Detection of Compromised Smart Grid Devices with Machine Learning and Convolution Techniques
2018 IEEE International Conference on Communications (ICC)(2018)
摘要
The smart grid concept has transformed the traditional power grid into a massive cyber-physical system that depends on advanced two-way communication infrastructure to integrate a myriad of different smart devices. While the introduction of the cyber component has made the grid much more flexible and efficient with so many smart devices, it also broadened the attack surface of the power grid. Particularly, compromised devices pose a great danger to the healthy operations of the smart-grid. For instance, the attackers can control the devices to change the behaviour of the grid and can impact the measurements. In this paper, to detect such misbehaving malicious smart grid devices, we propose a machine learning and convolution-based classification framework. Our framework specifically utilizes system and library call lists at the kernel level of the operating system on both resource-limited and resource-rich smart grid devices such as RTUs, PLCs, PMUs, and IEDs. Focusing on the types and other valuable features extracted from the system calls, the framework can successfully identify malicious smart-grid devices. In order to test the efficacy of the proposed framework, we built a representative testbed conforming to the IEC-61850 protocol suite and evaluated its performance with different system calls. The proposed framework in different evaluation scenarios yields very high accuracy (avg. 91 is effective to overcome compromised smart grid devices problem.
更多查看译文
关键词
Smart Grid,Compromised Devices,Cybersecurity,Machine Learning,Call List
AI 理解论文
溯源树
样例
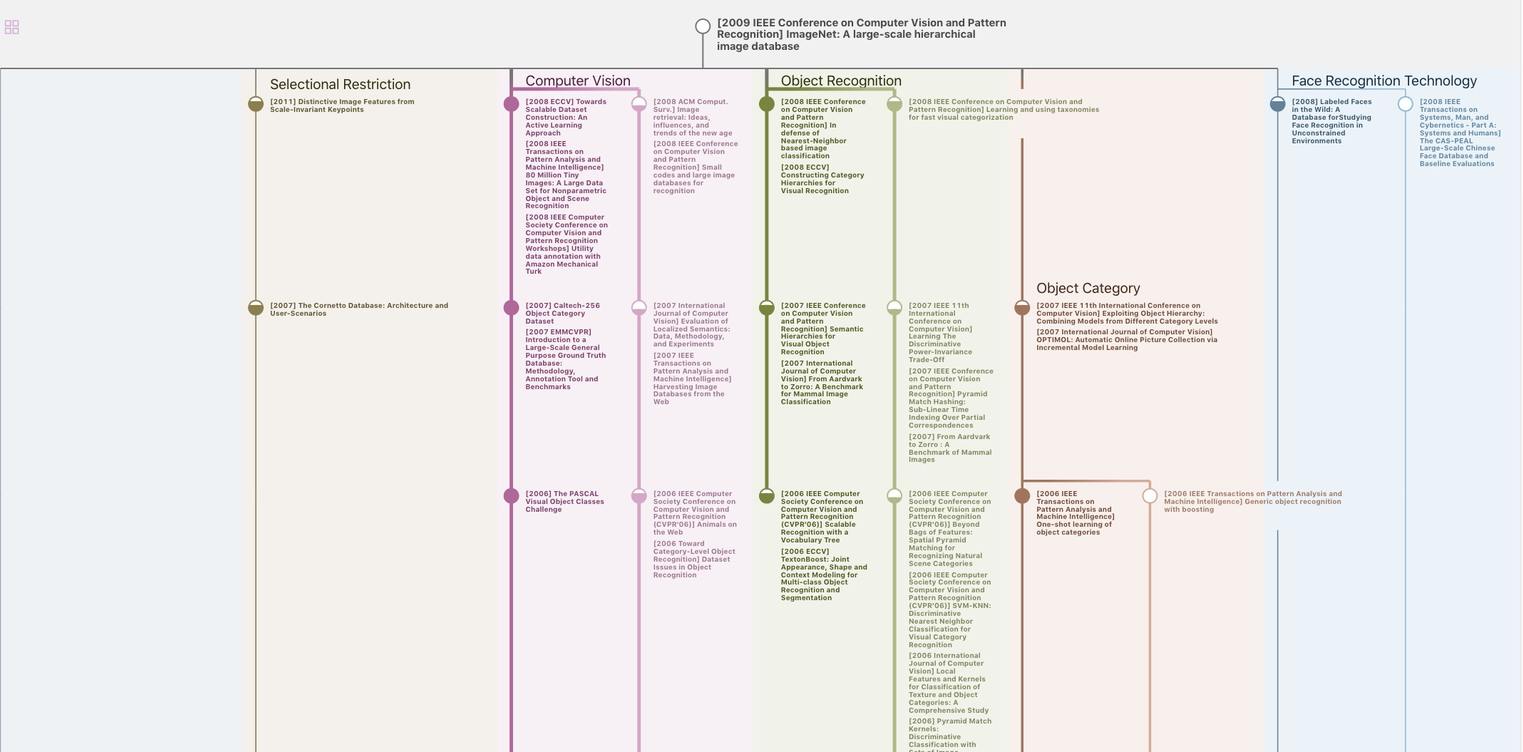
生成溯源树,研究论文发展脉络
Chat Paper
正在生成论文摘要