Discriminative Keyword Spotting for limited-data applications.
Speech Communication(2018)
摘要
Mobile devices are widely used around the world, frequently by people speaking local languages or dialects that are not well documented. For these languages, it might not be beneficial for commercial companies to develop Automatic Speech Recognition (ASR) systems, so users of these languages cannot utilize voice activation features (often using Keyword Spotting, KWS) of their devices. Standard KWS methods aim to statistically model the generation process of the speech signal, requiring hours of recorded and transcribed speech for training, and therefore are not adequate for limited-data scenarios. In this paper we propose a new KWS method, suitable for limited-data scenarios, which can be easily applied by developers. The proposed method uses a new histogram representation for words, obtained with respect to a pre-trained Gaussian Mixture Model (GMM). Sentences are represented by fixed-length global feature vectors, extracted from the response curves obtained by a word classifier. Word and sentence classifiers are trained using a discriminative approach, which is typically robust to training-set size. The dataset for training the GMM is easy to obtain, since no annotation is required. We compared the proposed system to a Hidden Markov Model (HMM) based system, trained using the same low data-resources conditions as ours, and to a state-of-the-art ASR system, trained using either the limited data scenario, or using many hours of recorded speech. In the limited data situation, our system performs better then both benchmarks in all experiments except for clean speech of children (CSLU dataset), where it performs as good as the HMM. Since the ASR benchmark performs poorly without enough training data, we also trained it without limiting the available data. In this case the ASR benchmark performs better when tested on speech of adults (TED-LIUM dataset of TED lectures) for all noise conditions, and our system performs better when tested on speech of children with low to moderate SNR values. The results demonstrate the advantages of the proposed system, and the conditions under which it performs better.
更多查看译文
关键词
AUC,Bagging predictors,Discriminative classification,Histogram representation,Keyword Spotting,ROC,SVM
AI 理解论文
溯源树
样例
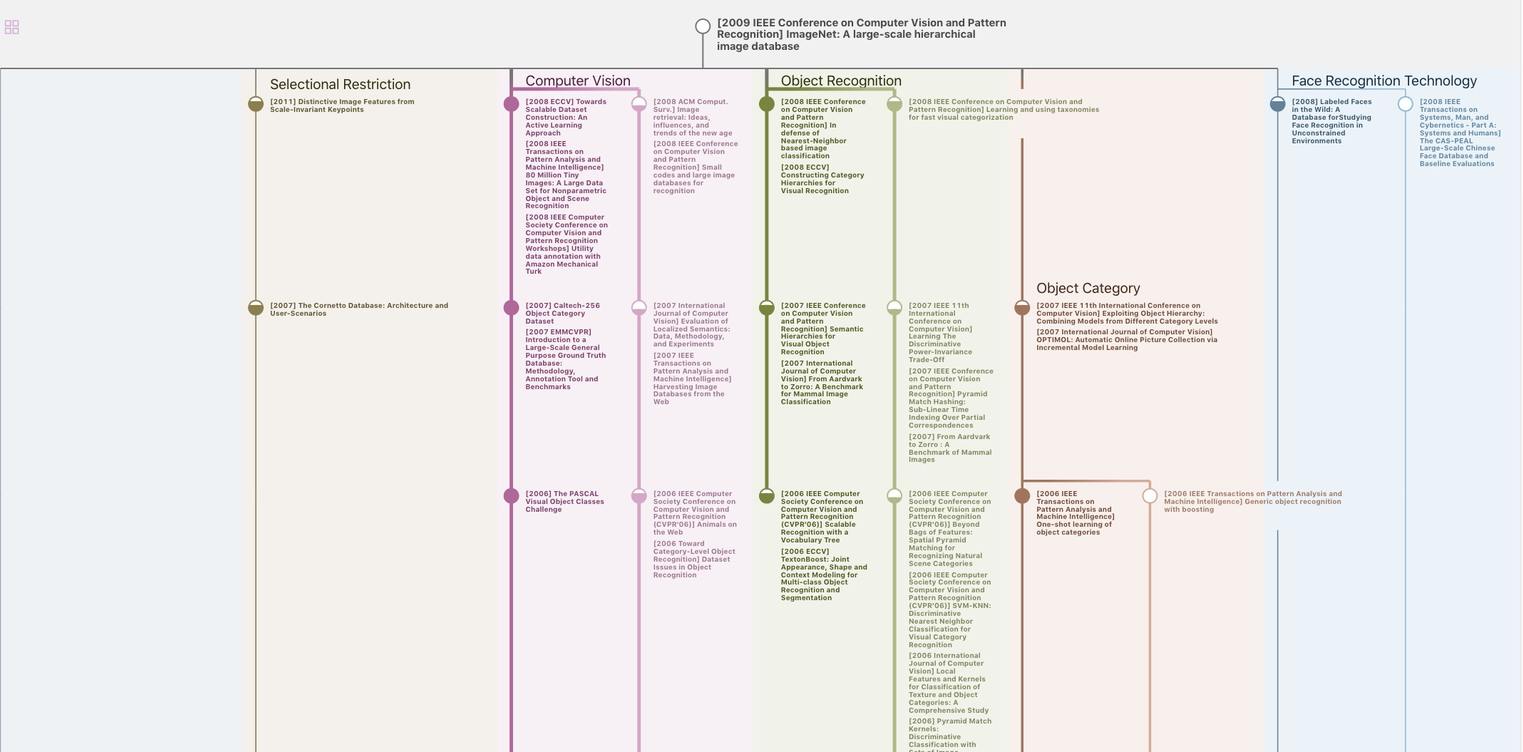
生成溯源树,研究论文发展脉络
Chat Paper
正在生成论文摘要