Scalable Bayesian Learning for State Space Models Using Variational Inference with SMC Samplers
International Conference on Artificial Intelligence and Statistics(2019)
摘要
We present a scalable approach to performing approximate fully Bayesian inference in generic state space models. The proposed method is an alternative to particle MCMC that provides fully Bayesian inference of both the dynamic latent states and the static parameters of the model. We build up on recent advances in computational statistics that combine variational methods with sequential Monte Carlo sampling and we demonstrate the advantages of performing full Bayesian inference over the static parameters rather than just performing variational EM approximations. We illustrate how our approach enables scalable inference in multivariate stochastic volatility models and self-exciting point process models that allow for flexible dynamics in the latent intensity function.
更多查看译文
AI 理解论文
溯源树
样例
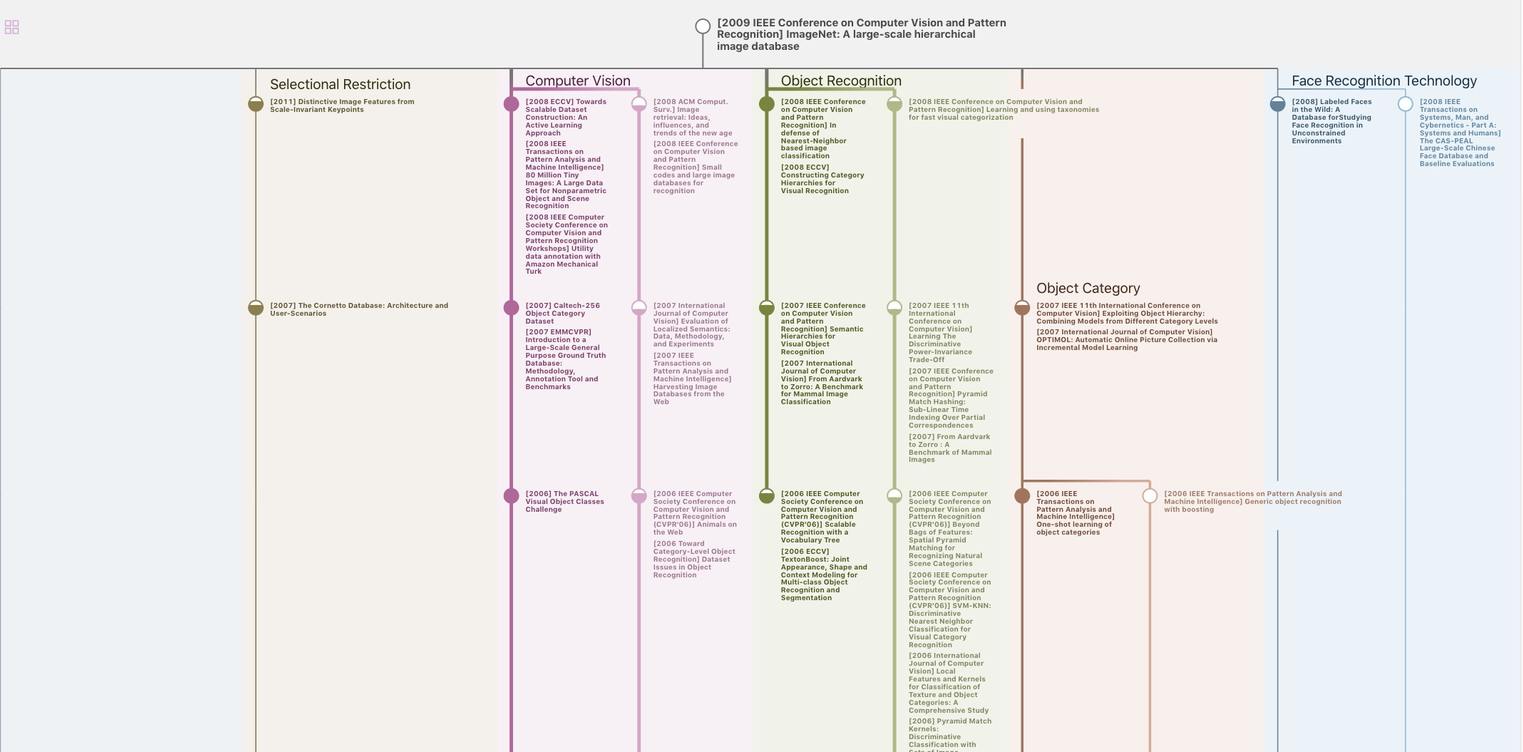
生成溯源树,研究论文发展脉络
Chat Paper
正在生成论文摘要