Deep Dynamical Modeling and Control of Unsteady Fluid Flows.
ADVANCES IN NEURAL INFORMATION PROCESSING SYSTEMS 31 (NIPS 2018)(2018)
摘要
The design of flow control systems remains a challenge due to the nonlinear nature of the equations that govern fluid flow. However, recent advances in computational fluid dynamics (CFD) have enabled the simulation of complex fluid flows with high accuracy, opening the possibility of using learning-based approaches to facilitate controller design. We present a method for learning the forced and unforced dynamics of airflow over a cylinder directly from CFD data. The proposed approach, grounded in Koopman theory, is shown to produce stable dynamical models that can predict the time evolution of the cylinder system over extended time horizons. Finally, by performing model predictive control with the learned dynamical models, we are able to find a straightforward, interpretable control law for suppressing vortex shedding in the wake of the cylinder.
更多查看译文
关键词
control law,complex fluid,control systems,time evolution,computational fluid dynamics,the simulation,fluid flows,model predictive control,vortex shedding
AI 理解论文
溯源树
样例
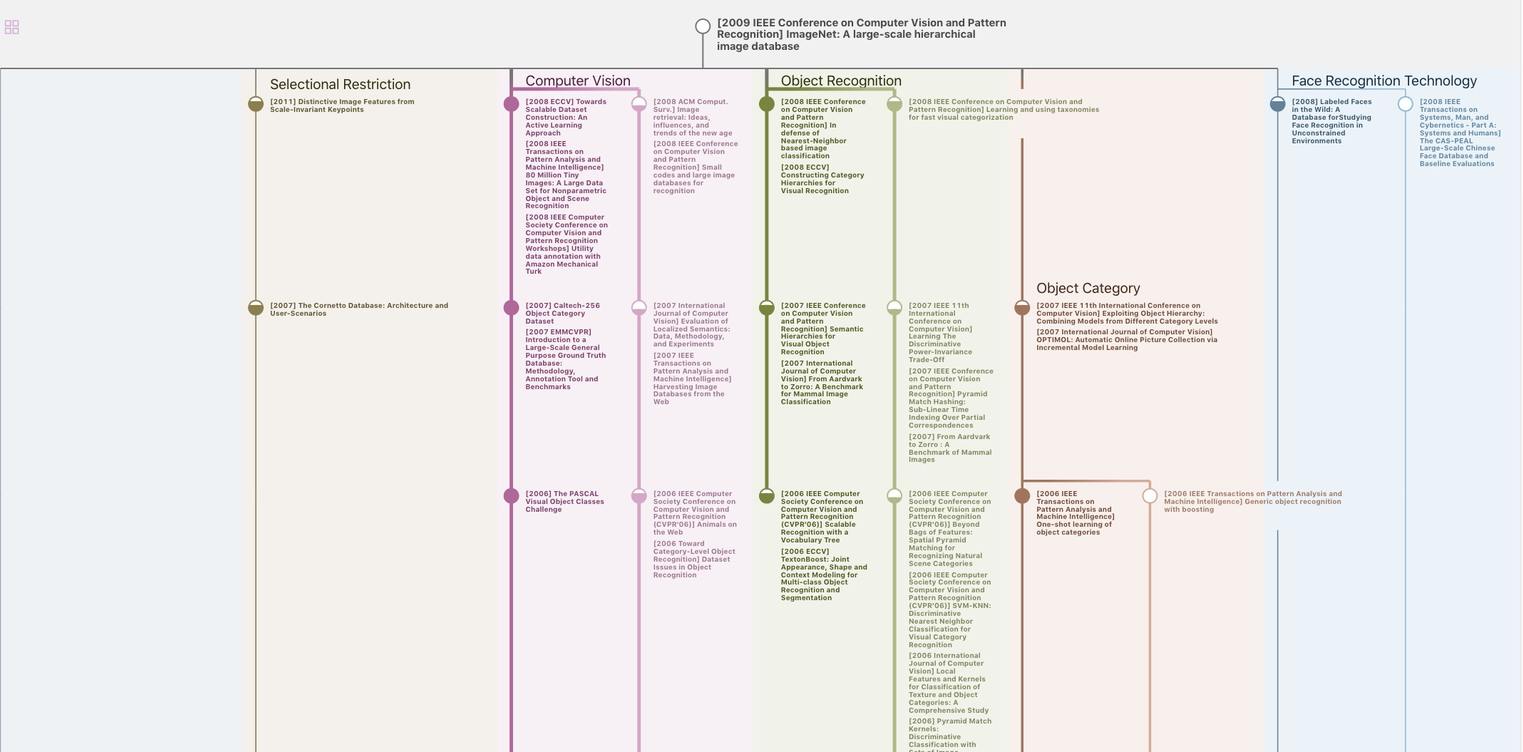
生成溯源树,研究论文发展脉络
Chat Paper
正在生成论文摘要