Ir Drop Prediction Of Eco-Revised Circuits Using Machine Learning
2018 IEEE 36TH VLSI TEST SYMPOSIUM (VTS 2018)(2018)
摘要
Excessive power supply noise (PSN), such as IR drop, can cause timing violation in VLSI chips. However, simulation PSN takes a very long time, especially when multiple iterations are needed in IR drop signoff. In this work, we propose a machine learning technique to build an IR drop prediction model based on circuits before ECO (engineer change order) revision. After revision, we can re-use this model to predict the IR drop of the revised circuit. Because the previous circuit(s) and the revised circuit are very similar, the model can be applied with small error. We proposed seven feature extractions, which are simple and scalable for large designs. Our experiment results show that prediction accuracy (average error 3.7mV) and correlation (0.55) are very high for a three million-gate real design. The run time speedup is up to 30X. The proposed method is very useful for designers to save the simulation time when fixing the IR drop problem.
更多查看译文
关键词
power supply noise, IR drop analyzer, machine learning
AI 理解论文
溯源树
样例
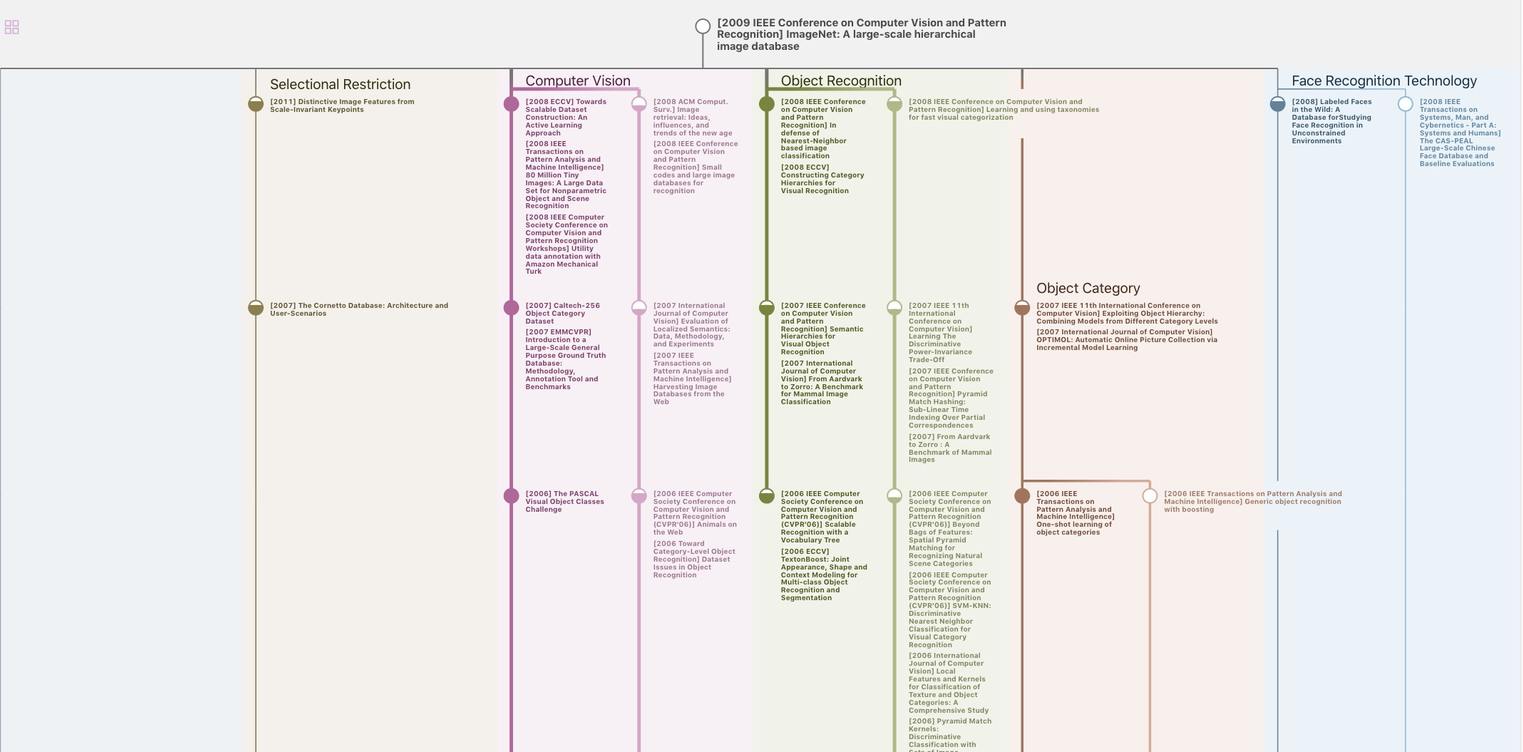
生成溯源树,研究论文发展脉络
Chat Paper
正在生成论文摘要