Glycaemic variability-based classification of impaired glucose tolerance vs. type 2 diabetes using continuous glucose monitoring data
Computers in Biology and Medicine(2018)
摘要
Many glycaemic variability (GV) indices extracted from continuous glucose monitoring systems data have been proposed for the characterisation of various aspects of glucose concentration profile dynamics in both healthy and non-healthy individuals. However, the inter-index correlations have made it difficult to reach a consensus regarding the best applications or a subset of indices for clinical scenarios, such as distinguishing subjects according to diabetes progression stage. Recently, a logistic regression-based method was used to address the basic problem of differentiating between healthy subjects and those affected by impaired glucose tolerance (IGT) or type 2 diabetes (T2D) in a pool of 25 GV-based indices. Whereas healthy subjects were classified accurately, the distinction between patients with IGT and T2D remained critical. In the present work, by using a dataset of CGM time-series collected in 62 subjects, we developed a polynomial-kernel support vector machine-based approach and demonstrated the ability to distinguish between subjects affected by IGT and T2D based on a pool of 37 GV indices complemented by four basic parameters—age, sex, BMI, and waist circumference—with an accuracy of 87.1%.
更多查看译文
关键词
Classification,Continuous glucose monitoring,Diabetes,Glycaemic variability,Support vector machine
AI 理解论文
溯源树
样例
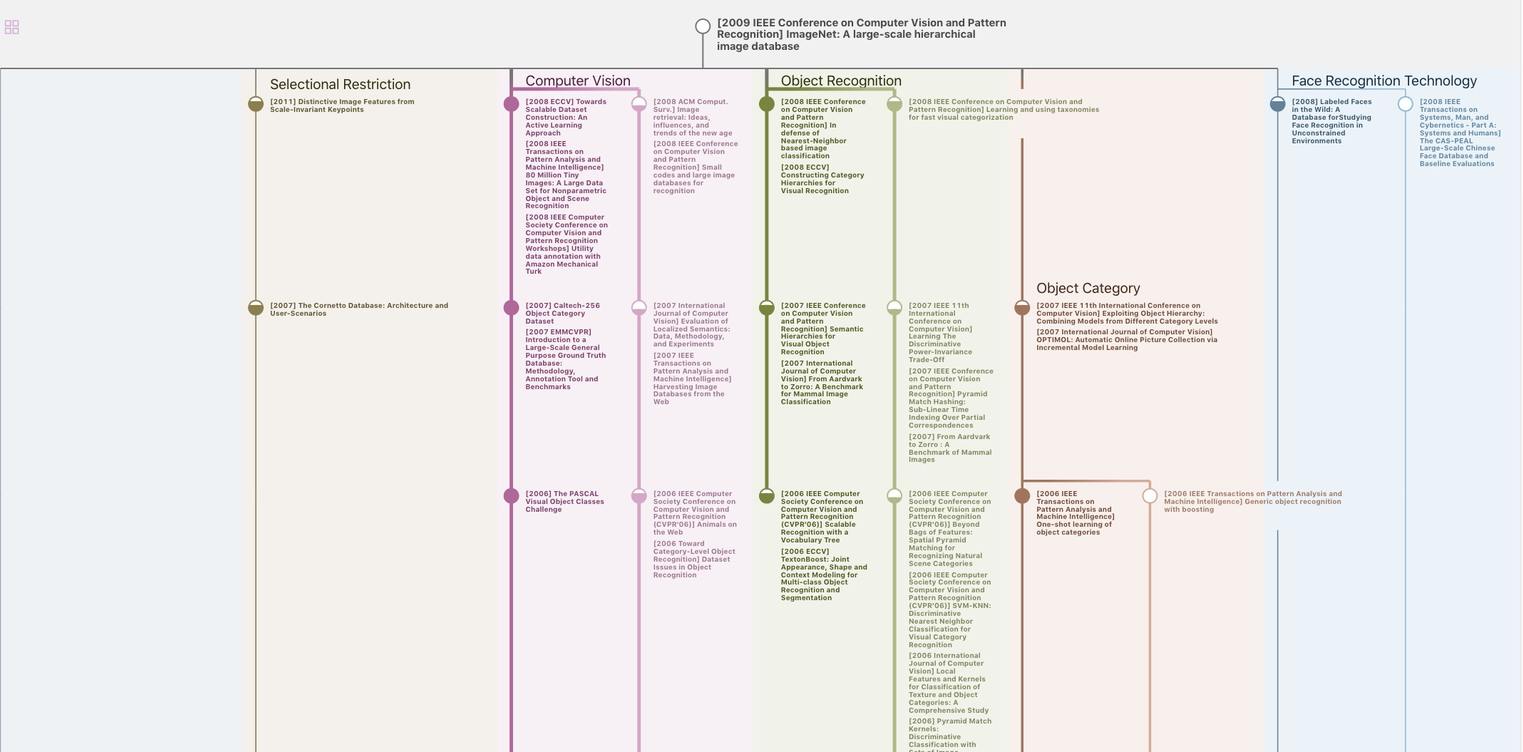
生成溯源树,研究论文发展脉络
Chat Paper
正在生成论文摘要