Modeling of Decline Dynamics of Knowledge Sharing Networks (KSNets) - A Wikipedia Case
2018 5th International Conference on Control, Decision and Information Technologies (CoDIT)(2018)
摘要
Online knowledge sharing networks (KSNets) have made significant impacts on the economy as well as wellbeing of societies through sharing. One of the most successful KSNets is Wikipedia that allows users to create contents in a collaborative manner and to provide fast and easy access at no cost to users. Recent research, however, has shown that the numbers of “Wikipedians” and new page creations have been declining, reflecting decrease in user contributions and in new contents. To facilitate management for sustainability, this paper aims at quantitatively modeling how the decline in new contents affects the number of Wikipedians and in turn content creations, and predicting decline start time and speed based on available Wikipedia data. The novel modeling approach adopts auto-regression with an extended Bass Diffusion model (AREBDM) embedded to describe the Wikipedia-wide evolutions of the number of Wikipedians and content developments. Model parameters are then extracted by a nonlinear least square method from early Wikipedia data. Simulation predictions match well with actual Wikipedia decline trajectories of later stages. Our analysis shows that the decline of new page creation leads in time the decline of the number of new Wikipedians, and the decline speed increases with the decrease of new contents. Our approach therefore has the potential to predict decline time and speed so that proactive actions can be taken as early as possible.
更多查看译文
关键词
Wikipedia-wide evolutions,Wikipedians,content developments,model parameters,early Wikipedia data,simulation predictions,page creation,decline time,decline dynamics,Wikipedia case,online knowledge sharing networks,collaborative manner,user contributions,decline start time,extended Bass Diffusion model,KSNets,content creations,nonlinear least square method,simulation prediction,auto-regression
AI 理解论文
溯源树
样例
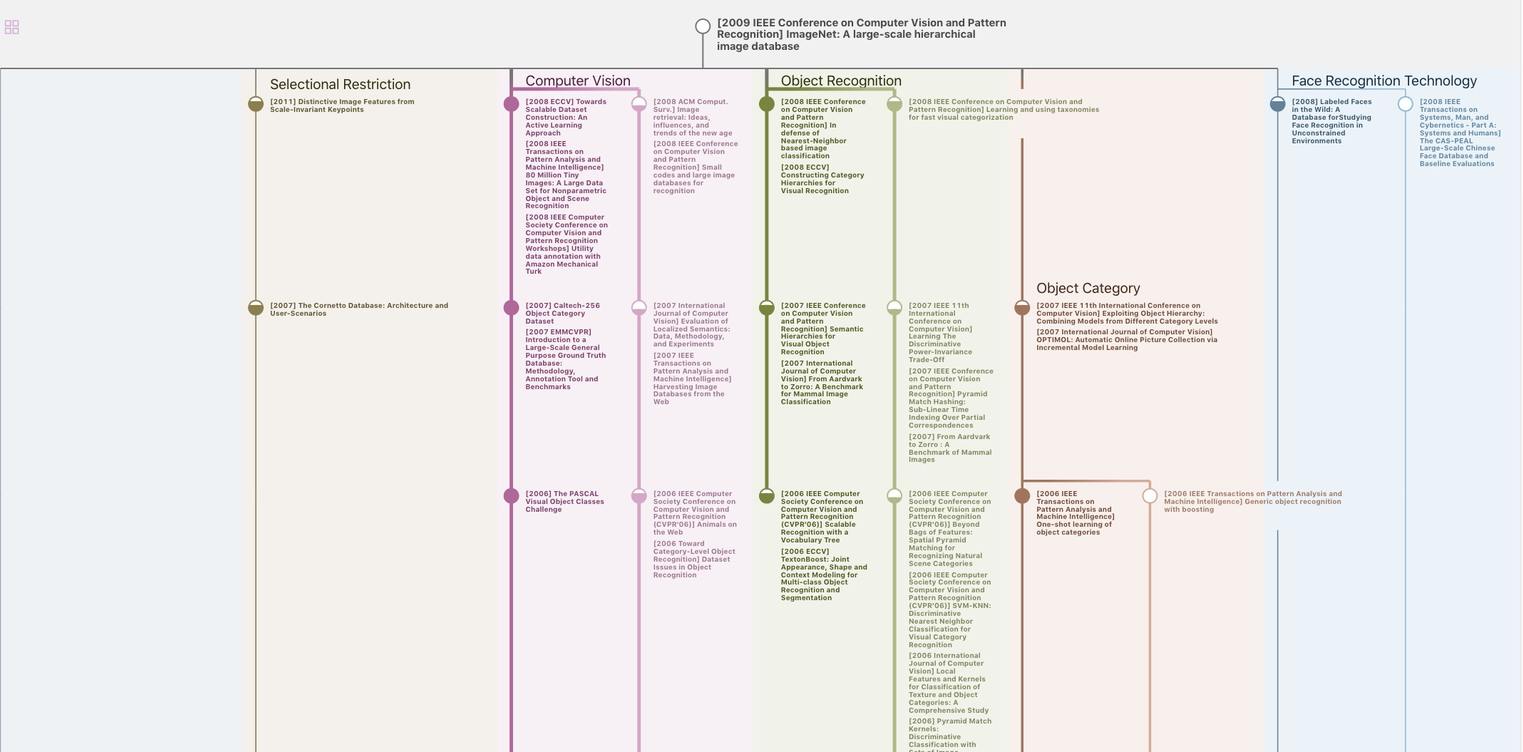
生成溯源树,研究论文发展脉络
Chat Paper
正在生成论文摘要