Unsupervised Domain Adaptation with Regularized Optimal Transport for Multimodal 2D+3D Facial Expression Recognition
2018 13th IEEE International Conference on Automatic Face & Gesture Recognition (FG 2018)(2018)
摘要
Since human expressions have strong flexibility and personality, subject-independent facial expression recognition is a typical data bias problem. To address this problem, we propose a novel approach, namely unsupervised domain adaptation with regularized optimal transport for multimodal 2D+3D Facial Expression Recognition (FER). In particular, Wasserstein distance is employed to measure the distribution inconsistency between the training samples (i.e. source domain) and test samples (i.e. target domain). Minimization of this Wasserstein distance is equivalent to finding an optimal transport mapping from training to test samples. Once we find this mapping, original training samples can be transformed into a new space in which the distributions of the mapped training samples and the test samples can be well-aligned. In this case, classifier learned from the transformed training samples can be well generalized to the test samples for expression prediction. In practice, approximate optimal transport can be effectively solved by adding entropy regularization. To fully explore the class label information of training samples, group sparsity regularizer is also used to enforce that the training samples from the same expression class can be mapped to the same group. Experimental results evaluated on the BU-3DFE and Bosphorus databases demonstrate that the proposed approach can achieve superior performance compared with the state-of-the-art methods.
更多查看译文
关键词
domain adaptation,optimal transport,facial expression recognition
AI 理解论文
溯源树
样例
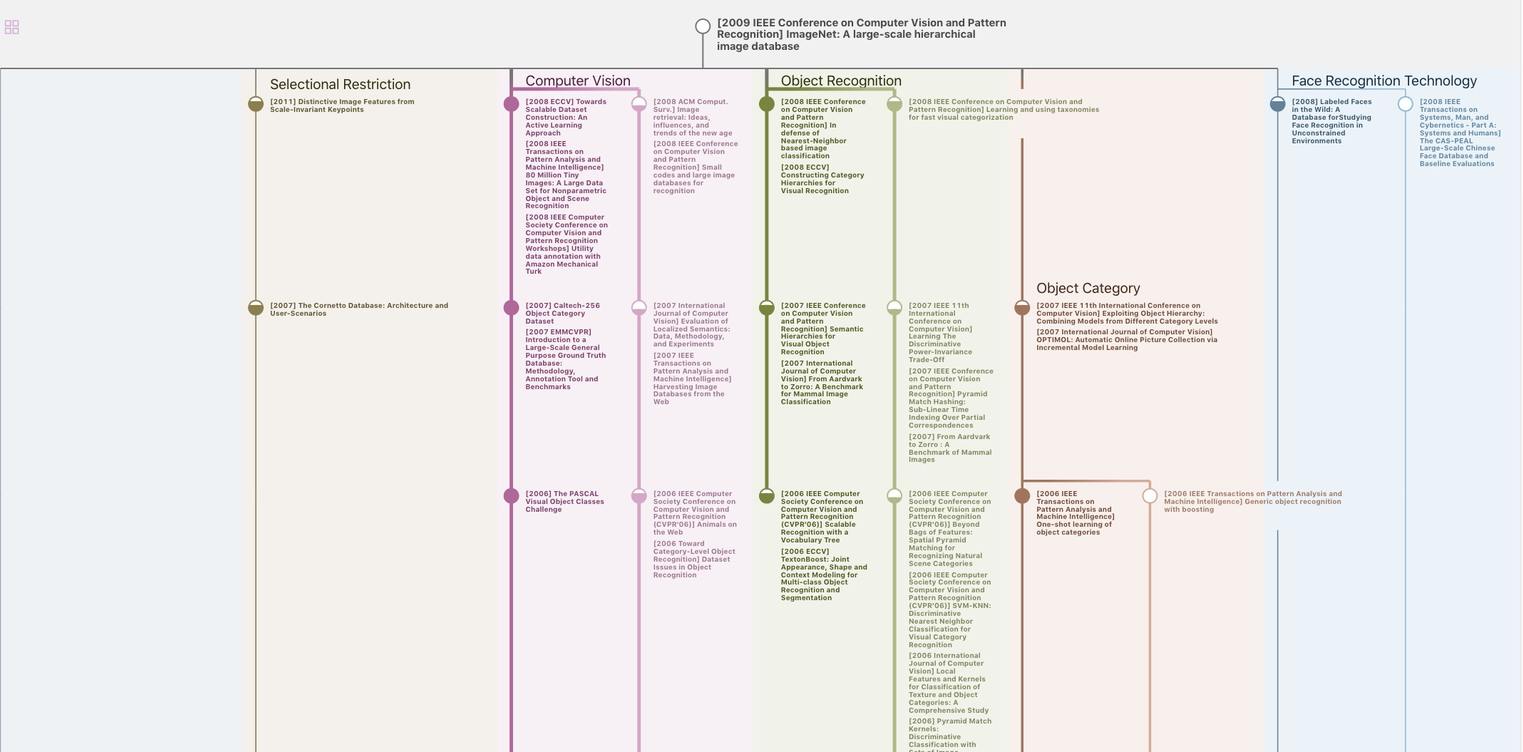
生成溯源树,研究论文发展脉络
Chat Paper
正在生成论文摘要