Enhancing Traffic Model Of Big Cities: Network Skeleton & Reciprocity
2018 10TH INTERNATIONAL CONFERENCE ON COMMUNICATION SYSTEMS & NETWORKS (COMSNETS)(2018)
摘要
Handling major challenges like traffic volume estimation, mobility pattern detection and feature extraction in mobility network usually form a weak balance among them. Most of the works are focused towards one of these areas which fail in improving altogether. In this paper, we present a model with modified conventional methods meeting all three above challenges to an extent. Extracting new temporal & directional feature, we introduce Reciprocity metric. It proves to be more informative and efficient in capturing mobility pattern of the network than existing metrics. We introduce the idea of network skeleton which is a reduced form of mobility network but captures approx 90% of its inherent characteristics. Network Skeleton can extract higher level of information from the network while enhancing network's short-term predictability. Our work has the following steps: 1) extracting and building "link reciprocity", a more informative feature; 2) pattern detection in random mobility introduced by "convergence of mobility network"; and 3) estimation of network skeleton formed using a link based approach for short-term forecasting. Our network convergence method outperforms conventional approaches and detects active regions at a very fast rate compared to other approaches. Long ShortTerm Memory (LSTM), a kind of Recursive Neural Networks (RNN) capable of learning long-term dependencies is used to estimate network traffic. Indicating link based network-skeleton helps to reduce short-term forecasting error up to 6% and 3/4 times in different time-slots. Our network skeleton approach can be used to meet the general problems of the traffic-rules formulation by characterizing important routes (links), detecting regions of high importance in less time and predicting short-term traffic volume in a more accurate way. Moreover, network skeleton with reduced network-size can be easily operable with existing methodologies, which is another essential contribution of our work.
更多查看译文
关键词
reciprocity, convergence, network skeleton
AI 理解论文
溯源树
样例
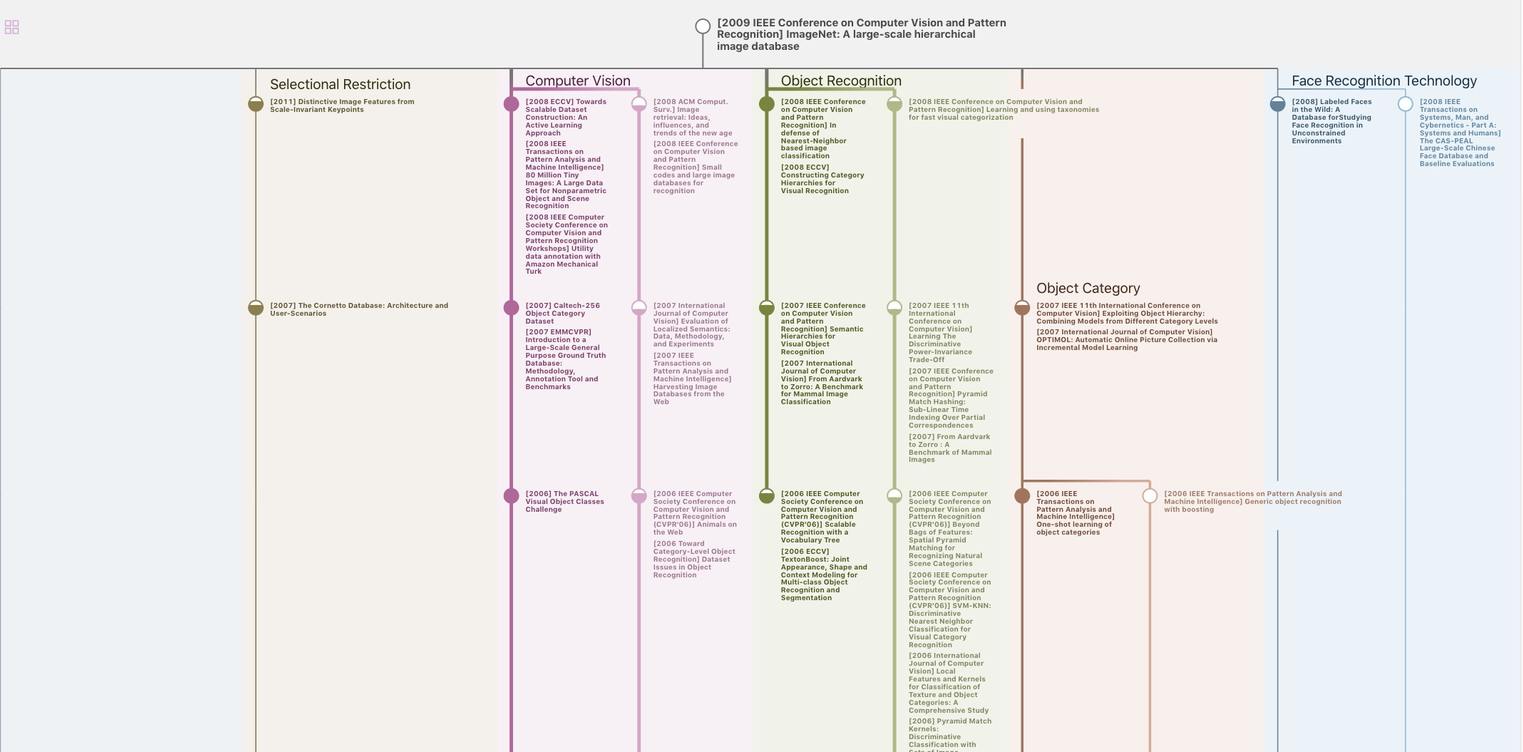
生成溯源树,研究论文发展脉络
Chat Paper
正在生成论文摘要