Image Copy-Move Forgery Detection Via An End-To-End Deep Neural Network
2018 IEEE WINTER CONFERENCE ON APPLICATIONS OF COMPUTER VISION (WACV 2018)(2018)
摘要
In this paper, for the first time, we introduce a new end-to-end deep neural network predicting forgery masks to the image copy-move forgery detection problem. Specifically, we use a convolutional neural network to extract block-like features from an image, compute self-correlations between different blocks, use a pointwise feature extractor to locate matching points, and reconstruct a forgery mask through a deconvolutional network. Unlike classic solutions requiring multiple stages of training and parameter tuning, ranging from feature extraction to postprocessing, the proposed solution is fully trainable and can be jointly optimized for the forgery mask reconstruction loss. Our experimental results demonstrate that the proposed method achieves better forgery detection performance than classic approaches relying on different features and matching schemes, and it is more robust against various known attacks like affine transformation, JPEG compression, blurring, etc.
更多查看译文
关键词
forgery mask reconstruction loss,end-to-end deep neural network,convolutional neural network,block-like features,pointwise feature extractor,deconvolutional network,Image Copy-Move Forgery Detection
AI 理解论文
溯源树
样例
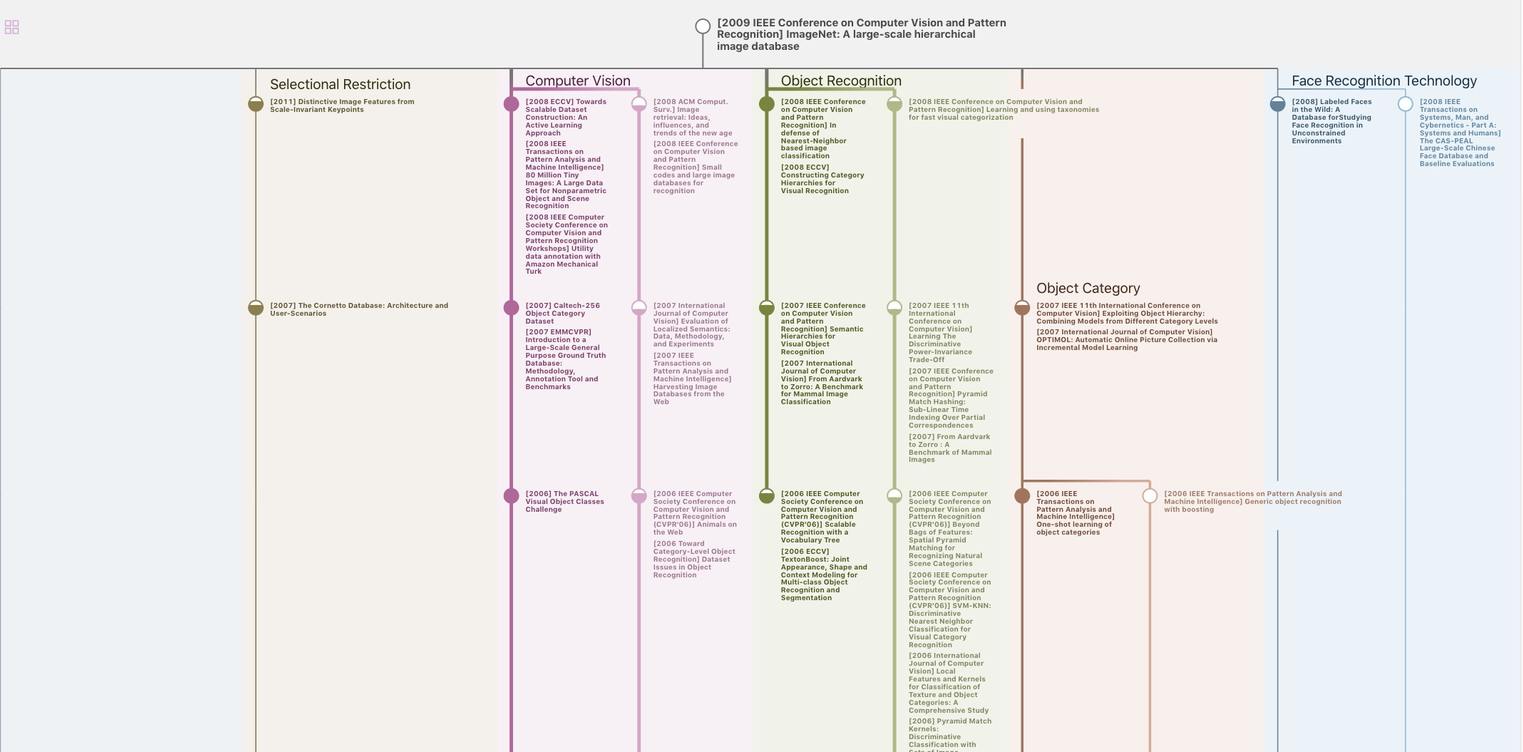
生成溯源树,研究论文发展脉络
Chat Paper
正在生成论文摘要