongly improve the performance of texture features in predicting pCR.
更多查看译文
关键词
Breast MRI,NAC Response,features selection,genetic algorithms,ACO
AI 理解论文
溯源树
样例
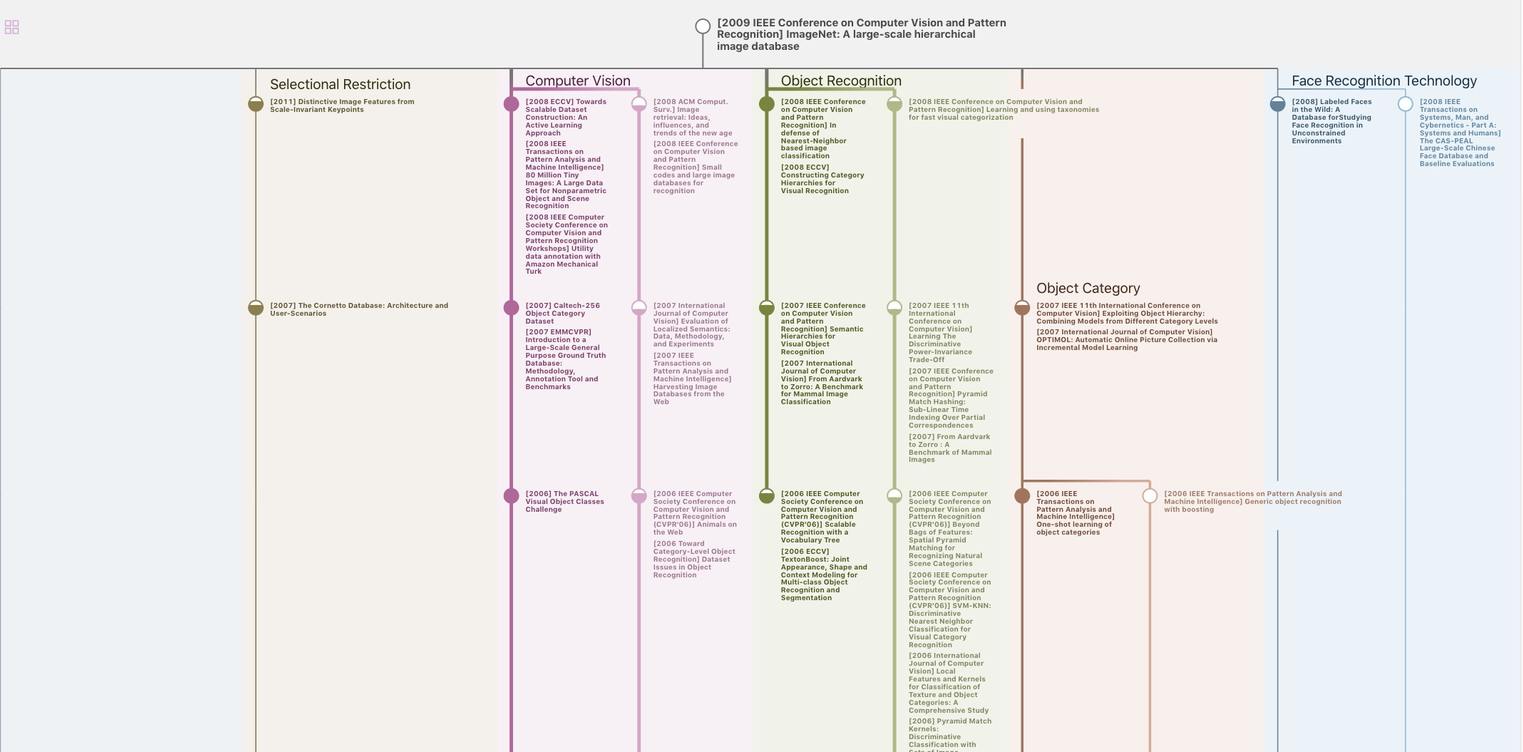
生成溯源树,研究论文发展脉络
Chat Paper
正在生成论文摘要