Leveraging External Knowledge For Phrase-Based Topic Modeling
2017 CONFERENCE ON TECHNOLOGIES AND APPLICATIONS OF ARTIFICIAL INTELLIGENCE (TAAI)(2017)
摘要
Topic modeling has been widely used for extracting the major topics from a corpus. Each discovered topic contains a set of related individual words that describe the topic itself. The discovered topics summarize the major themes of the corpus. Recently, a few phrase-based topic models have been proposed, which simultaneously model phrases and topics. The topics discovered by these models consist of phrases besides individual words, as phrases are typically more meaningful. However, these models typically require large amounts of data to provide reliable statistics for phrase-based topic modeling, thus limiting their performance in scenarios with limited data. To address this limitation, we propose a knowledge-based topic model that incorporates two types of pre-identilied external knowledge for topical phrase discovery: Phrase knowledge, and phrase correlation knowledge. Phrase knowledge guides the discovery of meaningful phrases by leveraging a set of pre-identified exemplary phrases; Phrase correlation knowledge guides the discovery of meaningful topics by exploiting a set of pre-identified pairs of related phrases. Experimental results show that our method outperforms the state-of-the-art baseline on both small and large datasets, extracting more meaningful phrases and coherent topics.
更多查看译文
关键词
topic modeling, phrase modeling, opinion mining
AI 理解论文
溯源树
样例
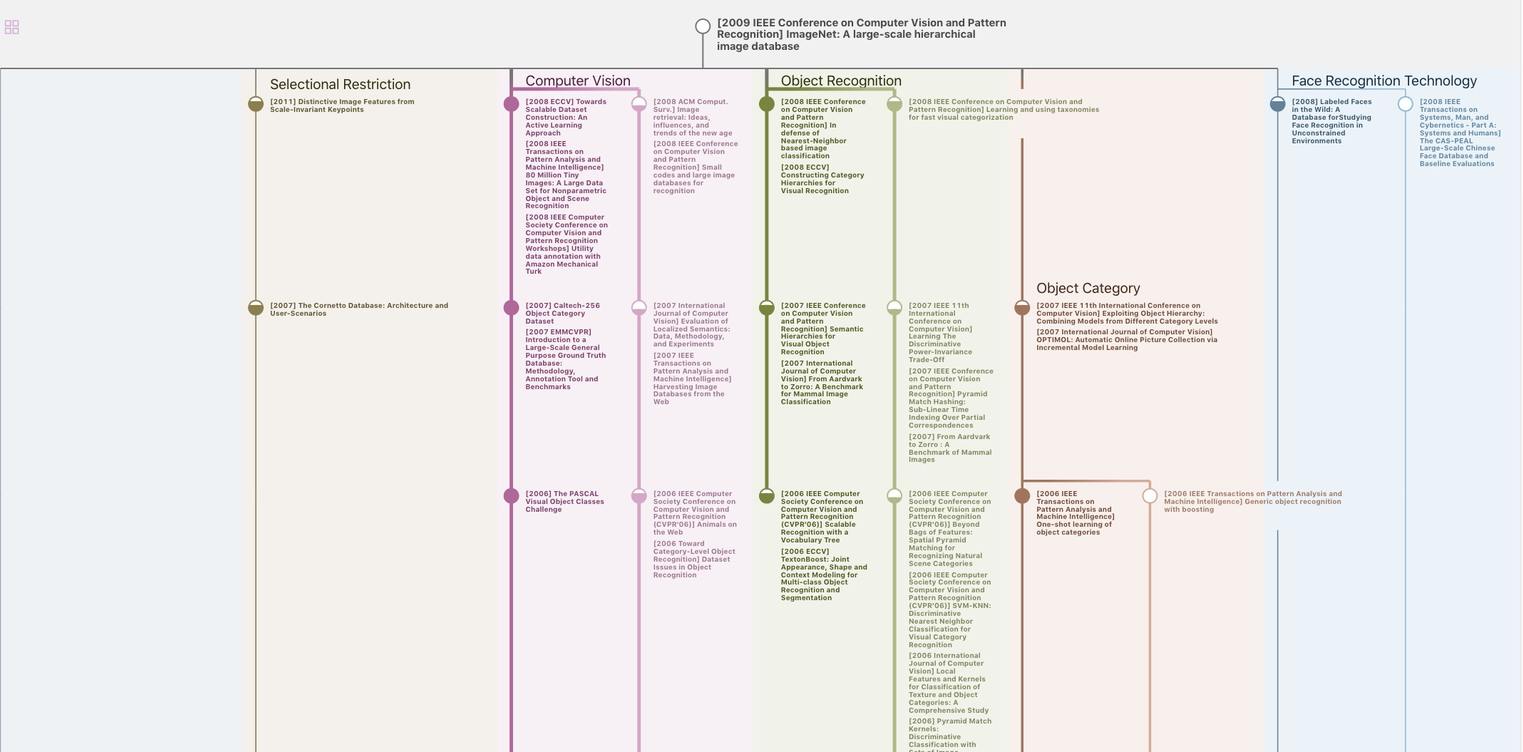
生成溯源树,研究论文发展脉络
Chat Paper
正在生成论文摘要