Object Localization and Motion Transfer learning with Capsules.
arXiv: Computer Vision and Pattern Recognition(2018)
摘要
Inspired by CapsNetu0027s routing-by-agreement mechanism, with its ability to learn object properties, and by center-of-mass calculations from physics, we propose a CapsNet architecture with object coordinate atoms and an LSTM network for evaluation. The first is based on CapsNet but uses a new routing algorithm to find the objectsu0027 approximate positions in the image coordinate system, and the second is a parameterized affine transformation network that can predict future positions from past positions by learning the translation transformation from 2D object coordinates generated from the first network. We demonstrate the learned translation transformation is transferable to another dataset without the need to train the transformation network again. Only the CapsNet needs training on the new dataset. As a result, our work shows that object recognition and motion prediction can be separated, and that motion prediction can be transferred to another dataset with different object types.
更多查看译文
AI 理解论文
溯源树
样例
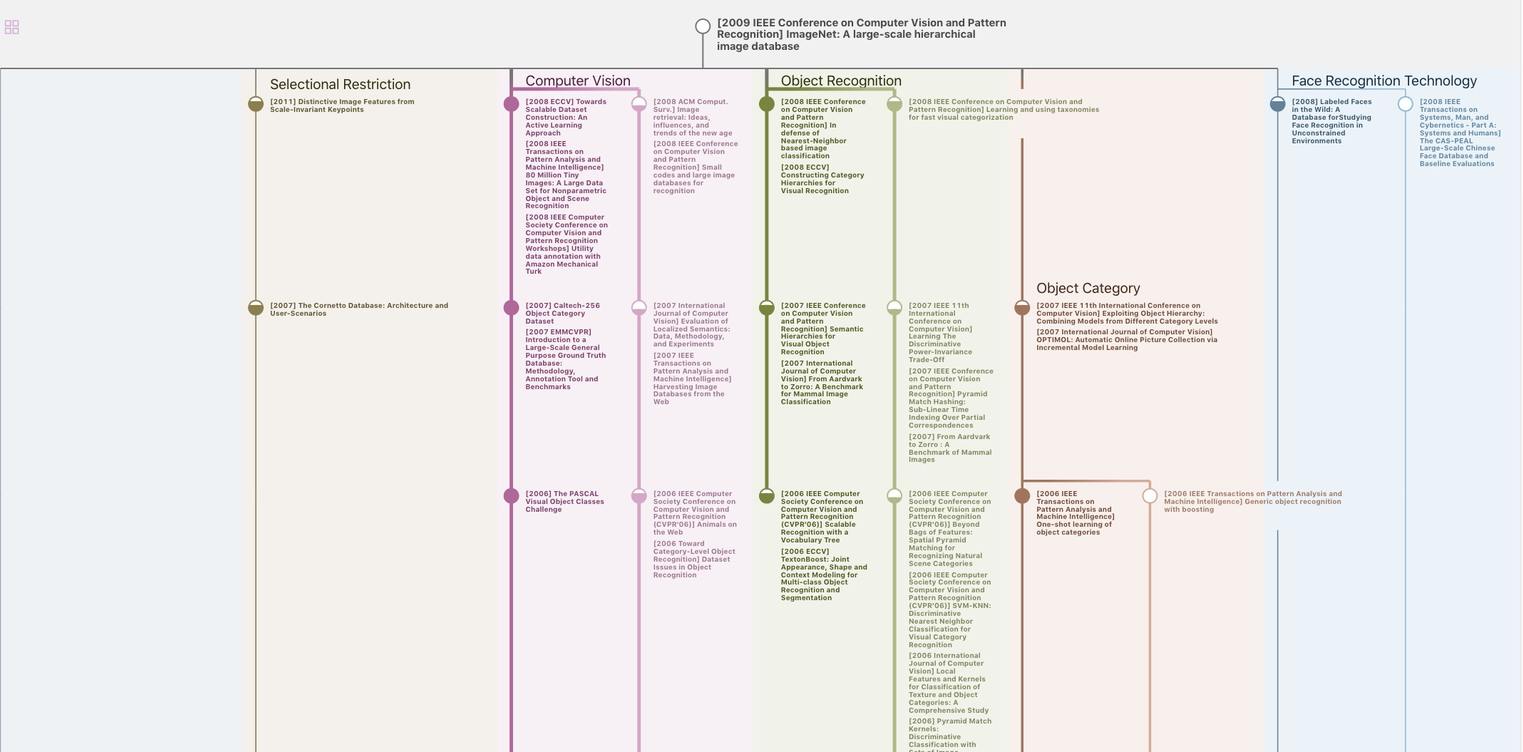
生成溯源树,研究论文发展脉络
Chat Paper
正在生成论文摘要