Dyhard-Dnn: Even More Dnn Acceleration With Dynamic Hardware Reconfiguration
2018 55TH ACM/ESDA/IEEE DESIGN AUTOMATION CONFERENCE (DAC)(2018)
摘要
Deep Neural Networks (DNNs) have demonstrated their utility across a wide range of input data types, usable across diverse computing substrates, from edge devices to datacenters. This broad utility has resulted in myriad hardware accelerator architectures. However, DNNs exhibit significant heterogeneity in their computational characteristics, e.g., feature and kernel dimensions, and dramatic variances in computational intensity, even between adjacent layers in one DNN. Consequently, accelerators with static hardware parameters run sub-optimally and leave energy efficiency margins unclaimed. We propose DyHard-DNNs, where accelerator microarchitectural parameters are dynamically reconfigured during DNN execution to significantly improve metrics of interest. We demonstrate the effectiveness of this approach on a configurable SIMD 2D systolic array and show a 15-65% performance improvement (at iso-power) and 25-90% energy improvement (at iso-latency) over the best static configuration in six mainstream DNN workloads.
更多查看译文
关键词
diverse computing substrates,datacenters,myriad hardware accelerator architectures,kernel dimensions,dramatic variances,computational intensity,static hardware parameters,energy-efficiency margins,DyHard-DNN,accelerator microarchitectural parameters,DNN execution,static configuration,mainstream DNN workloads,DNN acceleration,dynamic hardware reconfiguration,feature dimensions,SIMD 2D systolic array,performance improvement,energy improvement,deep neural networks,computational characteristics
AI 理解论文
溯源树
样例
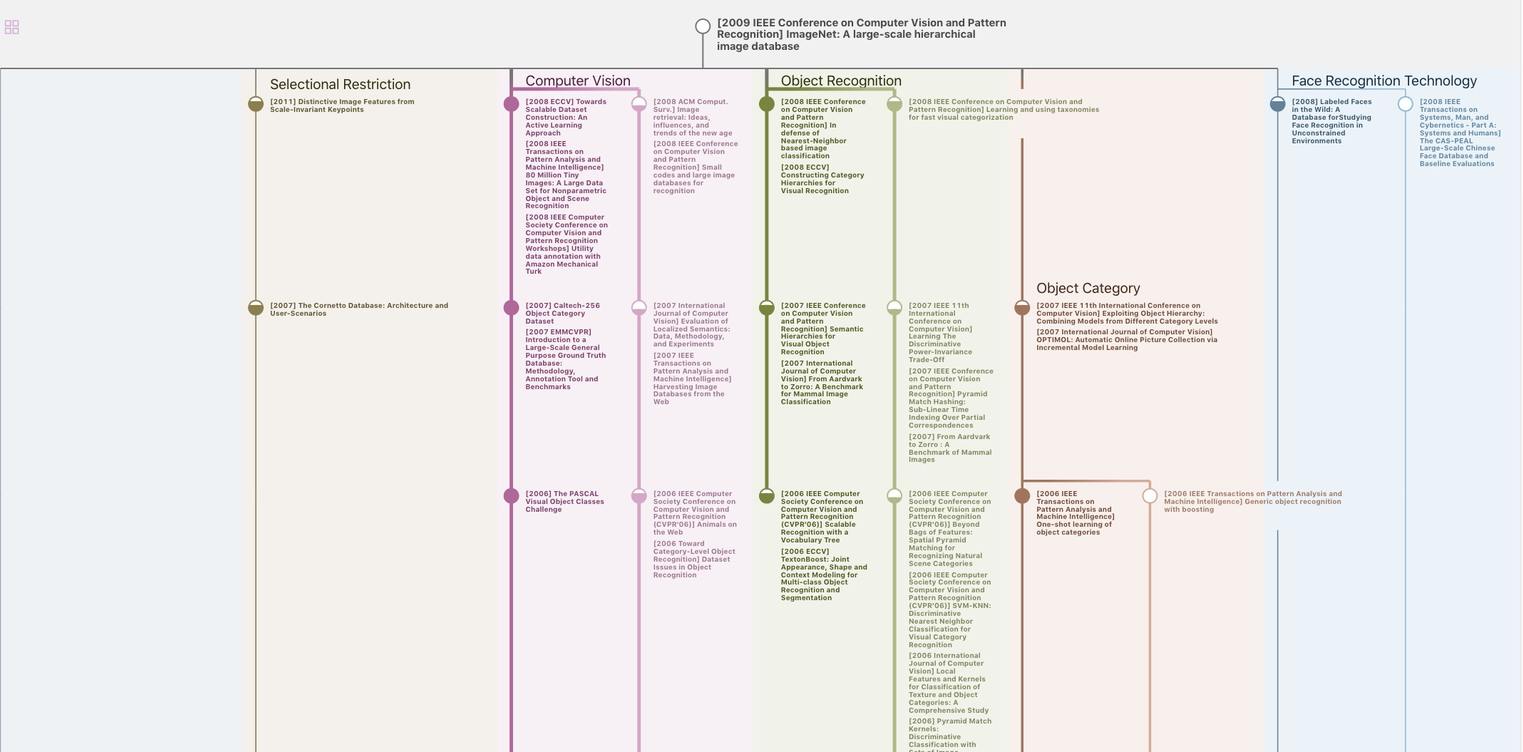
生成溯源树,研究论文发展脉络
Chat Paper
正在生成论文摘要