Group-Pair Convolutional Neural Networks for Multi-View Based 3D Object Retrieval.
THIRTY-SECOND AAAI CONFERENCE ON ARTIFICIAL INTELLIGENCE / THIRTIETH INNOVATIVE APPLICATIONS OF ARTIFICIAL INTELLIGENCE CONFERENCE / EIGHTH AAAI SYMPOSIUM ON EDUCATIONAL ADVANCES IN ARTIFICIAL INTELLIGENCE(2018)
摘要
In recent years, research interest in object retrieval has shifted from 2D towards 3D data. Despite many well-designed approaches, we point out that limitations still exist and there is tremendous room for improvement, including the heavy reliance on hand-crafted features, the separated optimization of feature extraction and object retrieval, and the lack of sufficient training samples. In this work, we address the above limitations for 3D object retrieval by developing a novel end-to-end solution named Group Pair Convolutional Neural Network (GPCNN). It can jointly learn the visual features from multiple views of a 3D model and optimize towards the object retrieval task. To tackle the insufficient training data issue, we innovatively employ a pair-wise learning scheme, which learns model parameters from the similarity of each sample pair, rather than the traditional way of learning from sparse label-sample matching. Extensive experiments on three public benchmarks show that our GPCNN solution significantly outperforms the state-of-the-art methods with 3% to 42% improvement in retrieval accuracy.
更多查看译文
AI 理解论文
溯源树
样例
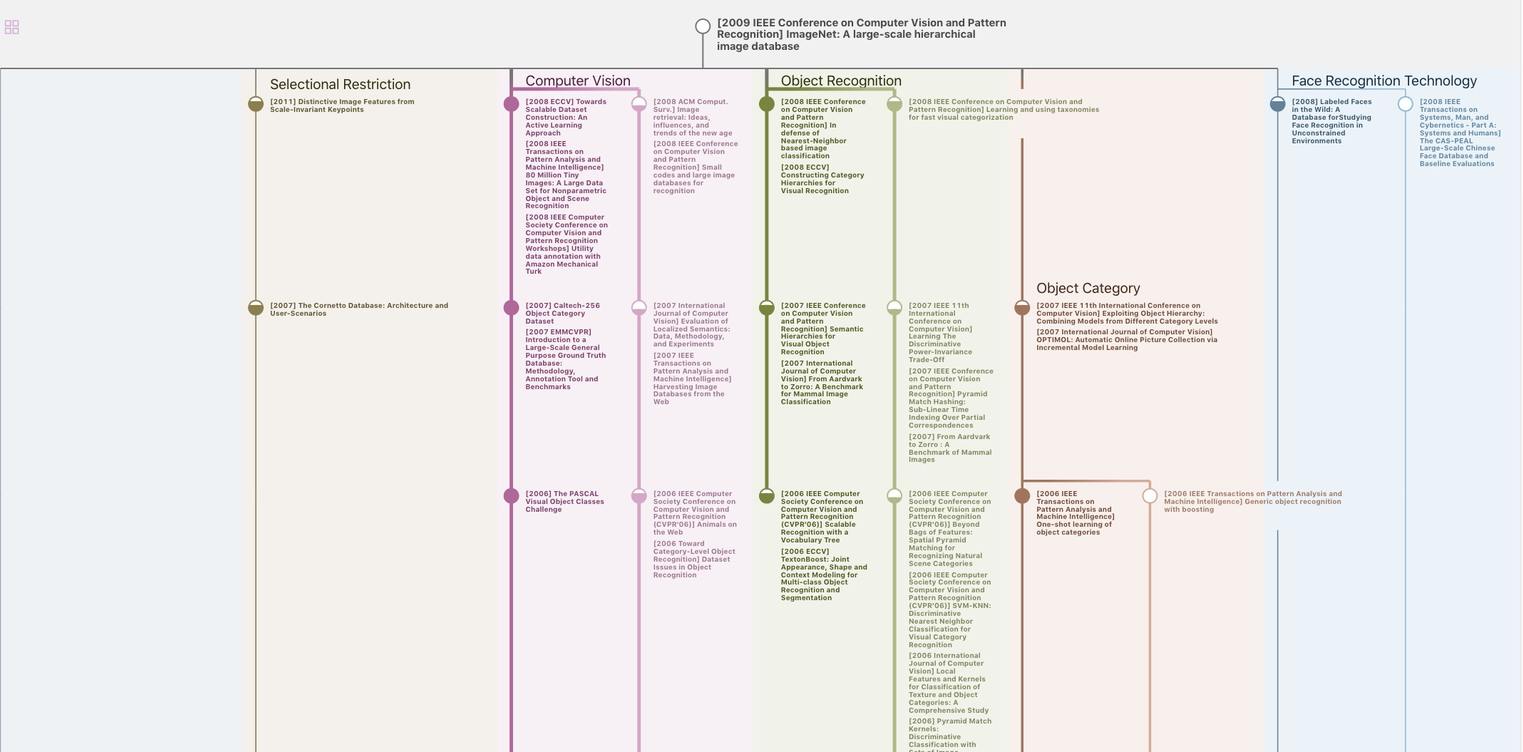
生成溯源树,研究论文发展脉络
Chat Paper
正在生成论文摘要