A multiresolution ensemble Kalman filter using the wavelet decomposition
Computational Geosciences(2017)
摘要
We present a method of using classical wavelet-based multiresolution analysis to separate scales in model and observations during data assimilation with the ensemble Kalman filter. In many applications, the underlying physics of a phenomena involve the interaction of features at multiple scales. Blending of observational and model error across scales can result in large forecast inaccuracies since large errors at one scale are interpreted as inexact data at all scales due to the misrepresentation of observational error. Our method uses a partitioning of the range of the observation operator into separate observation scales. This naturally induces a transformation of the observation covariance and we put forward several algorithms to efficiently compute the transformed covariance. Another advantage of our multiresolution ensemble Kalman filter is that scales can be weighted independently to adjust each scale’s affect on the forecast. To demonstrate feasibility, we present applications to a one-dimensional Kuramoto-Sivashinsky (K–S) model with scale-dependent observation noise and an application involving the forecasting of solar photospheric flux. The solar flux application uses the Air Force Data Assimilative Photospheric Transport (ADAPT) model which has model and observation error exhibiting strong scale dependence. Results using our multiresolution ensemble Kalman filter show significant improvement in solar forecast error compared to traditional ensemble Kalman filtering.
更多查看译文
关键词
Data assimilation,Wavelets,Multiresolution analysis,Ensemble Kalman filter,86A22,62L12,60-08
AI 理解论文
溯源树
样例
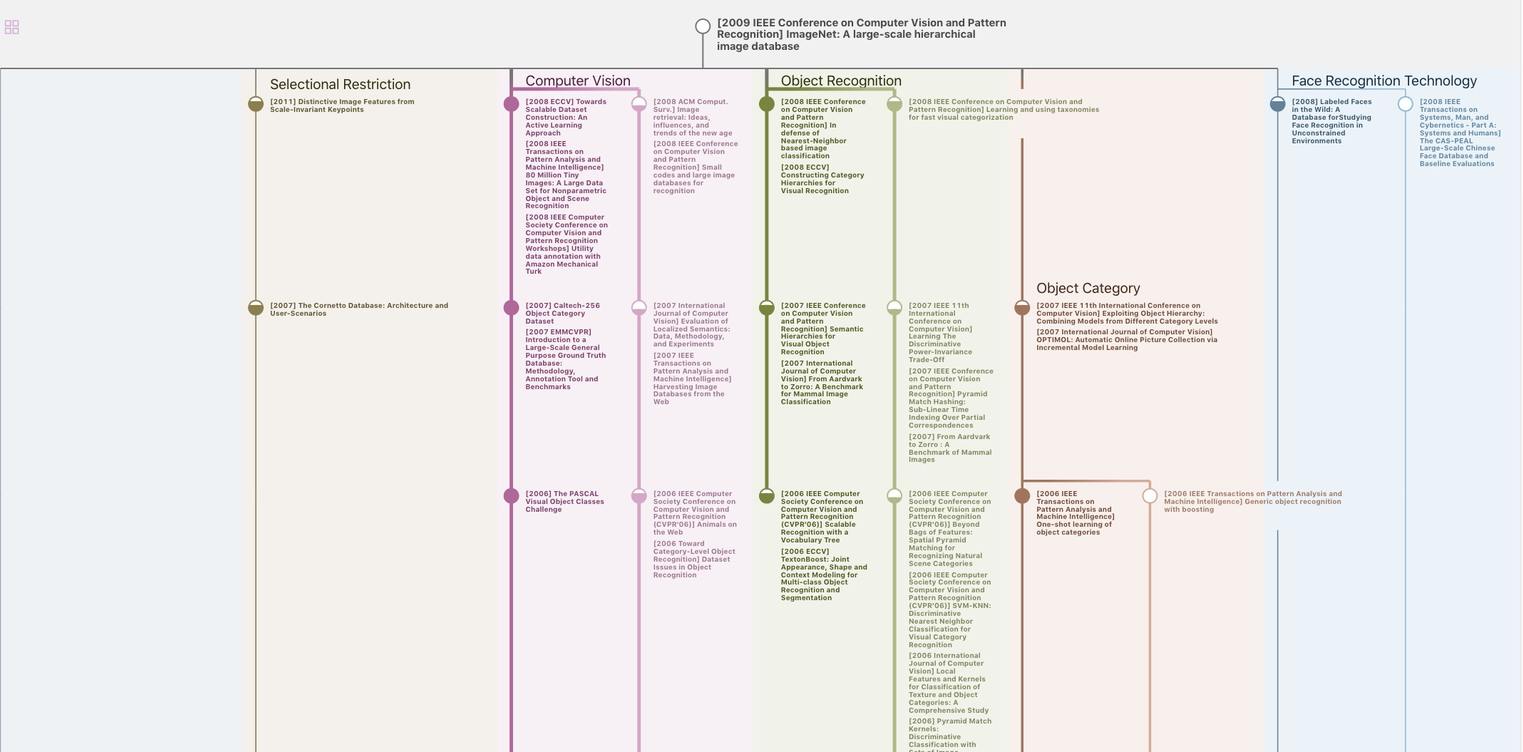
生成溯源树,研究论文发展脉络
Chat Paper
正在生成论文摘要