New Embedded Representations and Evaluation Protocols for Inferring Transitive Relations.
SIGIR(2018)
摘要
Beyond word embeddings, continuous representations of knowledge graph (KG) components, such as entities, types and relations, are widely used for entity mention disambiguation, relation inference and deep question answering. Great strides have been made in modeling general, asymmetric or antisymmetric KG relations using Gaussian, holographic, and complex embeddings. None of these directly enforce transitivity inherent in the is-instance-of and is-subtype-of relations. A recent proposal, called order embedding (OE), demands that the vector representing a subtype elementwise dominates the vector representing a supertype. However, the manner in which such constraints are asserted and evaluated have some limitations. In this short research note, we make three contributions specific to representing and inferring transitive relations. First, we propose and justify a significant improvement to the OE loss objective. Second, we propose a new representation of types as hyper-rectangular regions, that generalize and improve on OE. Third, we show that some current protocols to evaluate transitive relation inference can be misleading, and offer a sound alternative. Rather than use black-box deep learning modules off-the-shelf, we develop our training networks using elementary geometric considerations.
更多查看译文
关键词
relations,representations,evaluation protocols
AI 理解论文
溯源树
样例
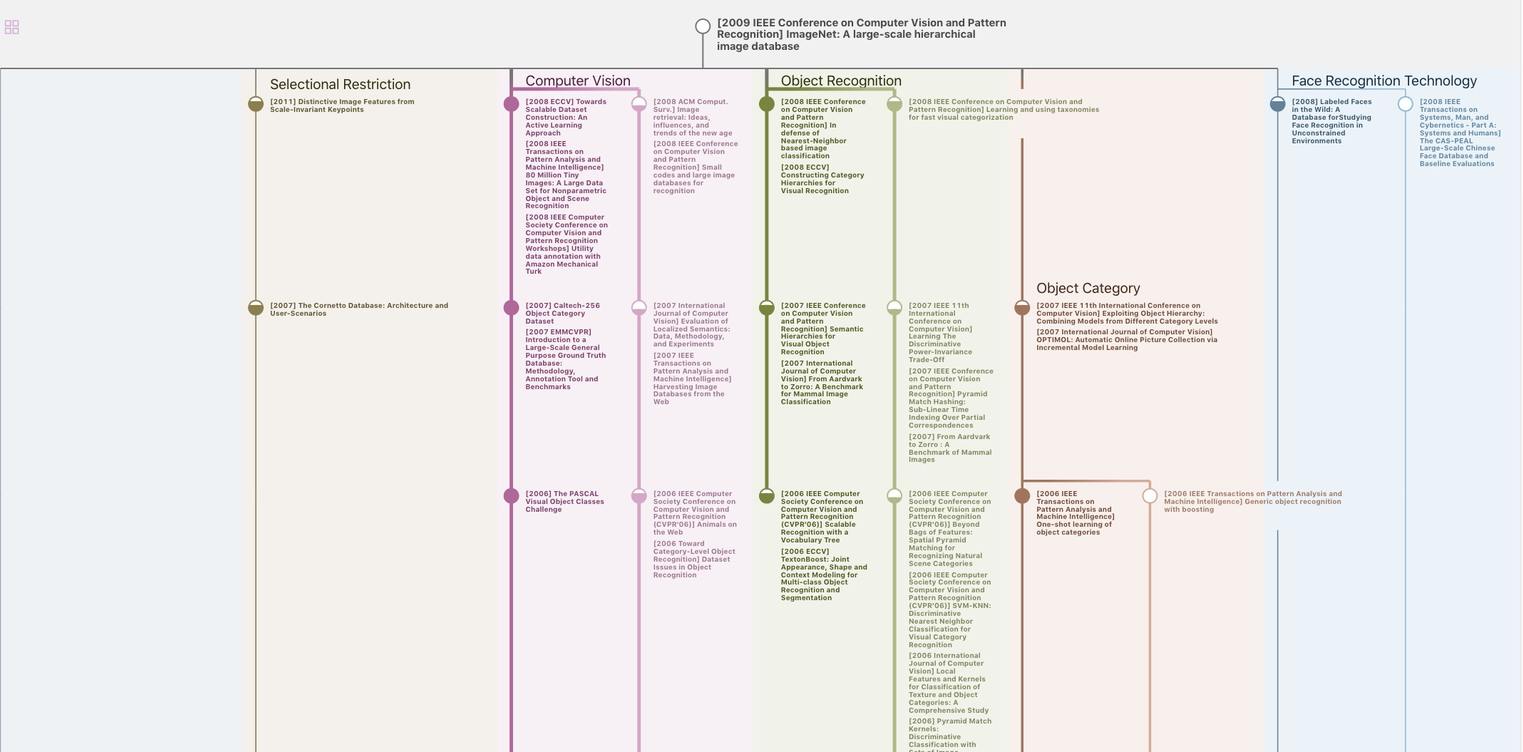
生成溯源树,研究论文发展脉络
Chat Paper
正在生成论文摘要