Non-Coding Rna Sequences Identification And Classification Using A Multi-Class And Multi-Label Ensemble Technique
ARTIFICIAL INTELLIGENCE APPLICATIONS AND INNOVATIONS, AIAI 2018(2018)
摘要
High throughput sequencing RNA-sequencing technologies and modern in silico techniques have expanded our knowledge on short non-coding RNAs. These sequences were initially split into various categories based on their cellular functionality and their sequential, thermodynamic and structural properties believing that their sequence can be used as an identifier to distinguish them. However, recent evidence has indicated that the same sequences can act and function as more than one type of non-coding RNAs with a striking example of mature microRNA sequences which can also be transfer RNA fragments. Most of the existing computational methods for the prediction of non-coding RNA sequences have emphasized on the prediction of only one type of noncoding RNAs and even the ones designed for multiclassification do not support multiple labeling and are thus not able to assign a sequence to more than one non-coding RNA type. In the present paper, we introduce a new multilabel- multiclass method based on the combination of multiobjective evolutionary algorithms and multilabel implementations of Random Forests to optimize the feature selection process and assign short RNA sequences to one or more non-coding RNA types. The overall methodology clearly outperformed other machine learning techniques which were used for the same purpose and it is applicable to data coming from RNA-sequencing experiments.
更多查看译文
关键词
Multi-label classification, Non-coding RNAs, Multi-objective optimization, Random forests, Dimensionality reduction
AI 理解论文
溯源树
样例
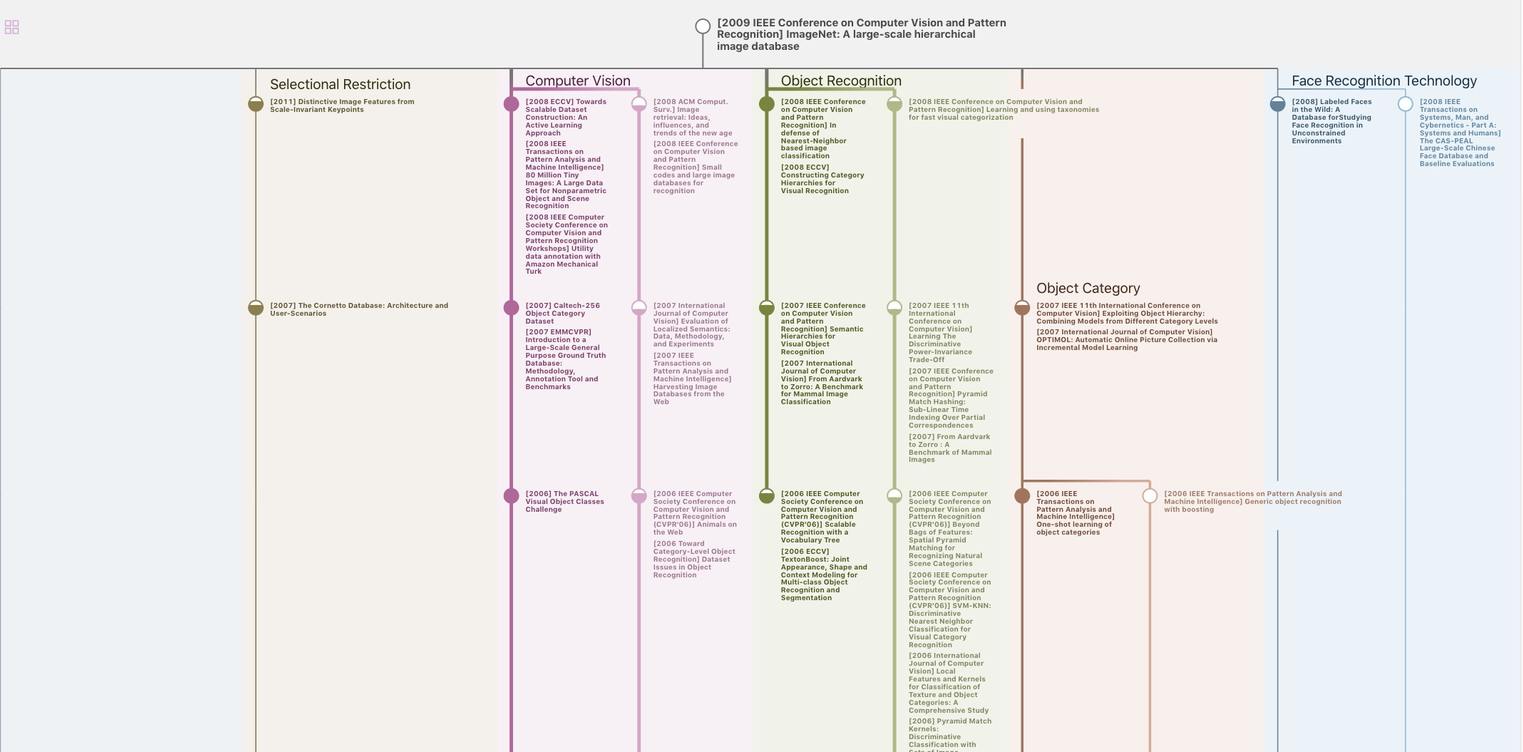
生成溯源树,研究论文发展脉络
Chat Paper
正在生成论文摘要