Energy and Area Efficiency in Neuromorphic Computing for Resource Constrained Devices.
ACM Great Lakes Symposium on VLSI(2018)
摘要
Resource constrained devices are the building blocks of the internet of things (IoT) era. Since the idea behind IoT is to develop an interconnected environment where the devices are tiny enough to operate with limited resources, several control systems have been built to maintain low energy and area consumption while operating as IoT edge devices. Several researchers have begun work on implementing control systems built from resource constrained devices using machine learning. However, there are many ways such devices can achieve lower power consumption and area utilization while maximizing application efficiency. Spiky neuromorphic computing (SNC) is an emerging paradigm that can be leveraged in resource constrained devices for several emerging applications. While delivering the benefits of machine learning, SNC also helps minimize power consumption. For example, low energy memory devices (memristors) are often used to achieve low power operation and also help in reducing system area. In total, we anticipate SNC will provide computational efficiency approaching that of deep learning while using low power, resource constrained devices.
更多查看译文
关键词
Neuromorphic, memristor, internet of things, machine learning
AI 理解论文
溯源树
样例
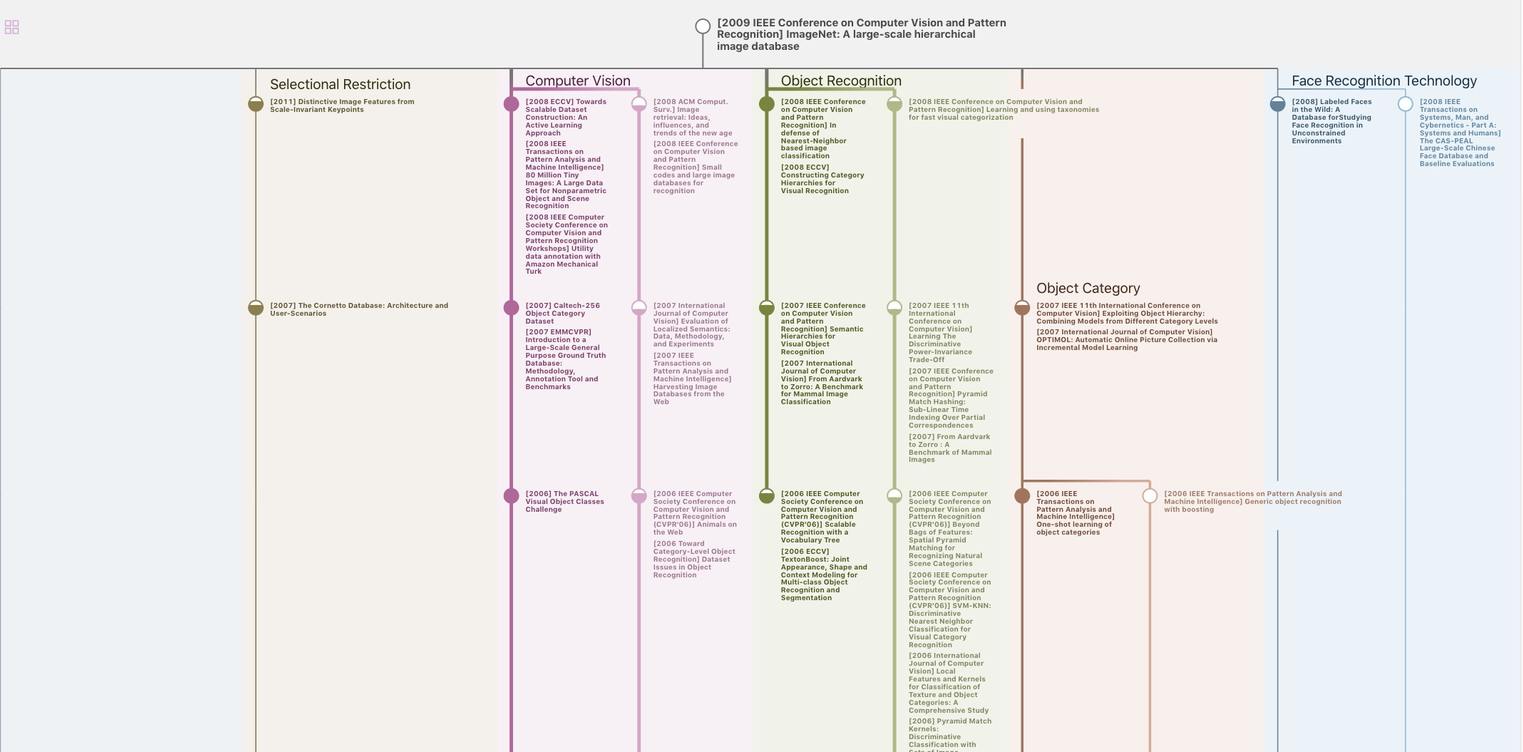
生成溯源树,研究论文发展脉络
Chat Paper
正在生成论文摘要