Adaptive Attention Network for Review Sentiment Classification.
ADVANCES IN KNOWLEDGE DISCOVERY AND DATA MINING, PAKDD 2018, PT I(2018)
摘要
Document-level sentiment classification is an important NLP task. The state of the art shows that attention mechanism is particularly effective on document-level sentiment classification. Despite the success of previous attention mechanism, it neglects the correlations among inputs (e.g., words in a sentence), which can be useful for improving the classification result. In this paper, we propose a novel Adaptive Attention Network (AAN) to explicitly model the correlations among inputs. Our AAN has a two-layer attention hierarchy. It first learns an attention score for each input. Given each input's embedding and attention score, it then computes a weighted sum over all the words' embeddings. This weighted sum is seen as a "context" embedding, aggregating all the inputs. Finally, to model the correlations among inputs, it computes another attention score for each input, based on the input embedding and the context embedding. These new attention scores are our final output of AAN. In document-level sentiment classification, we apply AAN to model words in a sentence and sentences in a review. We evaluate AAN on three public data sets, and show that it outperforms state-of-the-art baselines.
更多查看译文
AI 理解论文
溯源树
样例
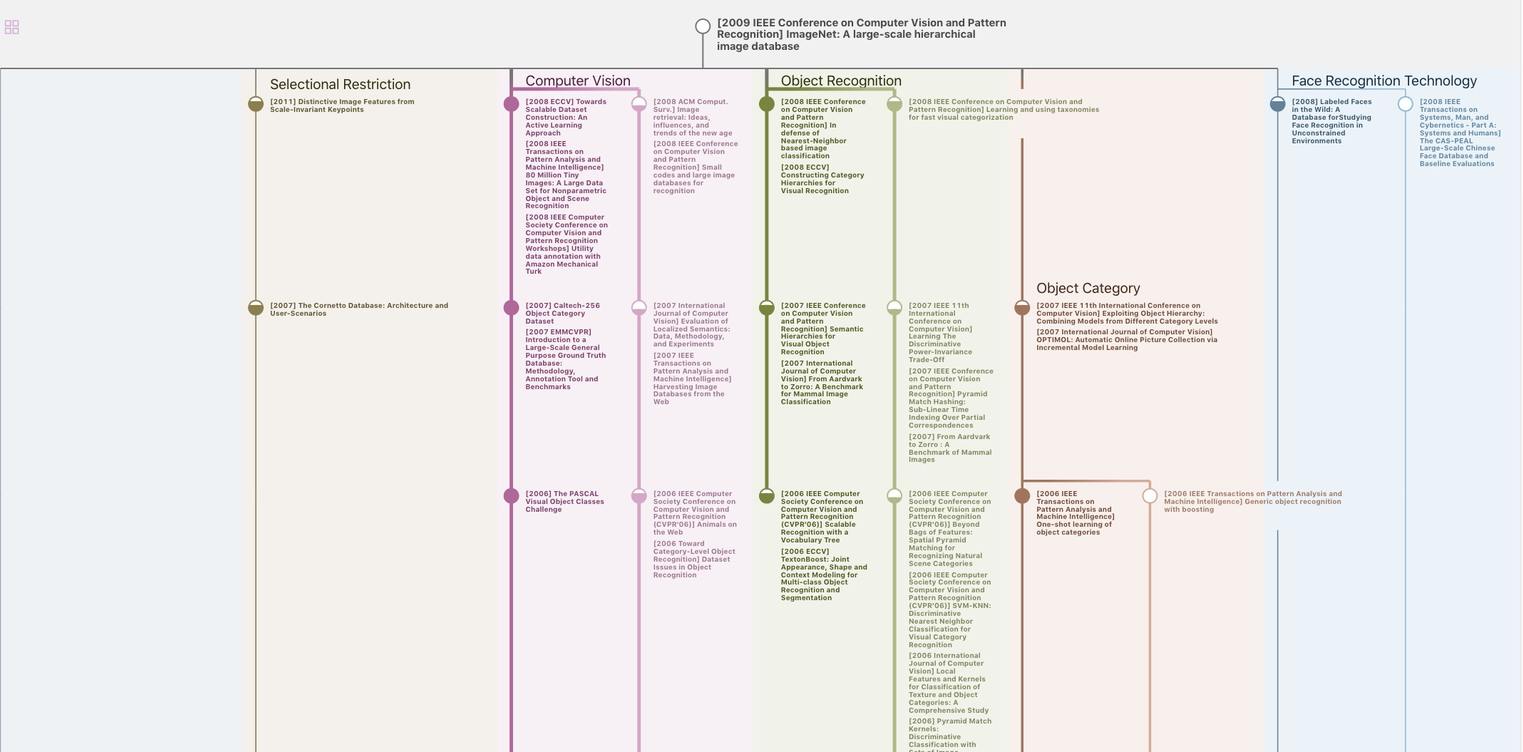
生成溯源树,研究论文发展脉络
Chat Paper
正在生成论文摘要