Selective Verification Strategy for Learning From Crowds.
THIRTY-SECOND AAAI CONFERENCE ON ARTIFICIAL INTELLIGENCE / THIRTIETH INNOVATIVE APPLICATIONS OF ARTIFICIAL INTELLIGENCE CONFERENCE / EIGHTH AAAI SYMPOSIUM ON EDUCATIONAL ADVANCES IN ARTIFICIAL INTELLIGENCE(2018)
摘要
To deal with the low qualities of web workers in crowdsourcing, many unsupervised label aggregation methods have been investigated but most of them provide inconsistent performance. In this paper, we explore the learning from crowds with selective verification problem. In addition to the noisy responses from the crowds, it also collects the ground truths for a well-chosen subset of tasks as the reference, then aggregates the redundant responses based on the patterns provided by both the supervised and unsupervised signal. To improve the labeling efficiency, we propose the EBM selecting strategy for choosing the verification subset, which is based on the loss error minimization. Specifically, we first establish the expected loss error given the semi-supervised learning estimate, then find the subset that minimizes this selecting criterion. We do extensive empirical comparisons on both synthetic and real-world datasets to show the benefits of this new learning setting as well as our proposal.
更多查看译文
AI 理解论文
溯源树
样例
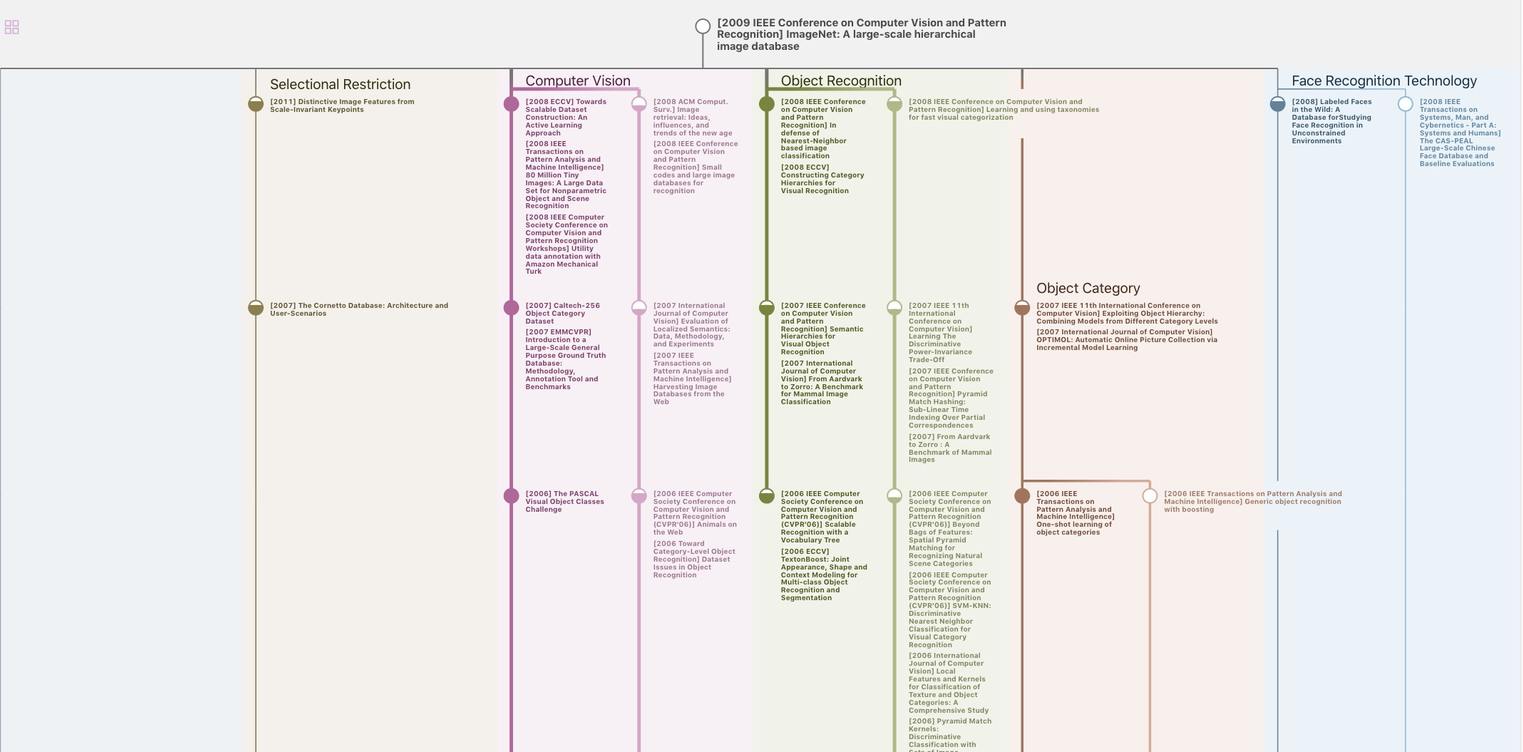
生成溯源树,研究论文发展脉络
Chat Paper
正在生成论文摘要