UFSSF - An Efficient Unsupervised Feature Selection for Streaming Features.
ADVANCES IN KNOWLEDGE DISCOVERY AND DATA MINING, PAKDD 2018, PT II(2018)
摘要
Streaming features applications pose challenges for feature selection. For such dynamic features applications: (a) features are sequentially generated and are processed one by one upon their arrival while the number of instances/ points remains fixed; and (b) the complete feature space is not known in advance. Existing approaches require class labels as a guide to select the representative features. However, in real-world applications most data are not labeled and, moreover, manual labeling is costly. A new algorithm, called Unsupervised Feature Selection for Streaming Features (UFSSF), is proposed in this paper to select representative features in streaming features applications without the need to know the features or class labels in advance. UFSSF extends the k-mean clustering algorithm to include linearly dependent similarity measures so as to incrementally decide whether to add the newly arrived feature to the existing set of representative features. Those features that are not representative are discarded. Experimental results indicates that UFSSF significantly has a better prediction accuracy and running time compared to the baseline approaches.
更多查看译文
关键词
Streaming Features, Unsupervised Feature Selection Approach, Require Class Labels, Complete Feature Space, Baseline Approach
AI 理解论文
溯源树
样例
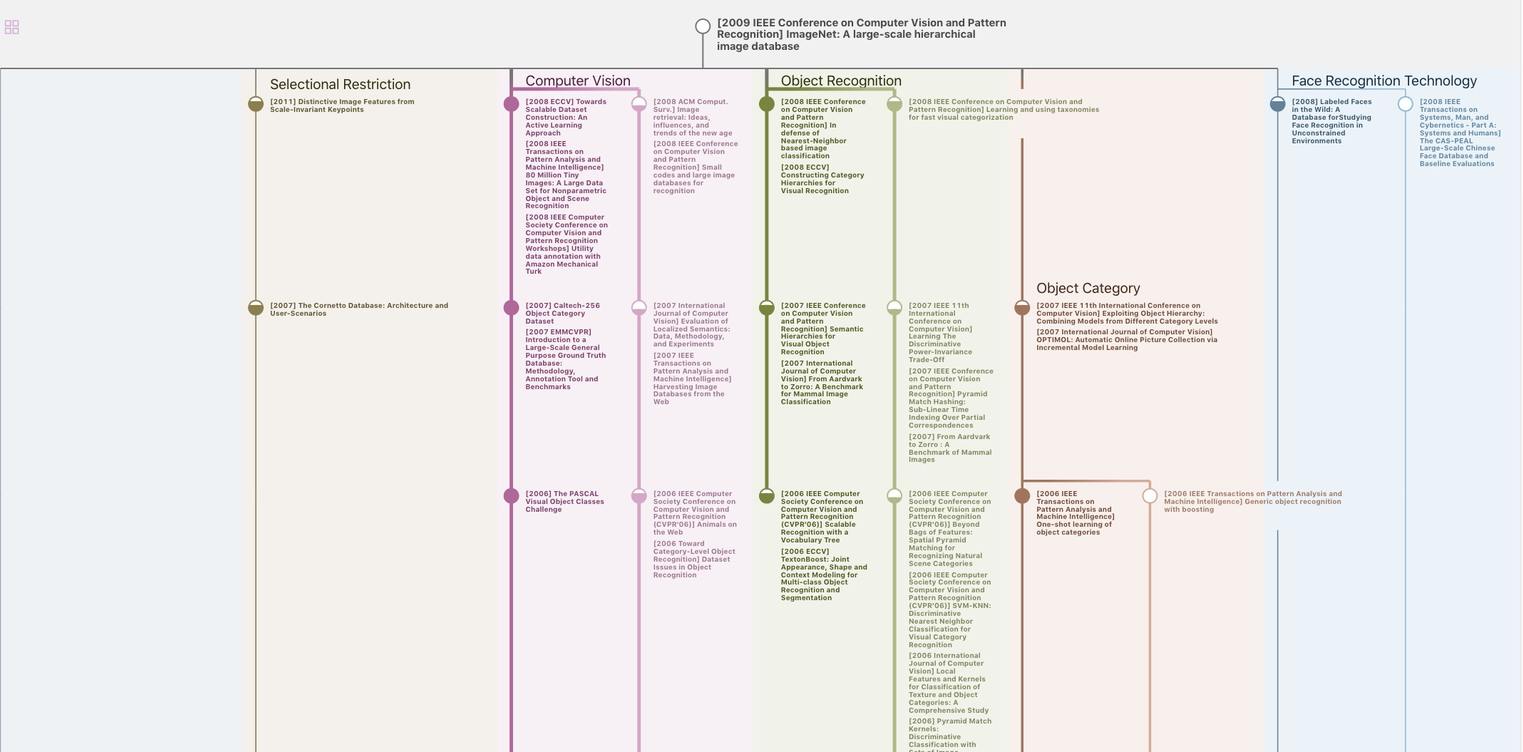
生成溯源树,研究论文发展脉络
Chat Paper
正在生成论文摘要