Using material classification methods for steel surface defect inspection
2016 IEEE 25th International Symposium on Industrial Electronics (ISIE)(2016)
摘要
Steel surface defect inspection is an important industrial application of machine vision that can be used to inspect the quality of many products. Most researchers in this field usually start from a task and design task-specific methods for such task. However, most surface defects can be viewed as surface areas that have abnormal material characteristics and material classification is an extensively studied field in computer vision. It will be very interesting to explore how the state-of-the-art method designed for general material classification works for domain-specific applications like defect inspection and how to improve it so that it can be adapted to real industrial applications where training samples are usually difficult to collect. In this paper, we will show our finding that the state-of-the-art general method for material classification can achieve better performance than the current best tailored method for steel surface defect inspection. In order to handle the difficulties in collecting training samples, we propose a new method called Local SIFT Pattern (LSP), based on the general method. The LSP can achieve satisfactory accuracy with extremely limited training samples.
更多查看译文
关键词
Steel surface defect classification,Local SIFT Pattern,Local Binary Pattern,Local Self Similarity,Improved Fisher Vector
AI 理解论文
溯源树
样例
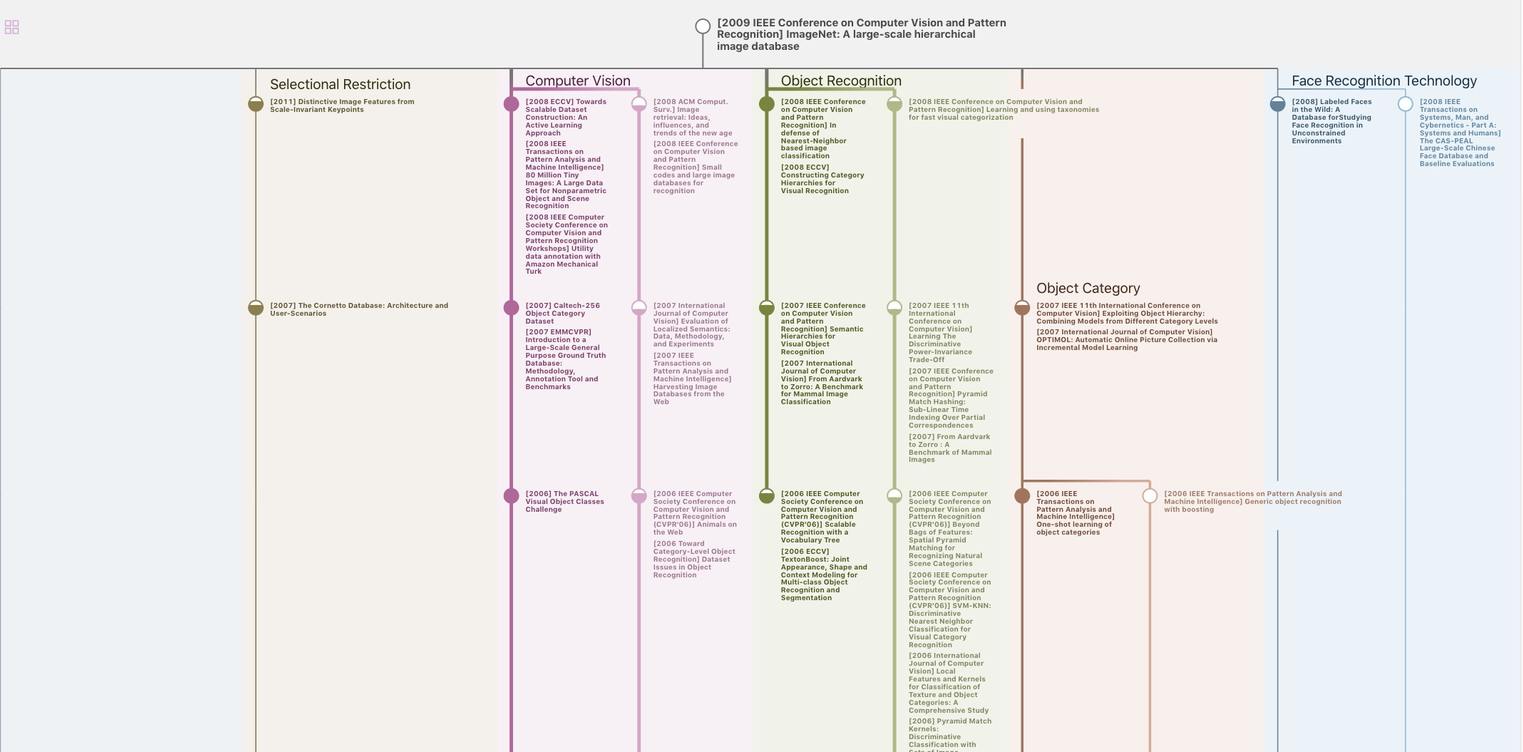
生成溯源树,研究论文发展脉络
Chat Paper
正在生成论文摘要