LF-Net: Learning Local Features from Images
ADVANCES IN NEURAL INFORMATION PROCESSING SYSTEMS 31 (NIPS 2018)(2018)
摘要
We present a novel deep architecture and a training strategy to learn a local feature pipeline from scratch, using collections of images without the need for human supervision. To do so we exploit depth and relative camera pose cues to create a virtual target that the network should achieve on one image, provided the outputs of the network for the other image. While this process is inherently non-differentiable, we show that we can optimize the network in a two-branch setup by confining it to one branch, while preserving differentiability in the other. We train our method on both indoor and outdoor datasets, with depth data from 3D sensors for the former, and depth estimates from an off-the-shelf Structure-from-Motion solution for the latter. Our models outperform the state of the art on sparse feature matching on both datasets, while running at 60+ fps for QVGA images.
更多查看译文
关键词
deep neural networks,optimal solution,the state of the art,feature learning,state of art,correspondence problem
AI 理解论文
溯源树
样例
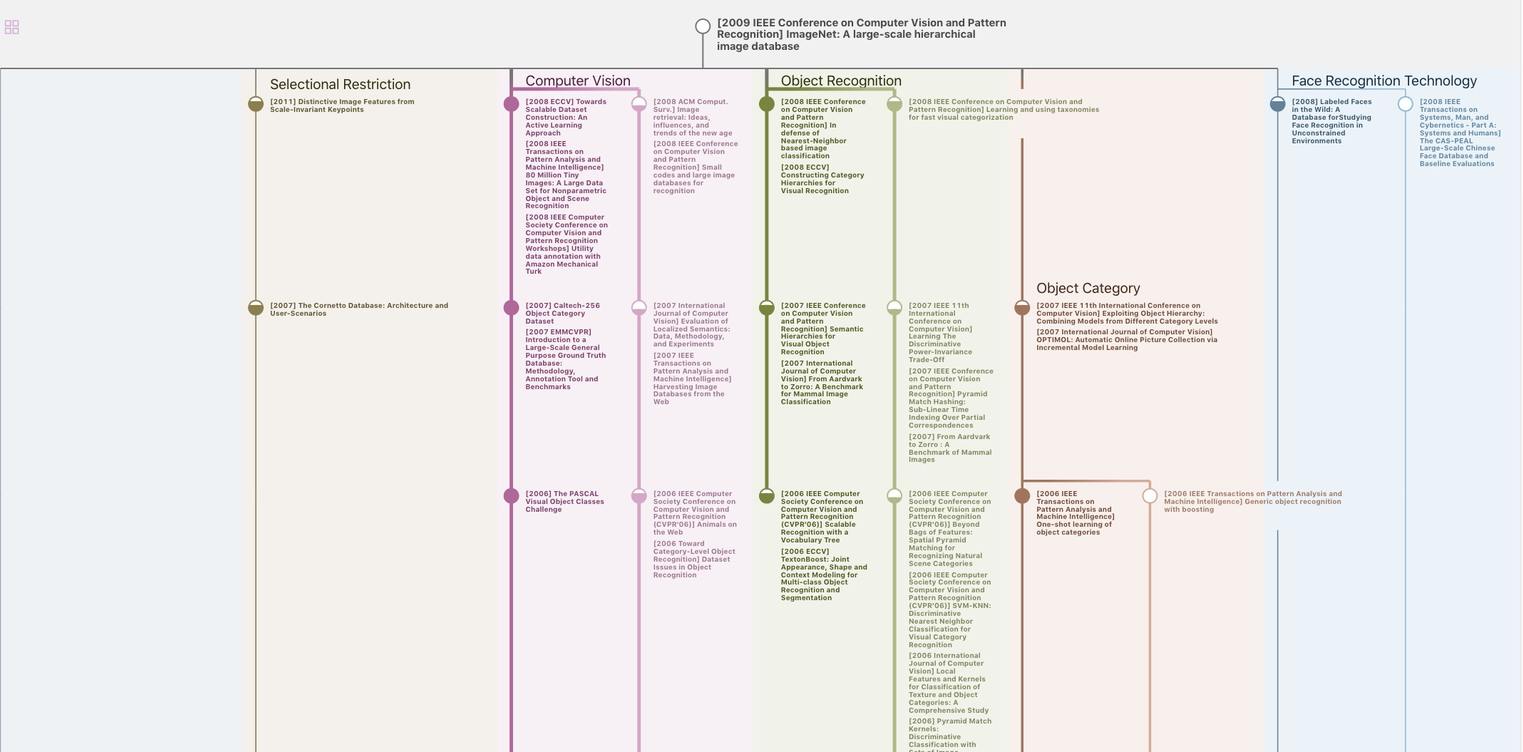
生成溯源树,研究论文发展脉络
Chat Paper
正在生成论文摘要