Measuring Congruence on High Dimensional Time Series.
arXiv: Computational Complexity(2018)
摘要
A time series is a sequence of data items; typical examples are videos, stock ticker data, or streams of temperature measurements. Quite some research has been devoted to comparing and indexing simple time series, i.e., time series where the data items are real numbers or integers. However, for many application scenarios, the data items of a time series are not simple, but high-dimensional data points. Motivated by an application scenario dealing with motion gesture recognition, we develop a distance measure (which we call congruence distance) that serves as a model for the approximate congruency of two multi-dimensional time series. This distance measure generalizes the classical notion of congruence from point sets to multi-dimensional time series. We show that, given two input time series $S$ and $T$, computing the congruence distance of $S$ and $T$ is NP-hard. Afterwards, we present two algorithms that compute an approximation of the congruence distance. We provide theoretical bounds that relate these approximations with the exact congruence distance.
更多查看译文
AI 理解论文
溯源树
样例
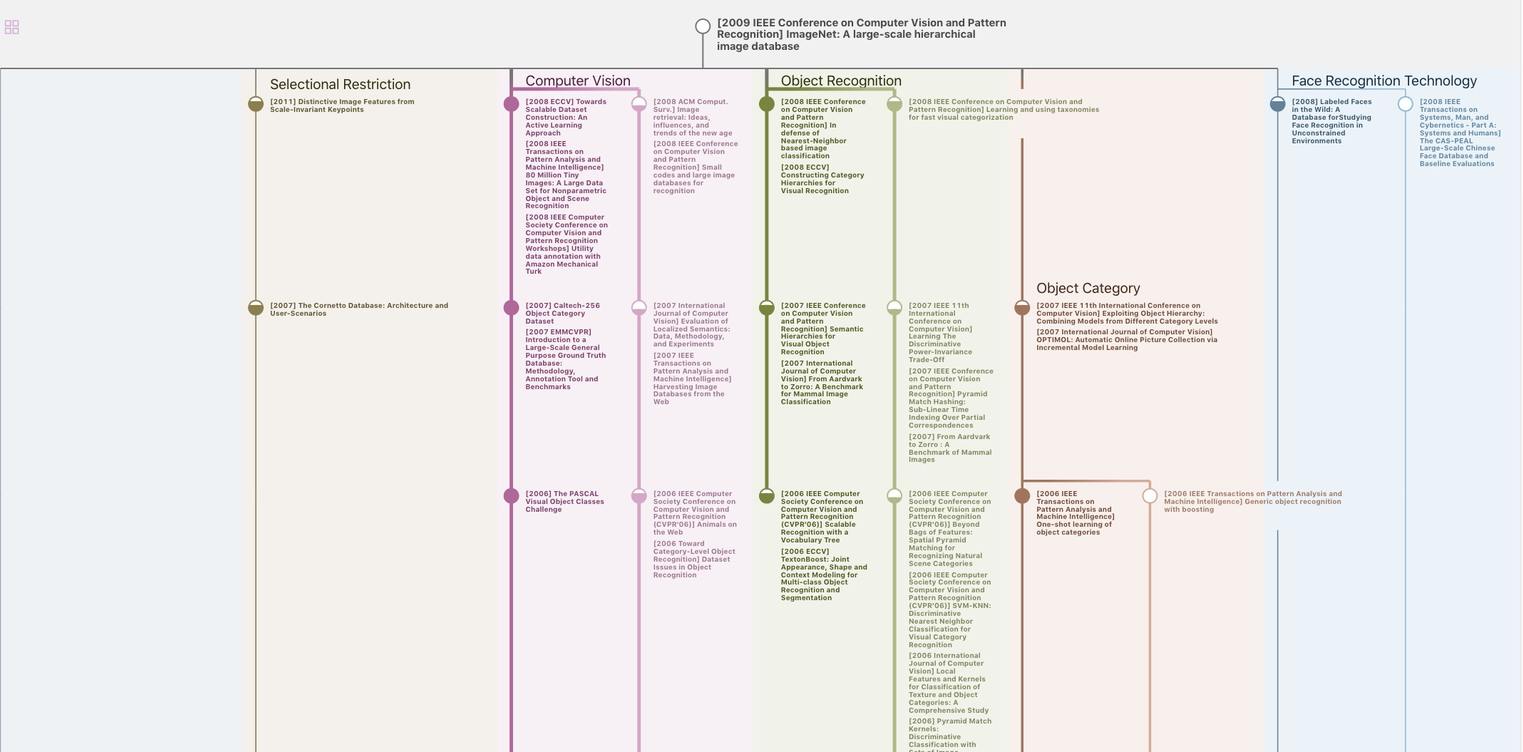
生成溯源树,研究论文发展脉络
Chat Paper
正在生成论文摘要