Baseline Detection in Historical Documents using Convolutional U-Nets
2018 13th IAPR International Workshop on Document Analysis Systems (DAS)(2018)
摘要
Baseline detection is still a challenging task for heterogeneous collections of historical documents. We present a novel approach to baseline extraction in such settings, turning out the winning entry to the ICDAR 2017 Competition on Baseline detection (cBAD). It utilizes deep convolutional nets (CNNs) for both, the actual extraction of baselines, as well as for a simple form of layout analysis in a pre-processing step. To the best of our knowledge it is the first CNN-based system for baseline extraction applying a U-net architecture and sliding window detection, profiting from a high local accuracy of the candidate lines extracted. Final baseline post-processing complements our approach, compensating for inaccuracies mainly due to missing context information during sliding window detection. We experimentally evaluate the components of our system individually on the cBAD dataset. Moreover, we investigate how it generalizes to different data by means of the dataset used for the baseline extraction task of the ICDAR 2017 Competition on Layout Analysis for Challenging Medieval Manuscripts (HisDoc). A comparison with the results reported for HisDoc shows that it also outperforms the contestants of the latter.
更多查看译文
关键词
Historical Document Analysis,Baseline Extraction,Deep Neural Networks
AI 理解论文
溯源树
样例
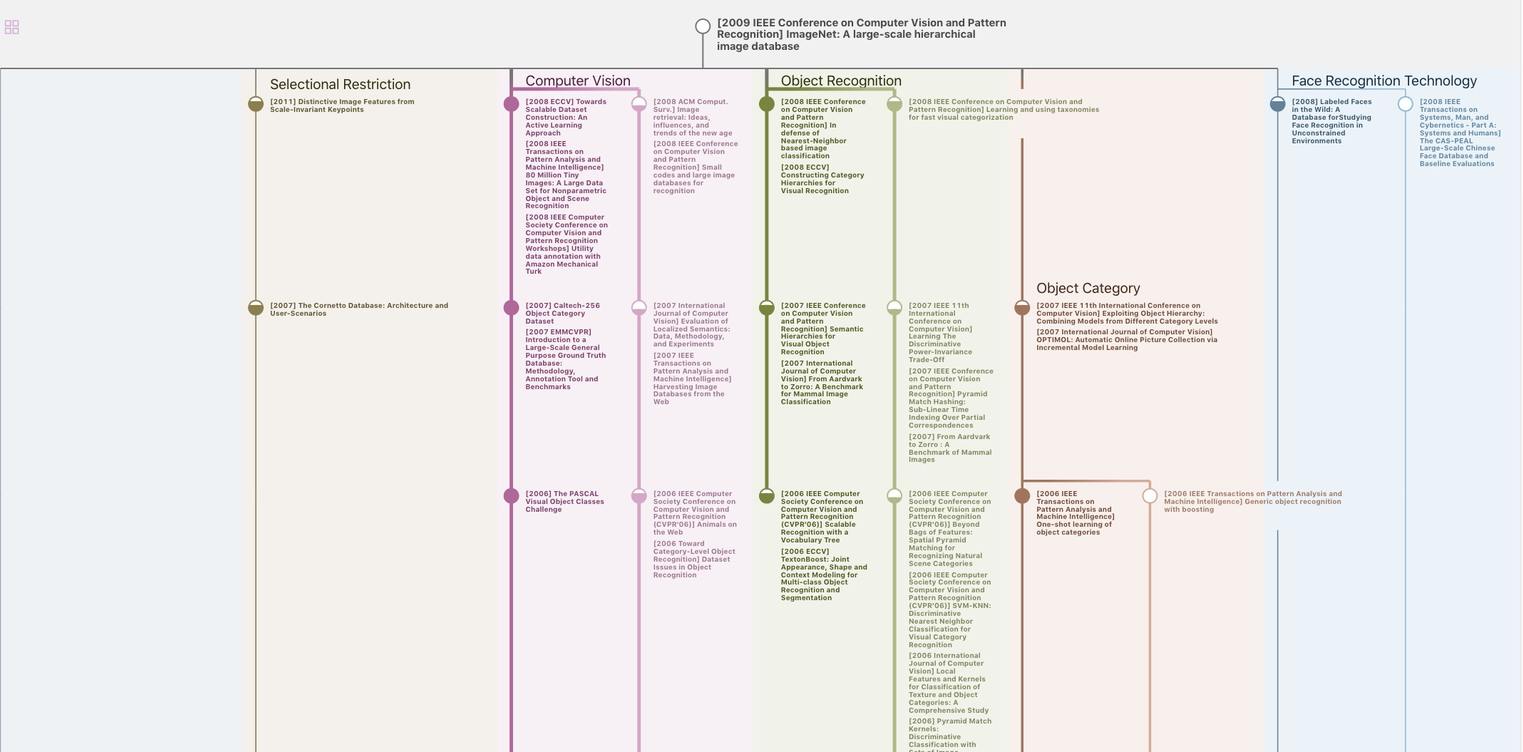
生成溯源树,研究论文发展脉络
Chat Paper
正在生成论文摘要