Formula Ranking within an Article.
JCDL(2018)
摘要
With the ever-increasing volume of formulae on the Web, formula retrieval has drawn much attention from researchers. However, most of the existing researches on formula retrieval treat each formula within an article equally, while different formulae in the same article have different importance to the article. In this paper, we address the issue to rank formulae within an article based on their importance. To evaluate the importance of each formula within an article, a formula citation graph is firstly built in a large scale corpus. And the inter-articles features of formulae are extracted by the link topology analysis of formulae based on the graph. Then the word embedding model is explored to extract the inner-article features by mining the semantic relevance between a formula and the corresponding article. Finally, we leverage learning to rank technique for formulae ranking within an article based on those features. The experimental results demonstrate that the proposed features are helpful for formula ranking and our approach yields better performance compared with other state-of-the-art methods.
更多查看译文
关键词
Formula Importance,Formula Ranking,Formula Citation Graph
AI 理解论文
溯源树
样例
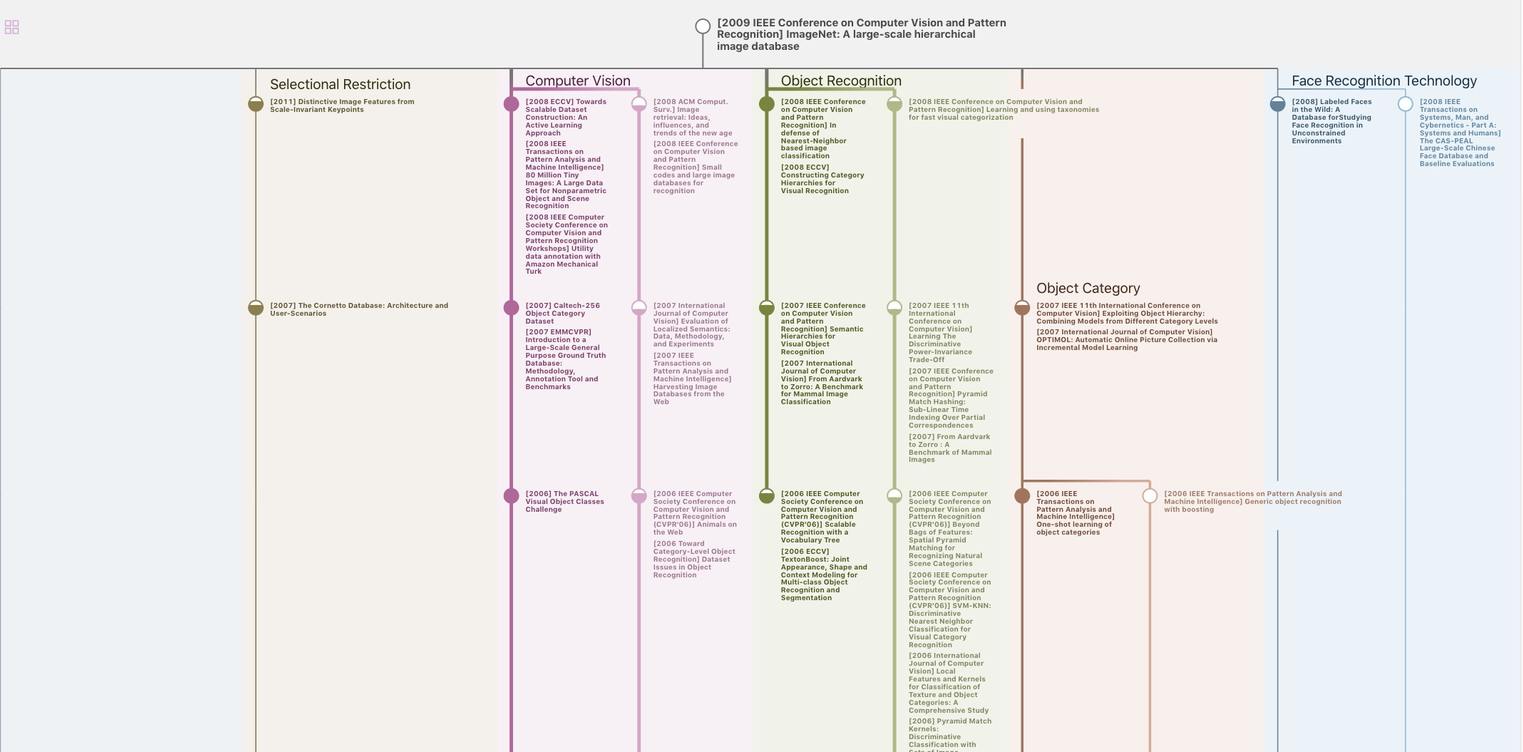
生成溯源树,研究论文发展脉络
Chat Paper
正在生成论文摘要