Intelligent Trainer for Model-Based Reinforcement Learning.
arXiv: Learning(2018)
摘要
Model-based deep reinforcement learning (DRL) is proposed as a potential solution to high sampling cost issue of DRL. However, the model quality can vary in practice, which makes it hard to pre-determine how much data we can use from the learned model and how to do the sampling. As the quality of an RL policy is largely determined by the data, the sampled data with improper setting can be a pure waste in practice and even re-using the data to re-train the policy with different parameter settings cannot help. To solve this issue, we propose a flexible reinforce on reinforce solution that can learn the optimal model-related setting on the fly. The basic unit of the framework is the model-based RL training process environment (TPE), in which a target controller communicates with the physical data and cyber data (generated by the model emulator) via state, action, and reward parameters for learning and training. On top of the TPE, we design an RL intelligent trainer to optimize the training of target controller in an online manner. This design decouples the cyber-model related settings from the training algorithms of the target controller, thus provides flexibility to implement different trainer designs. The entity of an intelligent trainer and a TPE is termed as single-head trainer, whose controller could be sensitive to cyber data quality and the action correlation could lead to performance degradation. To solve these problems we develop an ensemble trainer that consists of multi-single-head trainers and is incorporated with memory sharing, reference sampling, and weight transfer. We evaluated the proposed single-head trainer and ensemble trainer for five different tasks of OpenAI gym. The test results show that the proposed trainer method has a competitive performance with low cost, robustness, and auto-tuning.
更多查看译文
AI 理解论文
溯源树
样例
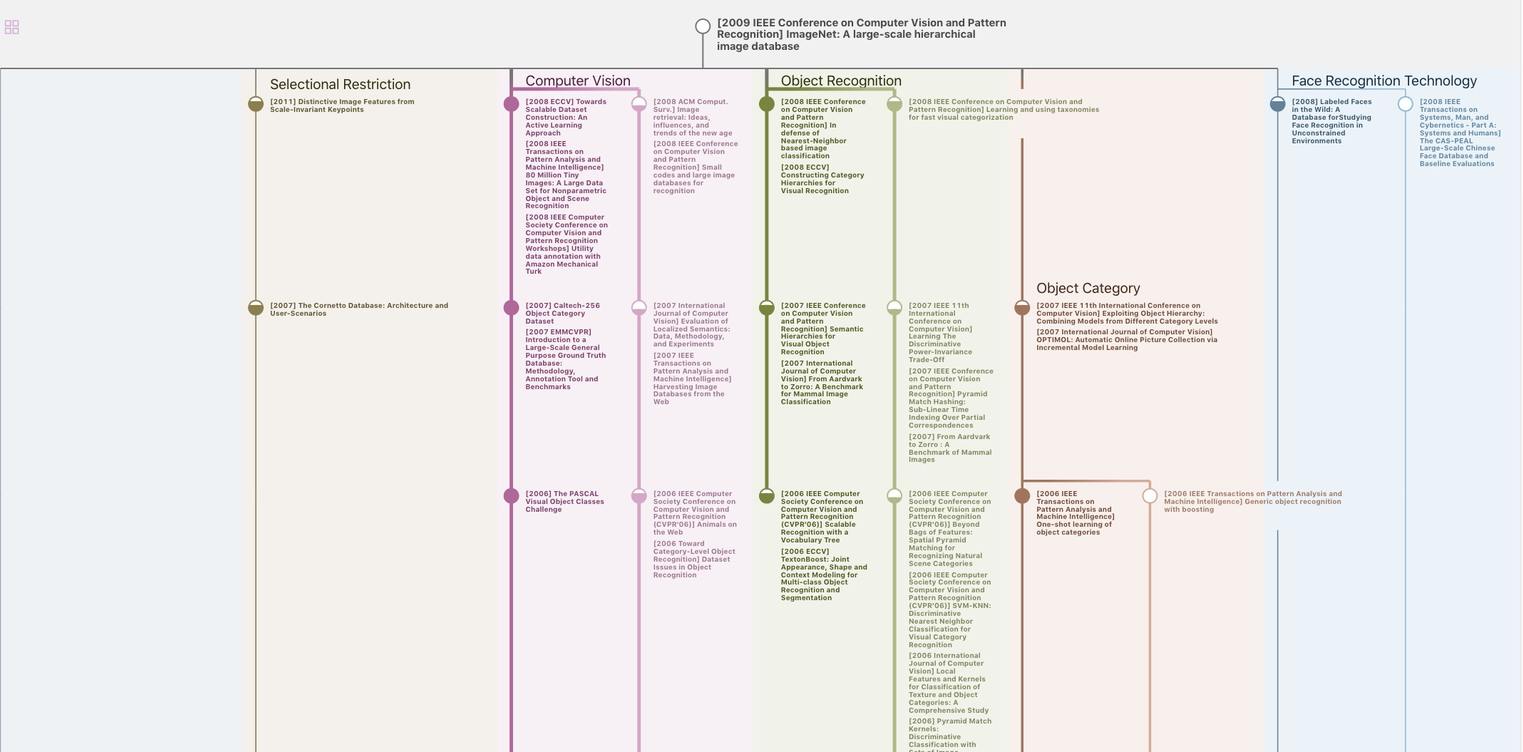
生成溯源树,研究论文发展脉络
Chat Paper
正在生成论文摘要