Parallel Graph Connectivity in Log Diameter Rounds
2018 IEEE 59th Annual Symposium on Foundations of Computer Science (FOCS)(2018)
摘要
Many modern parallel systems, such as MapReduce, Hadoop and Spark, can be modeled well by the MPC model. The MPC model captures well coarse-grained computation on large data — data is distributed to processors, each of which has a sublinear (in the input data) amount of memory and we alternate between rounds of computation and rounds of communication, where each machine can communicate an amount of data as large as the size of its memory. This model is stronger than the classical PRAM model, and it is an intriguing question to design algorithms whose running time is smaller than in the PRAM model. One fundamental graph problem is connectivity. On an undirected graph with n nodes and m edges, O(log n) round connectivity algorithms have been known for over 35 years. However, no algorithms with better complexity bounds were known. In this work, we give fully scalable, faster algorithms for the connectivity problem, by parameterizing the time complexity as a function of the diameter of the graph. Our main result is a O(log D log log_m/n n) time connectivity algorithm for diameter-d graphs, using Θ(m) total memory. If our algorithm can use more memory, it can terminate in fewer rounds, and there is no lower bound on the memory per processor. We extend our results to related graph problems such as spanning forest, finding a DFS sequence, exact/approximate minimum spanning forest, and bottleneck spanning forest. We also show that achieving similar bounds for reachability in directed graphs would imply faster boolean matrix multiplication algorithms. We introduce several new algorithmic ideas. We describe a general technique called double exponential speed problem size reduction which roughly means that if we can use total memory n to reduce a problem from size n to n/k, for k=(N/n)^Θ(1) in one phase, then we can solve the problem in O(loglog_N/n n) phases. In order to achieve this fast reduction for graph connectivity, we use a multistep algorithm. One key step is a carefully constructed truncated broadcasting scheme where each node broadcasts neighbor sets to its neighbors in a way that limits the size of the resulting neighbor sets. Another key step is random leader contraction, where we choose a smaller set of leaders than many previous works do.
更多查看译文
关键词
graph connectivity,MPC model,MapReduce,parallel algorithm,diameter
AI 理解论文
溯源树
样例
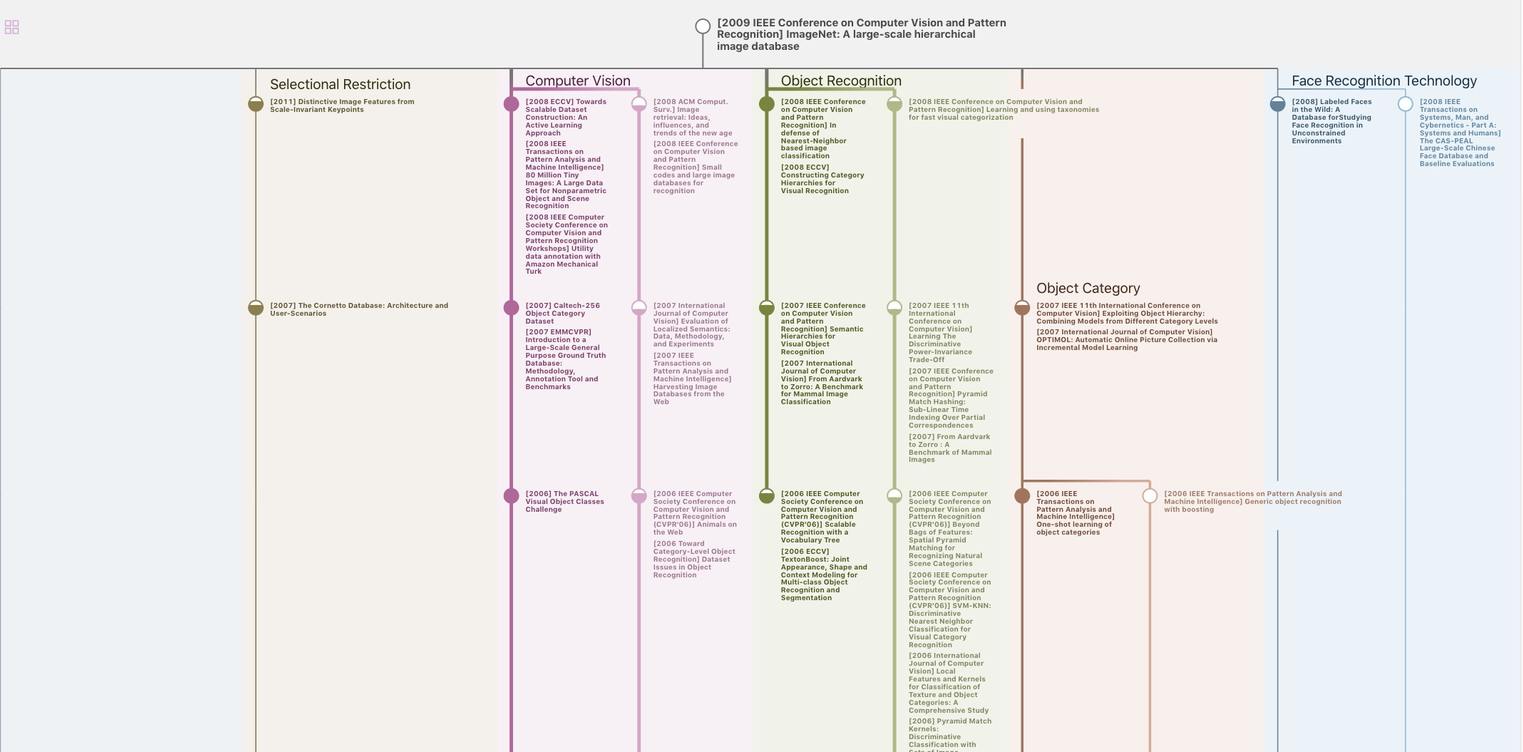
生成溯源树,研究论文发展脉络
Chat Paper
正在生成论文摘要