Toward automatic segmentation and quantification of tumor and stroma in whole-slide images of H and E stained rectal carcinomas.
Proceedings of SPIE(2015)
摘要
Visual estimation of tumor and stroma proportions in microscopy images yields a strong, Tumor-(lymph) Node-Metastasis (TNM) classification-independent predictor for patient survival in colorectal cancer. Therefore, it is also a potent (contra) indicator for adjuvant chemotherapy. However, quantification of tumor and stroma through visual estimation is highly subject to intra-and interobserver variability. The aim of this study is to develop and clinically validate a method for objective quantification of tumor and stroma in standard hematoxylin & eosin (H&E) stained microscopy slides of rectal carcinomas. A tissue segmentation algorithm, based on supervised machine learning and pixel classification, was developed, trained and validated using histological slides that were prepared from surgically excised rectal carcinomas in patients who had not received neoadjuvant chemotherapy and/or radiotherapy. Whole-slide scanning was performed at 20x magnification. A total of 40 images (4 million pixels each) were extracted from 20 whole-slide images at sites showing various relative proportions of tumor and stroma. Experienced pathologists provided detailed annotations for every extracted image. The performance of the algorithm was evaluated using cross-validation by testing on 1 image at a time while using the other 39 images for training. The total classification error of the algorithm was 9.4% (SD = 3.2%). Compared to visual estimation by pathologists, the algorithm was 7.3 times (P = 0.033) more accurate in quantifying tissues, also showing 60% less variability. Automatic tissue quantification was shown to be both reliable and practicable. We ultimately intend to facilitate refined prognostic stratification of (colo) rectal cancer patients and enable better personalized treatment.
更多查看译文
关键词
Segmentation,Quantification,Stroma,Hematoxylin and Eosin,Rectal cancer,Colorectal cancer,Pixel classification,Supervised machine learning
AI 理解论文
溯源树
样例
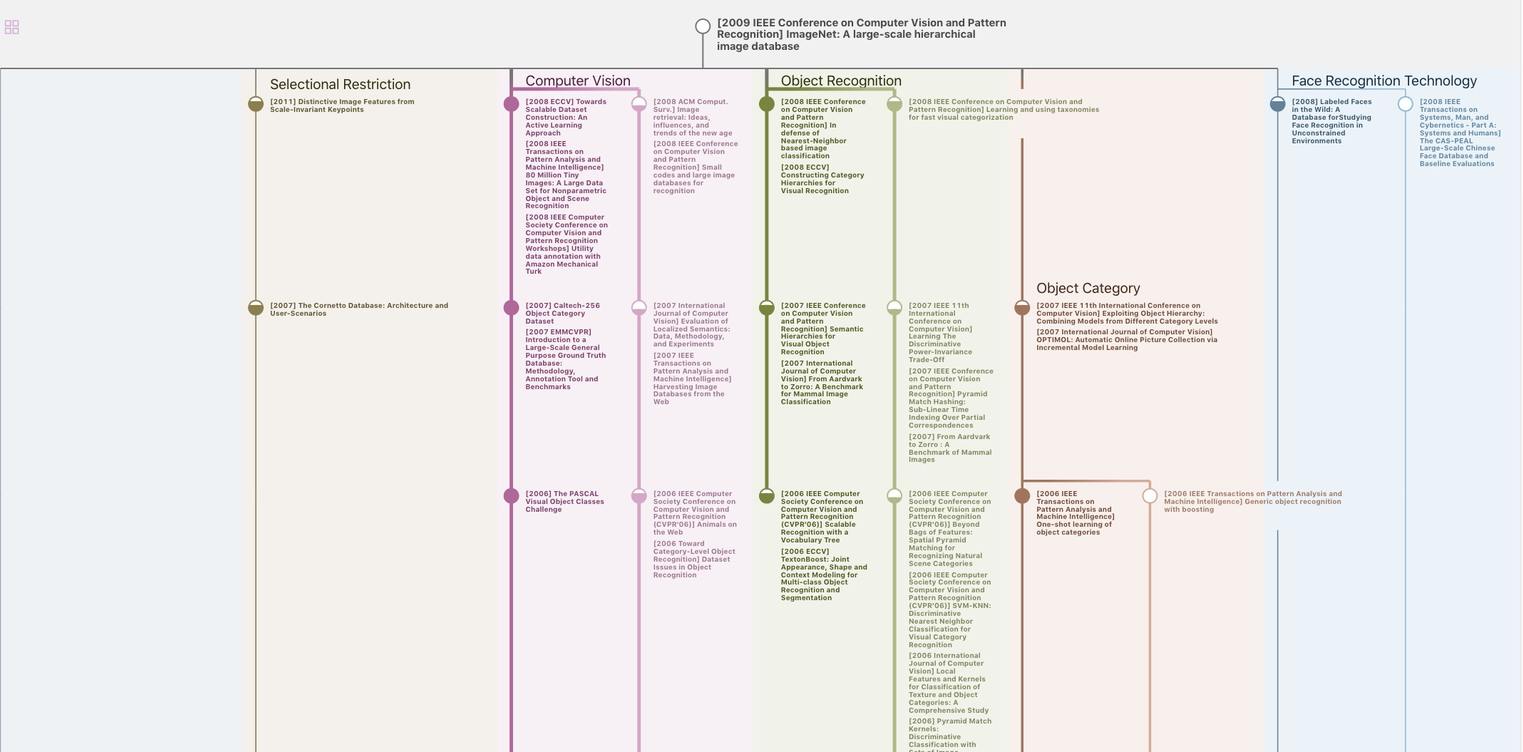
生成溯源树,研究论文发展脉络
Chat Paper
正在生成论文摘要