Large-Scale 3d Point Cloud Classification Based On Feature Description Matrix By Cnn
CASA(2018)
摘要
Large-scale 3D Point cloud classification is a basic topic for various applications. Traditional geometries features are usually independent of each other and difficult to adapt to a fixed classification model. With the rise of the neural network, deep learning is considered in 3D point cloud application. 3D points are difficult to feed the neural network directly based on deep learning, as they cannot be arranged in a fixed order as image pixels. In this paper, we combine traditional feature-based methods with the Convolutional neural network(CNN) to finish the classification task. The core idea is to construct a feasible structure called Feature Description Matrix(FDM) which encapsulates the local feature of the point to feed CNN for training and testing. By extracting geometry features and designed Feature Description Vectors(FDV) for FDM, a simple mechanism for point cloud classification is given, and experiments validate the effectiveness of our method, with higher classification accuracy compared to state-of-art works.
更多查看译文
关键词
point cloud, feature extraction, deep learning, feature description matrix
AI 理解论文
溯源树
样例
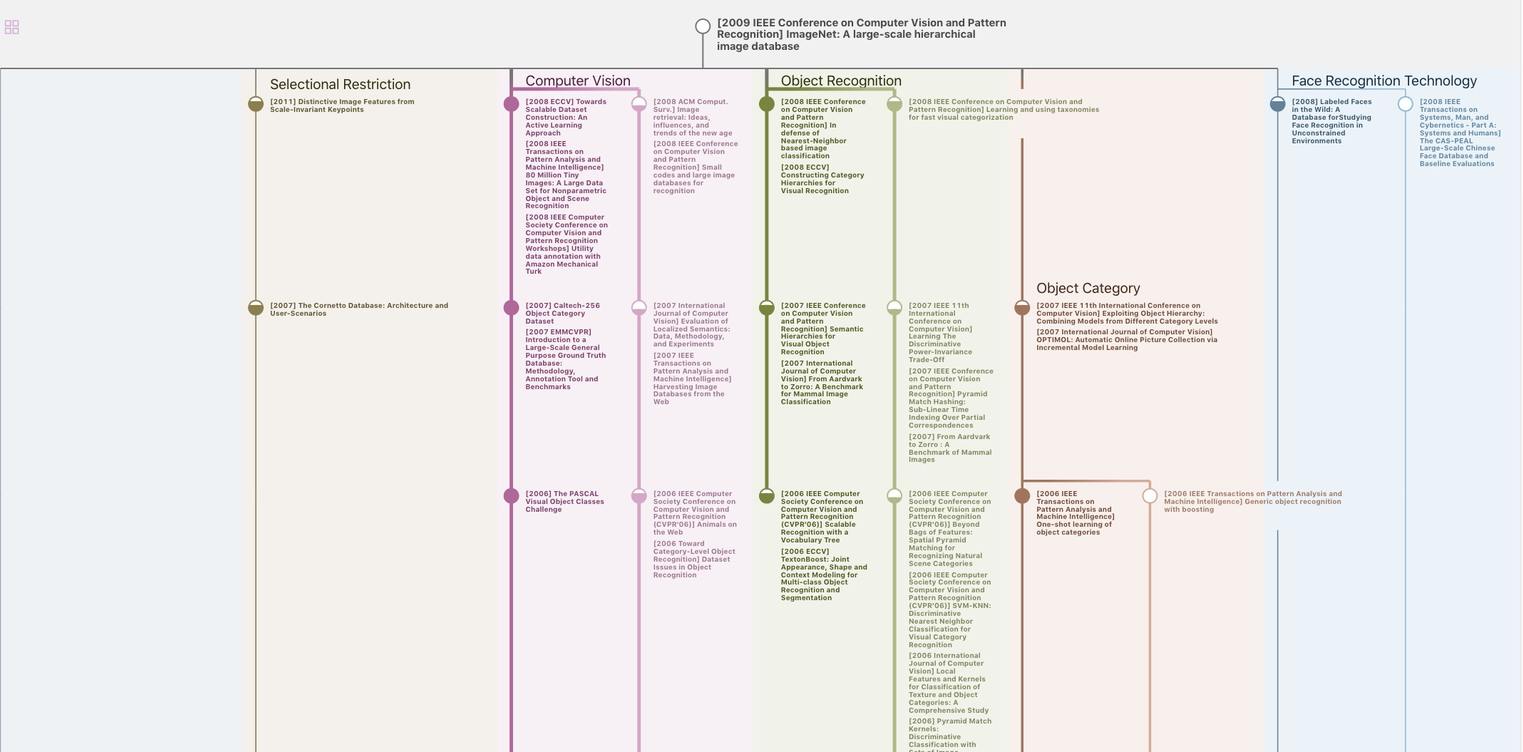
生成溯源树,研究论文发展脉络
Chat Paper
正在生成论文摘要