An Optimal Algorithm for Online Non-Convex Learning.
SIGMETRICS (Abstracts)(2018)
摘要
In many online learning paradigms, convexity plays a central role in the derivation and analysis of online learning algorithms. The results, however, fail to be extended to the non-convex settings, which are necessitated by tons of recent applications. The Online Non-Convex Learning problem generalizes the classic Online Convex Optimization framework by relaxing the convexity assumption on the cost function (to a Lipschitz continuous function) and the decision set. The state-of-the-art result for ønco demonstrates that the classic Hedge algorithm attains a sublinear regret of O(√T log T). The regret lower bound for øco, however, is Omega(√T), and to the best of our knowledge, there is no result in the context of the ønco problem achieving the same bound. This paper proposes the Online Recursive Weighting algorithm with regret of O(√T), matching the tight regret lower bound for the øco problem, and fills the regret gap between the state-of-the-art results in the online convex and non-convex optimization problems.
更多查看译文
关键词
expert problem, lipschitz loss function, metric space, online convex optimization, online non-convex learning, online recursive weighting, regret
AI 理解论文
溯源树
样例
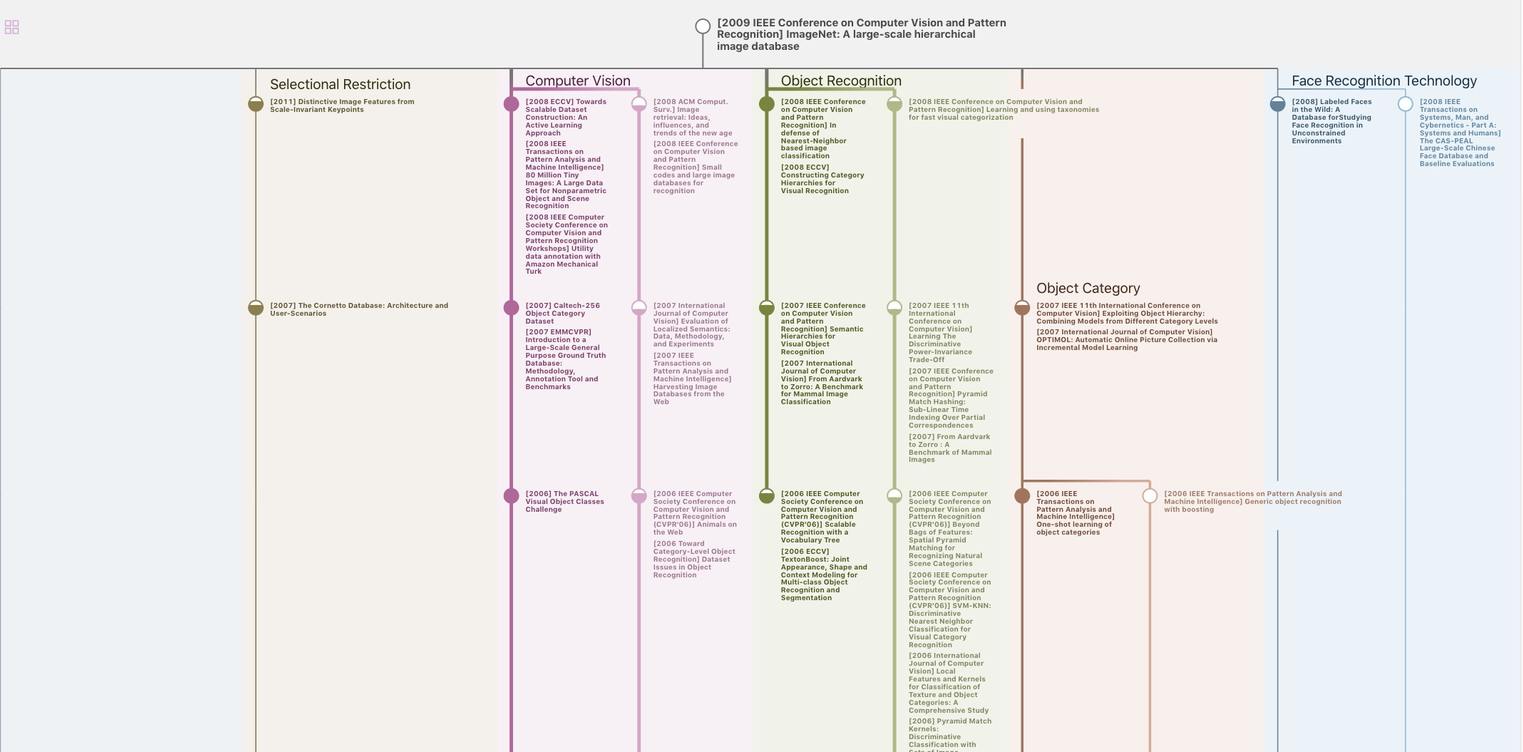
生成溯源树,研究论文发展脉络
Chat Paper
正在生成论文摘要