Matrix Profile X: VALMOD - Scalable Discovery of Variable-Length Motifs in Data Series.
SIGMOD/PODS '18: International Conference on Management of Data Houston TX USA June, 2018(2018)
摘要
In the last fifteen years, data series motif discovery has emerged as one of the most useful primitives for data series mining, with applications to many domains, including robotics, entomology, seismology, medicine, and climatology. Nevertheless, the state-of-the-art motif discovery tools still require the user to provide the motif length. Yet, in at least some cases, the choice of motif length is critical and unforgiving. Unfortunately, the obvious brute-force solution, which tests all lengths within a given range, is computationally untenable. In this work, we introduce VALMOD, an exact and scalable motif discovery algorithm that efficiently finds all motifs in a given range of lengths. We evaluate our approach with five diverse real datasets, and demonstrate that it is up to 20 times faster than the state-of-the-art. Our results also show that removing the unrealistic assumption that the user knows the correct length, can often produce more intuitive and actionable results, which could have been missed otherwise.
更多查看译文
关键词
Data Series,Time Series,Motif Discovery,Variable Length,Data Mining
AI 理解论文
溯源树
样例
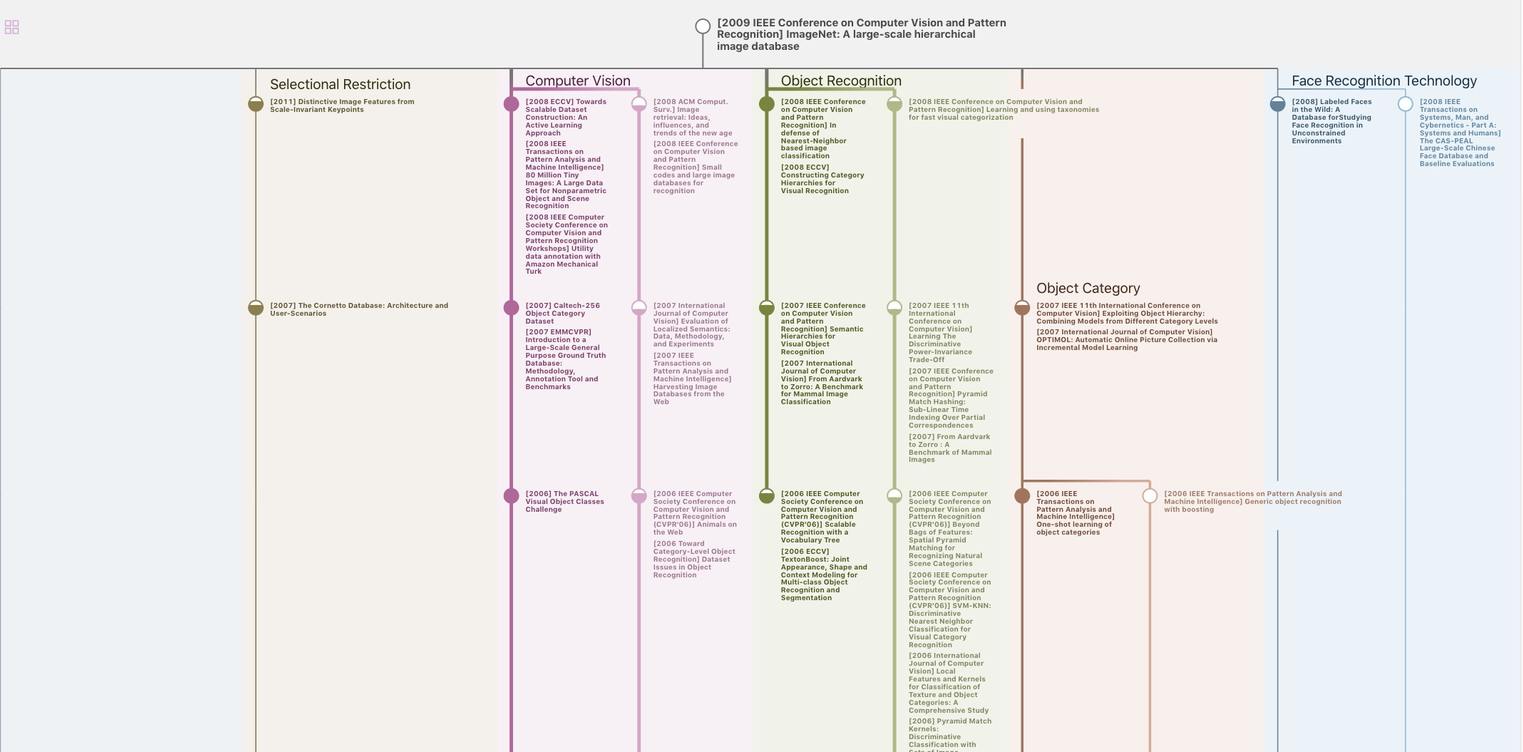
生成溯源树,研究论文发展脉络
Chat Paper
正在生成论文摘要