Semi-Supervised Deep Kernel Learning: Regression With Unlabeled Data By Minimizing Predictive Variance
ADVANCES IN NEURAL INFORMATION PROCESSING SYSTEMS 31 (NIPS 2018)(2018)
摘要
Large amounts of labeled data are typically required to train deep learning models. For many real-world problems, however, acquiring additional data can be expensive or even impossible. We present semi-supervised deep kernel learning (SSDKL), a semi-supervised regression model based on minimizing predictive variance in the posterior regularization framework. SSDKL combines the hierarchical representation learning of neural networks with the probabilistic modeling capabilities of Gaussian processes. By leveraging unlabeled data, we show improvements on a diverse set of real-world regression tasks over supervised deep kernel learning and semi-supervised methods such as VAT and mean teacher adapted for regression.
更多查看译文
关键词
neural networks,gaussian processes,labeled data
AI 理解论文
溯源树
样例
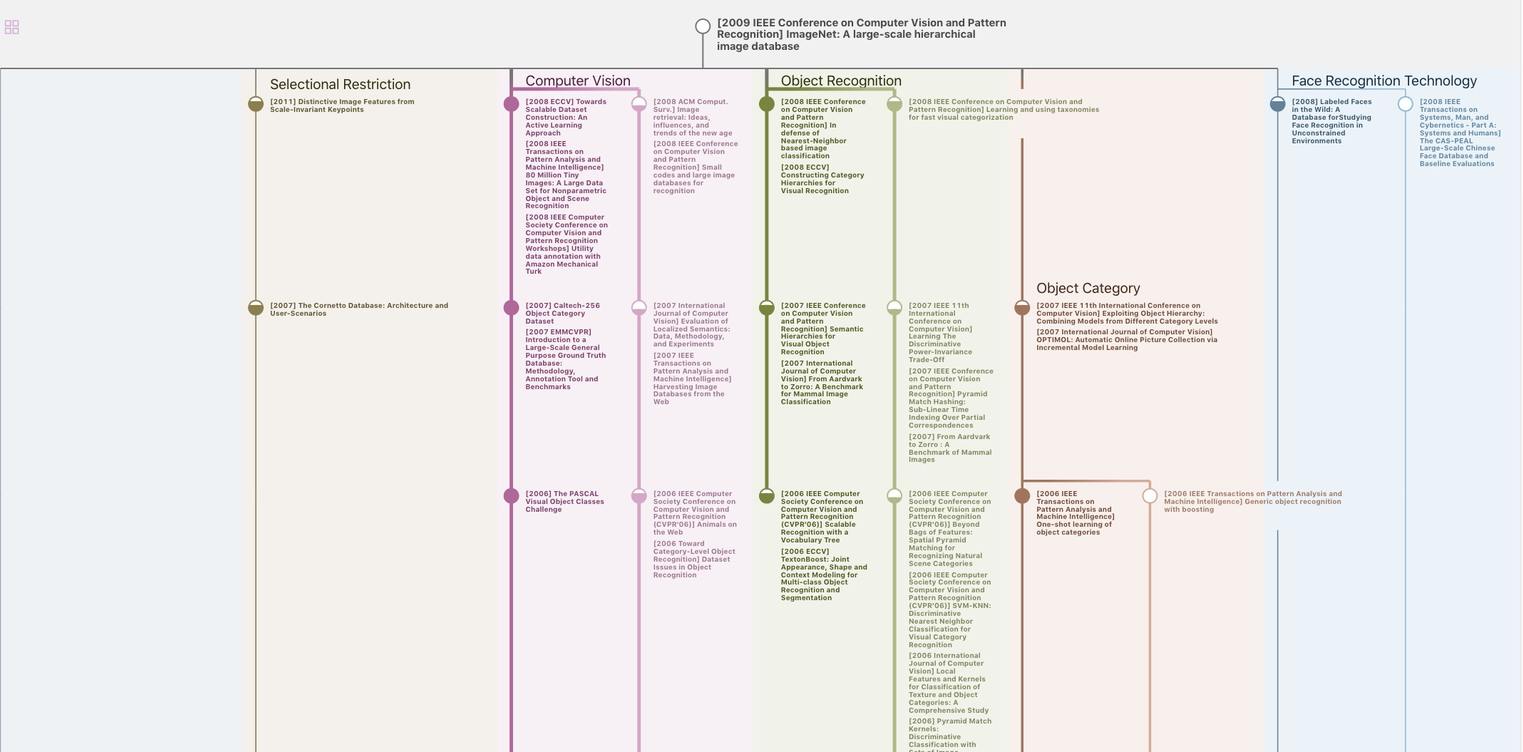
生成溯源树,研究论文发展脉络
Chat Paper
正在生成论文摘要