A multiple time series-based recurrent neural network for short-term load forecasting
Soft Comput.(2017)
摘要
Electricity, an indispensable resource in daily life and industrial production, is hard to store, so accurate short-term load forecasting (STLF) plays a vital role in resource allocation, capital budgeting of power companies, energy deployment and government control. In recent decades, the strong dependency relationships of time series have been considered in many researches, but the discrete information has not proven to be very useful in their experiments. In general, while discrete information is weak, it can provide macro trends compared to the micro trends of continuous information. In this research, we aim to combine macro and micro information by continuous and discrete time series to generate multiple time series (MTS). The MTS comprise four information sequences: short-term, cycle, long short-term and cross-long short-term. These MTS are used to build a STLF system using a recurrent neural network (RNN) model that can learn sequential information between continuous and discrete series. Therefore, the RNN model with MTS information can improve the forecasting performance for short-term load forecasting. The experimental results show that our proposed forecasting system outperforms the state-of-the-art approach.
更多查看译文
关键词
Multiple time series,Deep learning,Short-term load forecasting,Recurrent neural networks,Long short-term memory,Gated recurrent units
AI 理解论文
溯源树
样例
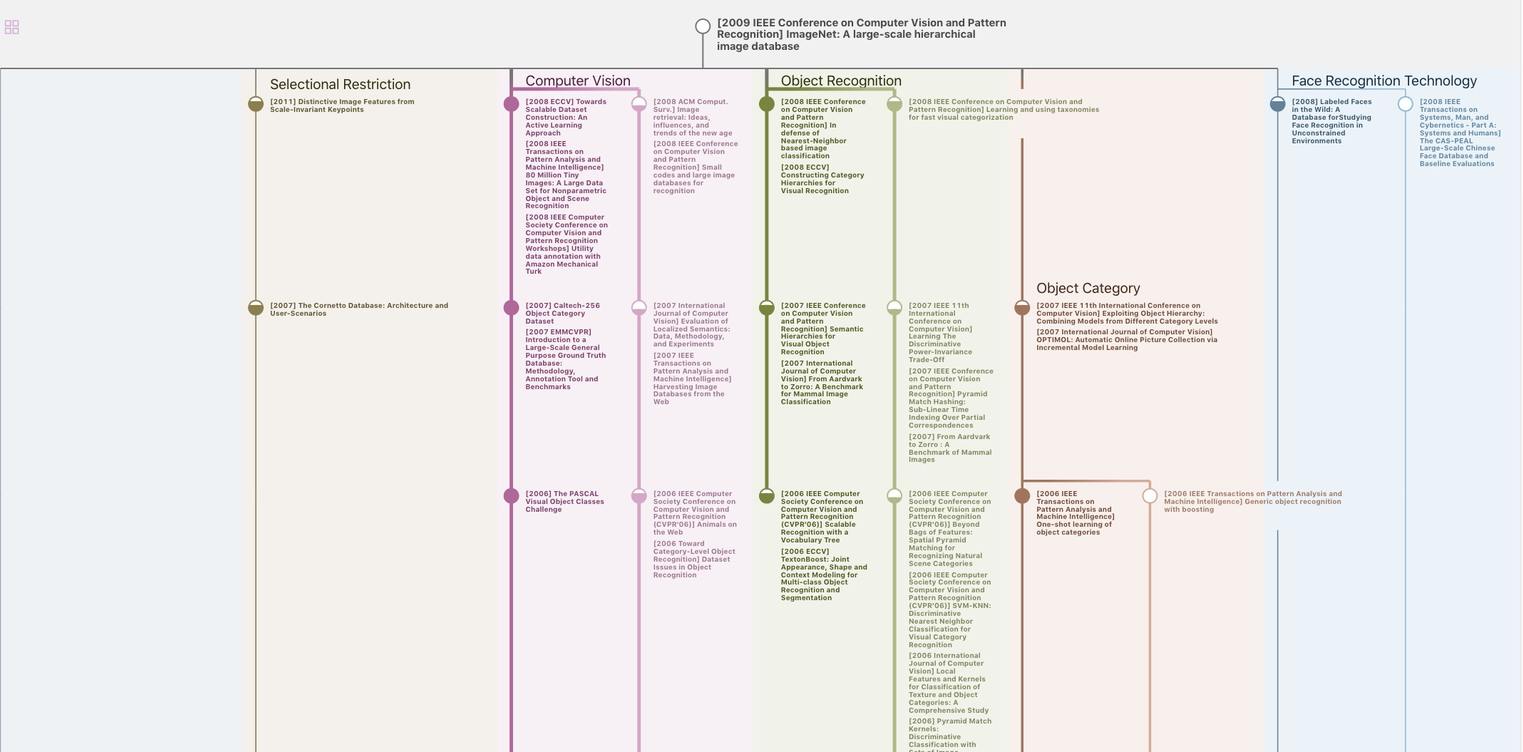
生成溯源树,研究论文发展脉络
Chat Paper
正在生成论文摘要