Improving domain-specific SMT for low-resourced languages using data from different domains.
LREC(2018)
摘要
This paper evaluates the impact of different types of data sources in developing a domain-specific statistical machine translation (SMT) system for the domain of official government letters, between the low-resourced language pair Sinhala and Tamil. The baseline was built with a small in-domain parallel dataset containing official government letters. The translation system was evaluated with two different test data sets. Test data from the same sources as training and tuning gave a higher score due to over-fitting, while the test data from a different source resulted in a considerably lower score. With the motive to improve translation, more data was collected from, (a) different government sources other than official letters (pseudo in-domain), and (b) online sources such as blogs, news and wiki dumps (out-domain). Use of pseudo in-domain data showed an improvement for both the test sets as the language is formal and context was similar to that of the in-domain though the writing style varies. Out-domain data, however, did not give a positive impact, either in filtered or unfiltered forms, as the writing style was different and the context was much more general than that of the official government documents.
更多查看译文
关键词
domain-specific statistical machine translation, low-resourced languages, Sinhala, Tamil, Domain Adaptation
AI 理解论文
溯源树
样例
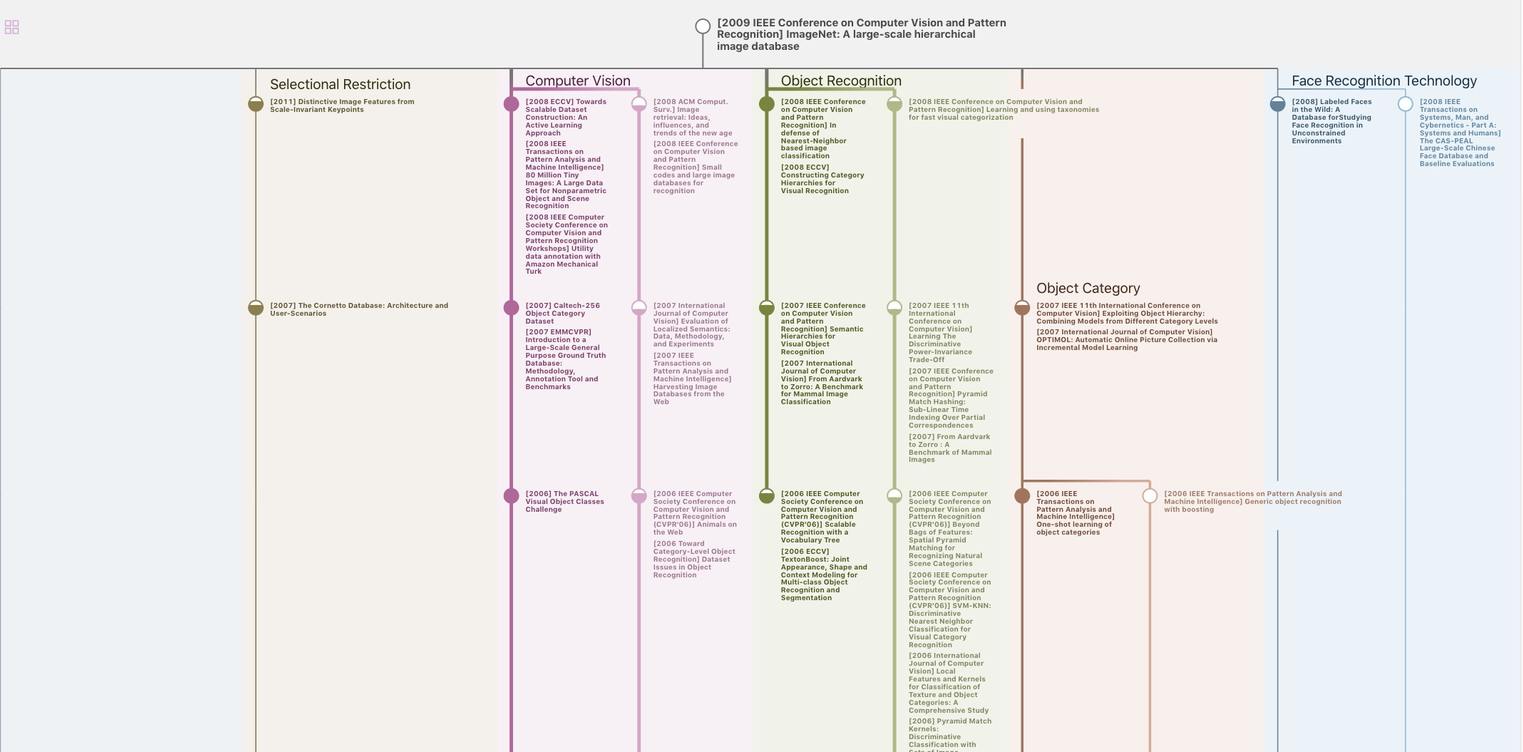
生成溯源树,研究论文发展脉络
Chat Paper
正在生成论文摘要