A Computer Vision Approach for Lung Cancer Classification Using FNAC-Based Cytological Images.
CVIP(2018)
摘要
Lung cancer represents malignant tumour having uncontrolled lung cell growth/proliferation. It can be diagnosed by invasive and non-invasive diagnostic approaches. One of the most effective and accurate approach is Papanicolaou (Pap)-stained cell cytology from fine needle aspiration cytology (FNAC). The manual assessment of cytopathology slides under light microscopy is time-consuming and suffers from feature ambiguities including inter-observer variability. To overcome such problems, the automated cytological analysis is the need of time. This study presents an automated computer vision approach to identify and classify cancerous cell present in microscopic images of Pap smear. The proposed methodology follows colour normalization, image filtering, nucleus segmentation and classification of segmented cells. The nucleus is segmented using the Random Walker with K-means clustering method. The post-processing is carried out on the segmented images to delineate joined nucleus and to remove unwanted regions. Subsequently, multiple nuclear features, i.e. colour, texture and geometric attributes are extracted from each segmented nucleus. After that, a comparative study on supervised classifier selection for the extracted features was adopted towards improving classification accuracy for distinguishing nucleus of non-small cell and small cell lung cancer. Artificial neural network performs best with sensitivity of \( 97.58\% \), specificity of \( 97.6\% \), accuracy of \( 97.46\% \).
更多查看译文
关键词
Lung Cancer Classification, Nuclei Segmentation, Nuclei Classification, Nucleus Region, Contrast Limited Adaptive Histogram Equalization (CLAHE)
AI 理解论文
溯源树
样例
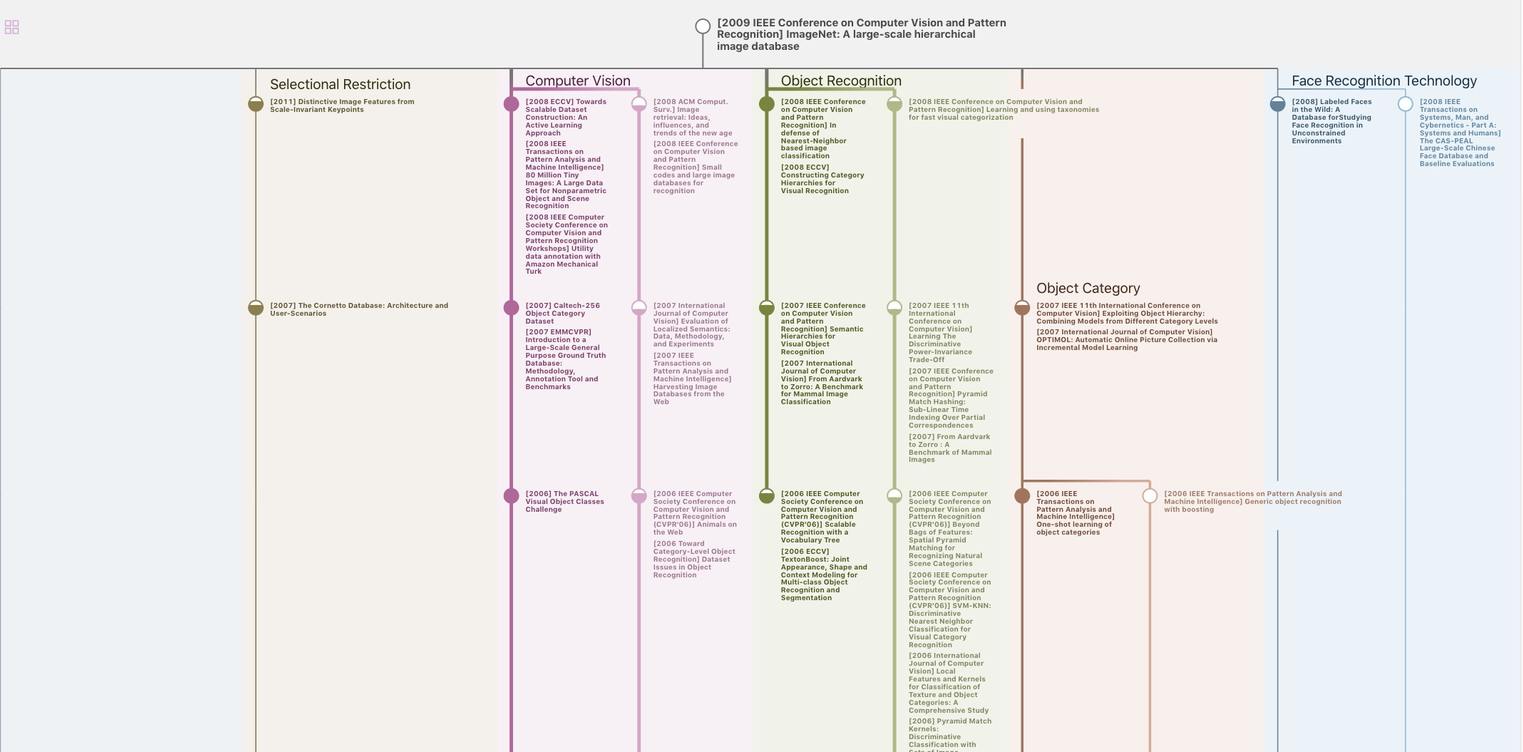
生成溯源树,研究论文发展脉络
Chat Paper
正在生成论文摘要