Exact MAP-Inference by Confining Combinatorial Search With LP Relaxation.
THIRTY-SECOND AAAI CONFERENCE ON ARTIFICIAL INTELLIGENCE / THIRTIETH INNOVATIVE APPLICATIONS OF ARTIFICIAL INTELLIGENCE CONFERENCE / EIGHTH AAAI SYMPOSIUM ON EDUCATIONAL ADVANCES IN ARTIFICIAL INTELLIGENCE(2018)
摘要
We consider the MAP-inference problem for graphical models, which is a valued constraint satisfaction problem defined on real numbers with a natural summation operation. We propose a family of relaxations (different from the famous Sherali-Adams hierarchy), which naturally define lower bounds for its optimum. This family always contains a tight relaxation and we give an algorithm able to find it and therefore, solve the initial non-relaxed NP-hard problem. The relaxations we consider decompose the original problem into two non-overlapping parts: an easy LP-tight part and a difficult one. For the latter part a combinatorial solver must be used. As we show in our experiments, in a number of applications the second, difficult part constitutes only a small fraction of the whole problem. This property allows to significantly reduce the computational time of the combinatorial solver and therefore solve problems which were out of reach before.
更多查看译文
关键词
lp relaxation,confining combinatorial search,map-inference
AI 理解论文
溯源树
样例
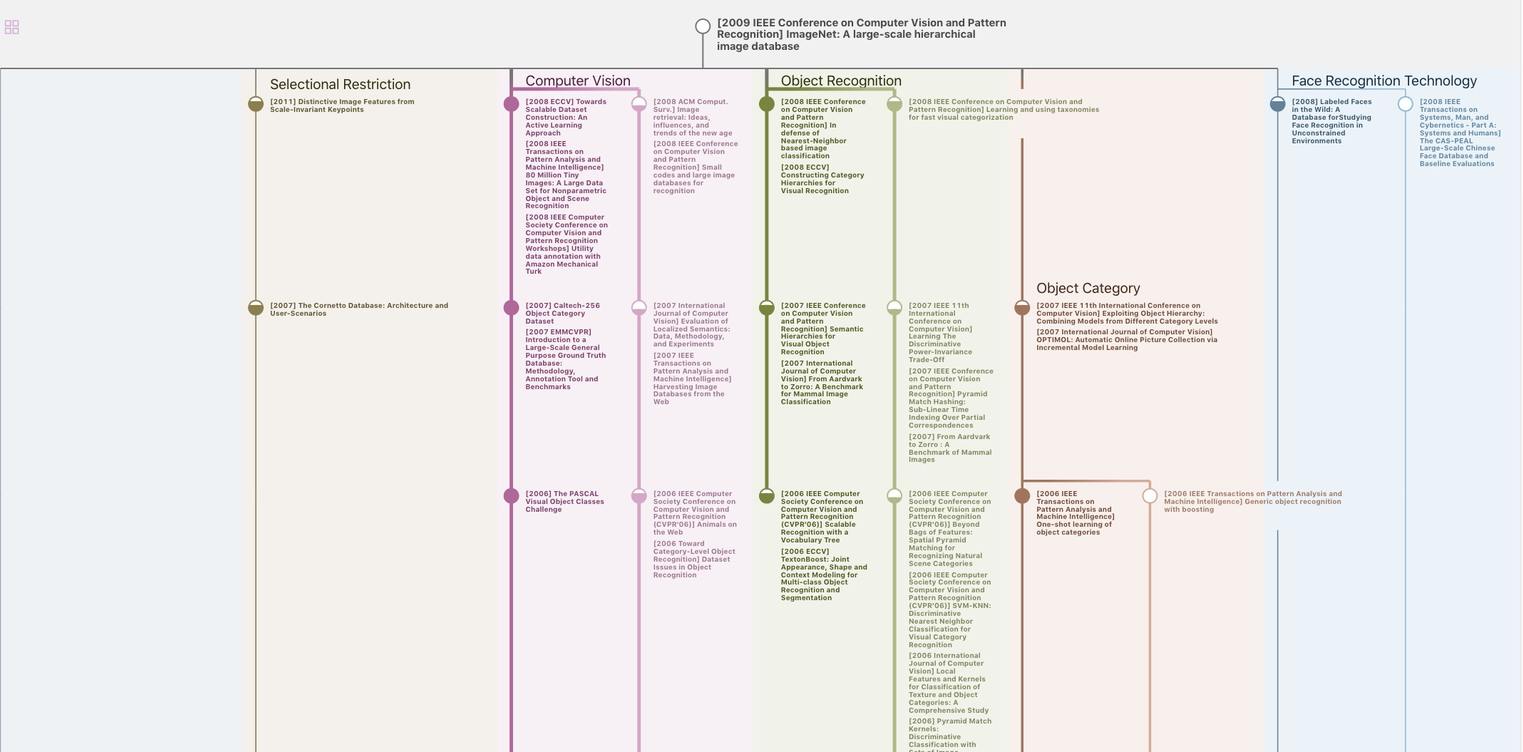
生成溯源树,研究论文发展脉络
Chat Paper
正在生成论文摘要