Synthetically Trained Neural Networks for Learning Human-Readable Plans from Real-World Demonstrations
2018 IEEE International Conference on Robotics and Automation (ICRA)(2018)
摘要
We present a system to infer and execute a human-readable program from a real-world demonstration. The system consists of a series of neural networks to perform perception, program generation, and program execution. Leveraging convolutional pose machines, the perception network reliably detects the bounding cuboids of objects in real images even when severely occluded, after training only on synthetic images using domain randomization. To increase the applicability of the perception network to new scenarios, the network is formulated to predict in image space rather than in world space. Additional networks detect relationships between objects, generate plans, and determine actions to reproduce a real-world demonstration. The networks are trained entirely in simulation, and the system is tested in the real world on the pick-and-place problem of stacking colored cubes using a Baxter robot.
更多查看译文
关键词
convolutional pose machines,human-readable plans learning,domain randomization,Baxter robot,image space,synthetic images,perception network,program execution,program generation,human-readable program,synthetically trained neural networks,world space
AI 理解论文
溯源树
样例
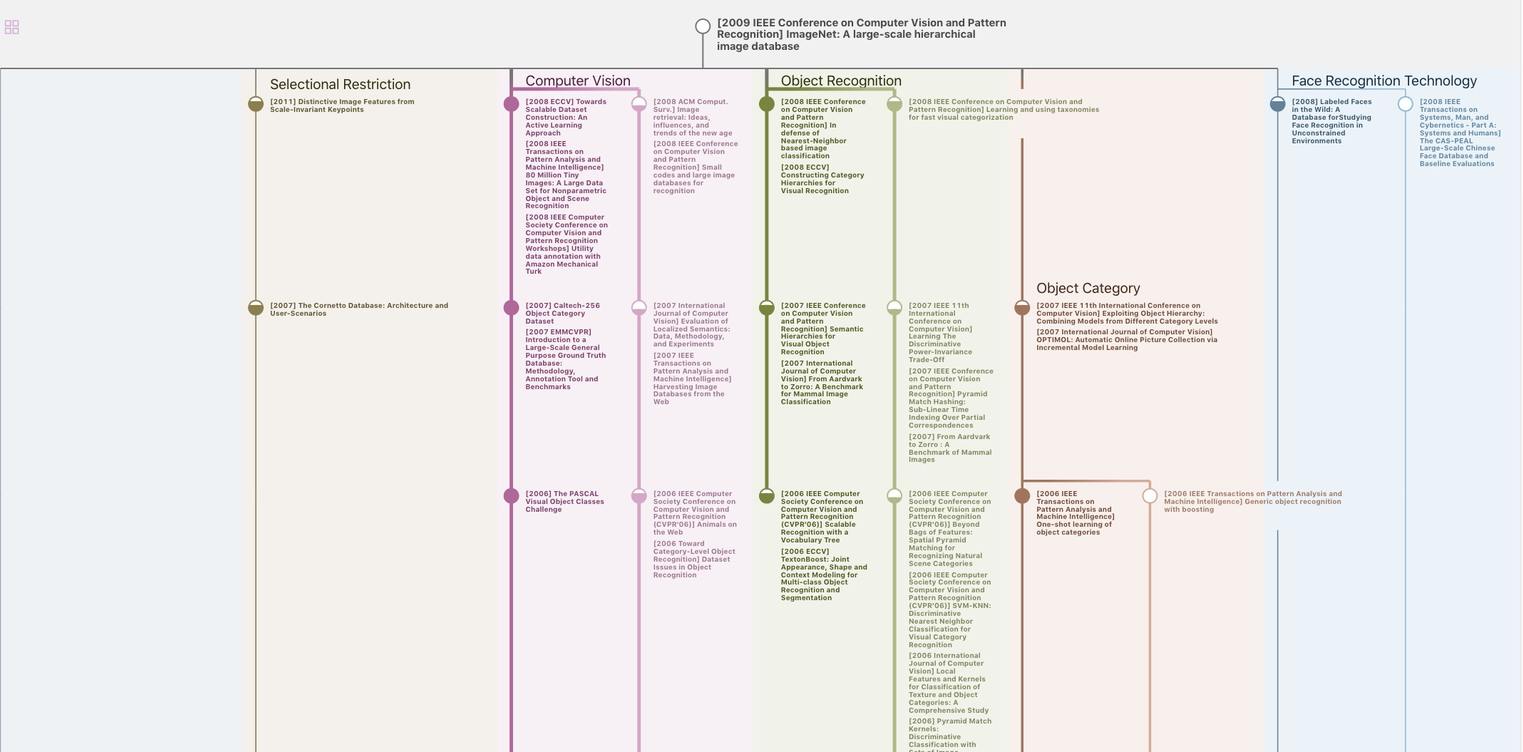
生成溯源树,研究论文发展脉络
Chat Paper
正在生成论文摘要