Quantitative Projection Coverage for Testing ML-enabled Autonomous Systems
arXiv (Cornell University)(2018)
摘要
Systematically testing models learned from neural networks remains a crucial unsolved barrier to successfully justify safety for autonomous vehicles engineered using data-driven approach. We propose quantitative k-projection coverage as a metric to mediate combinatorial explosion while guiding the data sampling process. By assuming that domain experts propose largely independent environment conditions and by associating elements in each condition with weights, the product of these conditions forms scenarios, and one may interpret weights associated with each equivalence class as relative importance. Achieving full k-projection coverage requires that the data set, when being projected to the hyperplane formed by arbitrarily selected k-conditions, covers each class with number of data points no less than the associated weight. For the general case where scenario composition is constrained by rules, precisely computing k-projection coverage remains in NP. In terms of finding minimum test cases to achieve full coverage, we present theoretical complexity for important sub-cases and an encoding to 0-1 integer programming. We have implemented a research prototype that generates test cases for a visual object defection unit in automated driving, demonstrating the technological feasibility of our proposed coverage criterion.
更多查看译文
关键词
Model Checking
AI 理解论文
溯源树
样例
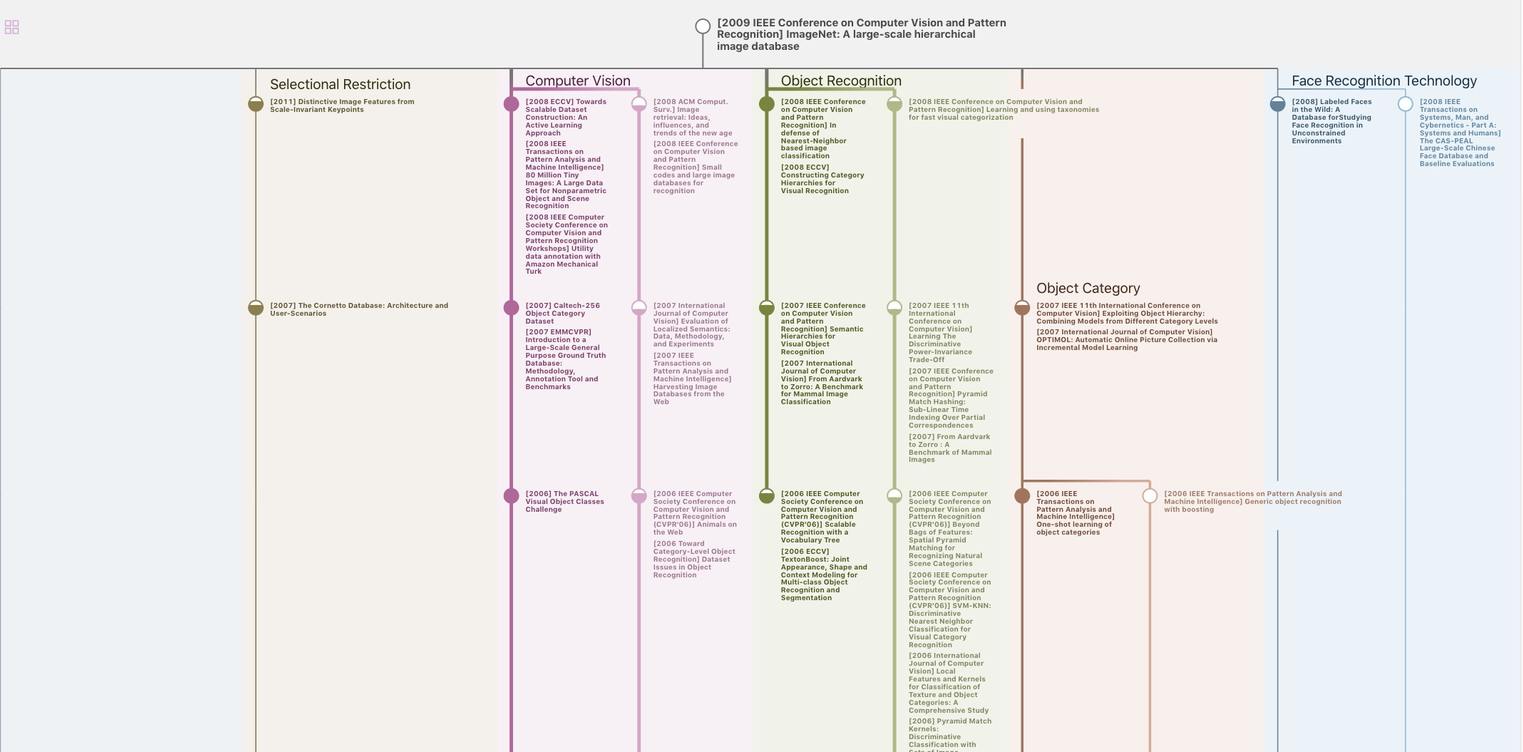
生成溯源树,研究论文发展脉络
Chat Paper
正在生成论文摘要