Learning From Semi-Supervised Weak-Label Data.
THIRTY-SECOND AAAI CONFERENCE ON ARTIFICIAL INTELLIGENCE / THIRTIETH INNOVATIVE APPLICATIONS OF ARTIFICIAL INTELLIGENCE CONFERENCE / EIGHTH AAAI SYMPOSIUM ON EDUCATIONAL ADVANCES IN ARTIFICIAL INTELLIGENCE(2018)
摘要
Multi-label learning deals with data objects associated with multiple labels simultaneously. Previous studies typically assume that for each instance, the full set of relevant labels associated with each training instance is given. In many applications such as image annotation, however, it's usually difficult to get the full label set for each instance and only a partial or even empty set of relevant labels is available. We call this kind of problem as 'semi-supervised weak-label learning' problem. In this work we propose the SSWL (Semi-Supervised Weak-Label) method to address this problem. Both instance similarity and label similarity are considered for the complement of missing labels. Ensemble of multiple models are utilized to improve the robustness when label information is insufficient. We formulate the objective as a bi-convex optimization problem with an efficient block coordinate descent algorithm. Experiments validate the effectiveness of SSWL.
更多查看译文
AI 理解论文
溯源树
样例
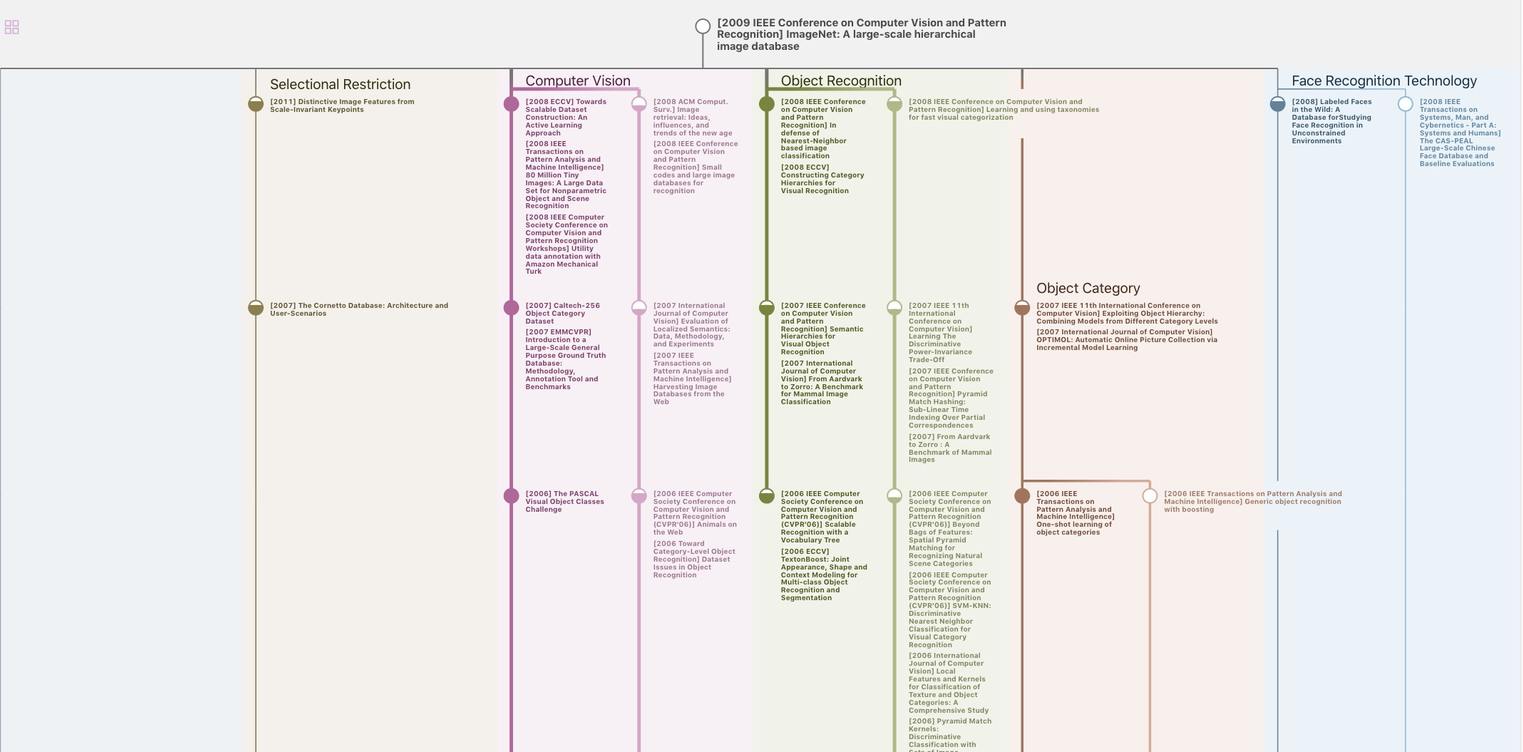
生成溯源树,研究论文发展脉络
Chat Paper
正在生成论文摘要