Bandit Assignment for Educational Experiments: Benefits to Students Versus Statistical Power.
AIED(2018)
摘要
Randomized experiments can lead to improvements in educational technologies, but often require many students to experience conditions associated with inferior learning outcomes. Multi-armed bandit (MAB) algorithms can address this by modifying experimental designs to direct more students to more helpful conditions. Using simulations and modeling data from previous educational experiments, we explore the statistical impact of using MABs for experiment design, focusing on the tradeoff between acquiring statistically reliable information and benefits to students. Results suggest that while MAB experiments can improve average benefits for students, at least twice as many participants are needed to attain power of 0.8 and false positives are twice as frequent as expected. Optimistic prior distributions in the MAB algorithm can mitigate the loss in power to some extent, without meaningfully reducing benefits or further increasing false positives.
更多查看译文
AI 理解论文
溯源树
样例
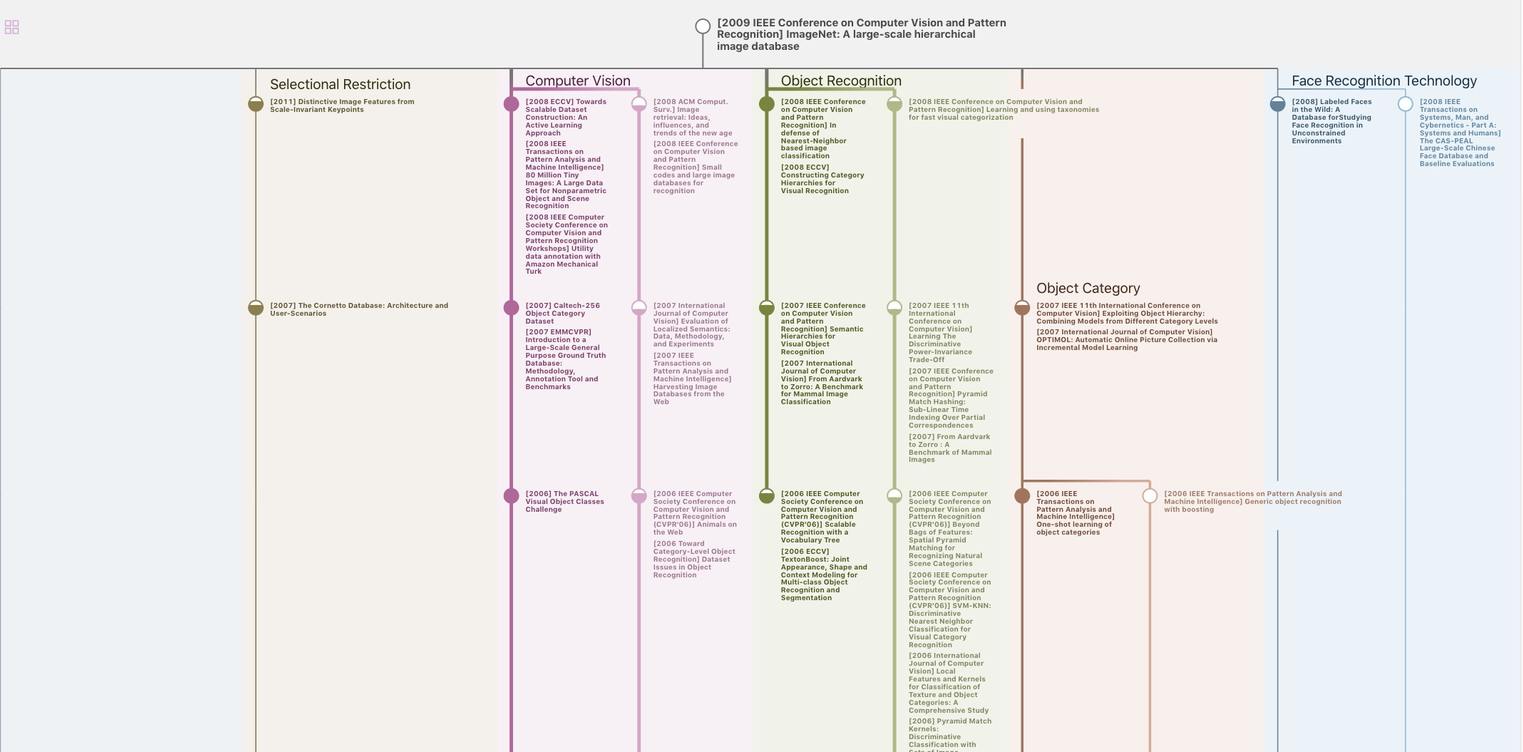
生成溯源树,研究论文发展脉络
Chat Paper
正在生成论文摘要