Heuristically Accelerated Reinforcement Learning by Means of Case-Based Reasoning and Transfer Learning
Journal of Intelligent and Robotic Systems(2017)
摘要
Reinforcement Learning (RL) is a well-known technique for learning the solutions of control problems from the interactions of an agent in its domain. However, RL is known to be inefficient in problems of the real-world where the state space and the set of actions grow up fast. Recently, heuristics, case-based reasoning (CBR) and transfer learning have been used as tools to accelerate the RL process. This paper investigates a class of algorithms called Transfer Learning Heuristically Accelerated Reinforcement Learning (TLHARL) that uses CBR as heuristics within a transfer learning setting to accelerate RL. The main contributions of this work are the proposal of a new TLHARL algorithm based on the traditional RL algorithm Q ( λ ) and the application of TLHARL on two distinct real-robot domains: a robot soccer with small-scale robots and the humanoid-robot stability learning. Experimental results show that our proposed method led to a significant improvement of the learning rate in both domains.
更多查看译文
关键词
Reinforcement learning,Transfer learning,Case-based reasoning,Robotics
AI 理解论文
溯源树
样例
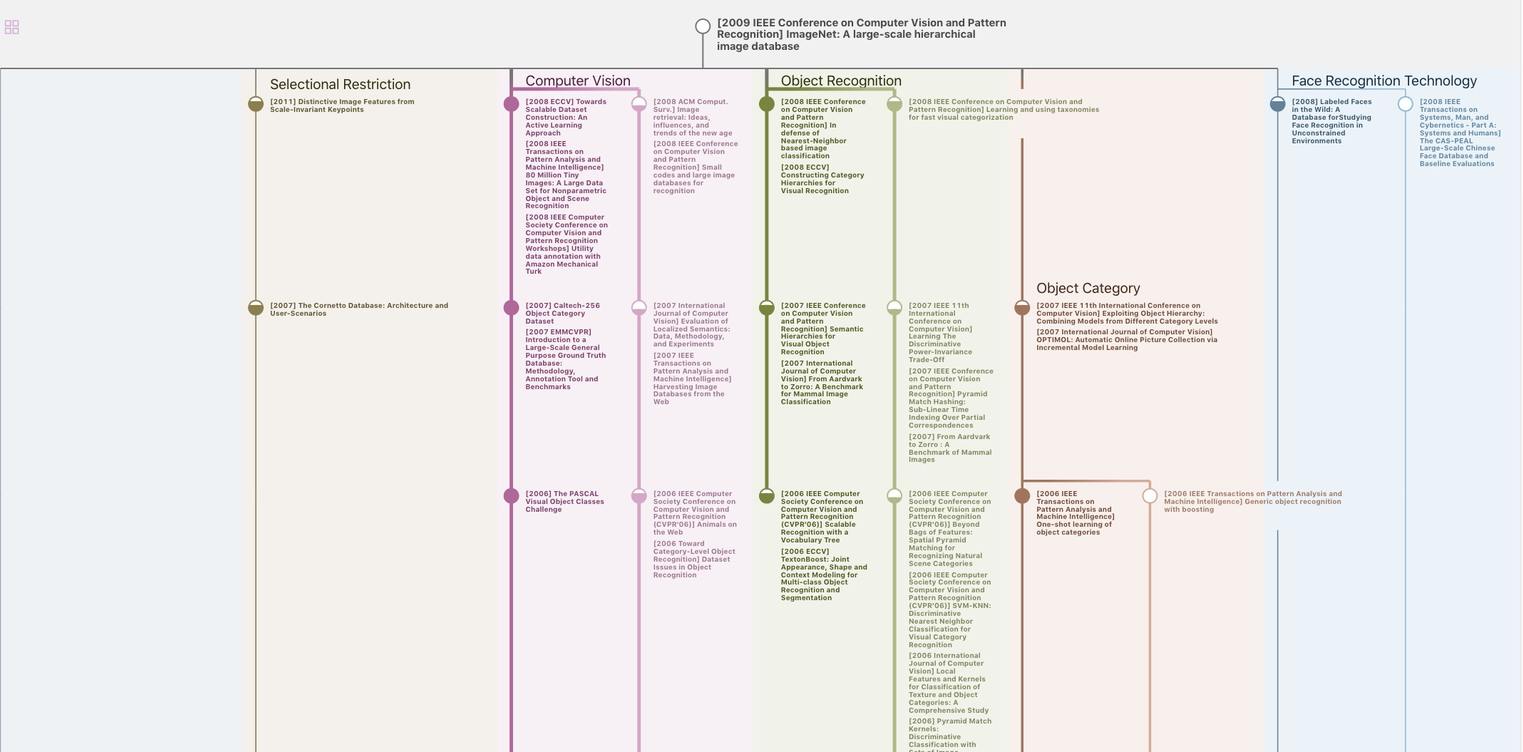
生成溯源树,研究论文发展脉络
Chat Paper
正在生成论文摘要