Evaluation of Decoding Algorithms for Estimating Bladder Pressure from Dorsal Root Ganglia Neural Recordings
Annals of biomedical engineering(2017)
摘要
A closed-loop device for bladder control may offer greater clinical benefit compared to current open-loop stimulation devices. Previous studies have demonstrated the feasibility of using single-unit recordings from sacral-level dorsal root ganglia (DRG) for decoding bladder pressure. Automatic online sorting, to differentiate single units, can be computationally heavy and unreliable, in contrast to simple multi-unit thresholded activity. In this study, the feasibility of using DRG multi-unit recordings to decode bladder pressure was examined. A broad range of feature selection methods and three algorithms (multivariate linear regression, basic Kalman filter, and a nonlinear autoregressive moving average model) were used to create training models and provide validation fits to bladder pressure for data collected in seven anesthetized feline experiments. A non-linear autoregressive moving average (NARMA) model with regularization provided the most accurate bladder pressure estimate, based on normalized root-mean-squared error, NRMSE, (17 ± 7%). A basic Kalman filter yielded the highest similarity to the bladder pressure with an average correlation coefficient, CC, of 0.81 ± 0.13. The best algorithm set (based on NRMSE) was further evaluated on data obtained from a chronic feline experiment. Testing results yielded a NRMSE and CC of 10.7% and 0.61, respectively from a model that was trained on data recorded 2 weeks prior. From offline analysis, implementation of NARMA in a closed-loop scheme for detecting bladder contractions would provide a robust control signal. Ultimate integration of closed-loop algorithms in bladder neuroprostheses will require evaluations of parameter and signal stability over time.
更多查看译文
关键词
Neural network,Kalman filter,Microelectrode,Lower urinary tract,Bladder,Dorsal root ganglia,DRG
AI 理解论文
溯源树
样例
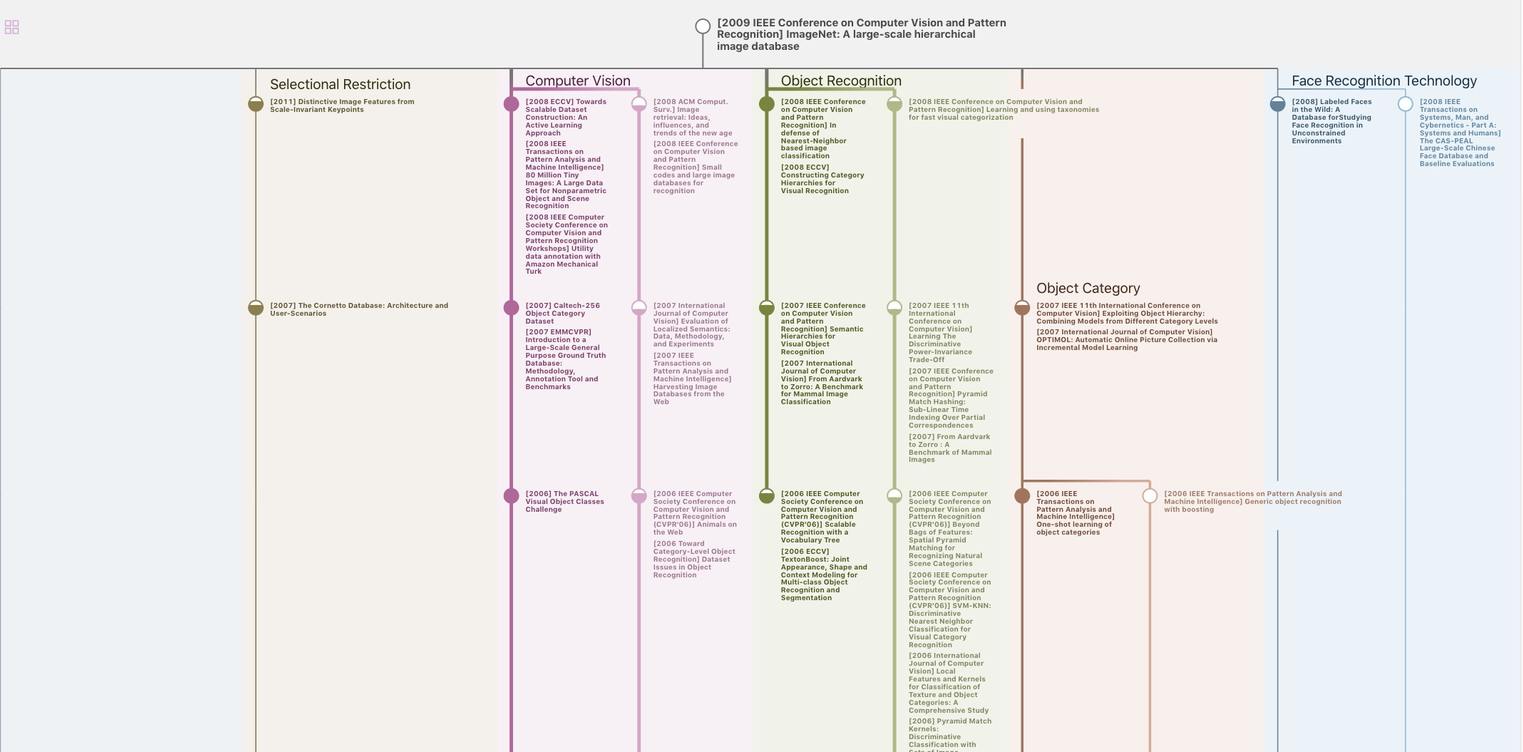
生成溯源树,研究论文发展脉络
Chat Paper
正在生成论文摘要