Scheduling data-intensive scientific workflows with reduced communication.
SSDBM(2018)
摘要
Data-intensive scientific workflows, typically modelled by directed acyclic graphs, consist of inter-dependent tasks that exchange significant amounts of data and are executed on parallel/distributed clusters. However, the energy or monetary costs associated with large data transfers between tasks executing on different nodes may be significant. As a result, there is scope to explore the possibility of trading some communication for computation, aiming to reduce overall communication costs. In this work, we propose a scheduling approach that scales the weight of communication to increase its impact when building the schedule of a scientific workflow; the aim is to assign pairs of tasks with significant data transfers to the same computational node so that the overall communication cost is minimized. The proposed approach is evaluated using simulation and three real-world scientific workflows. The tradeoff between scientific workflow execution time and the size of data transfers is assessed for different weights and a different number of computational nodes.
更多查看译文
关键词
Data-intensive workflows, workflow scheduling, communication
AI 理解论文
溯源树
样例
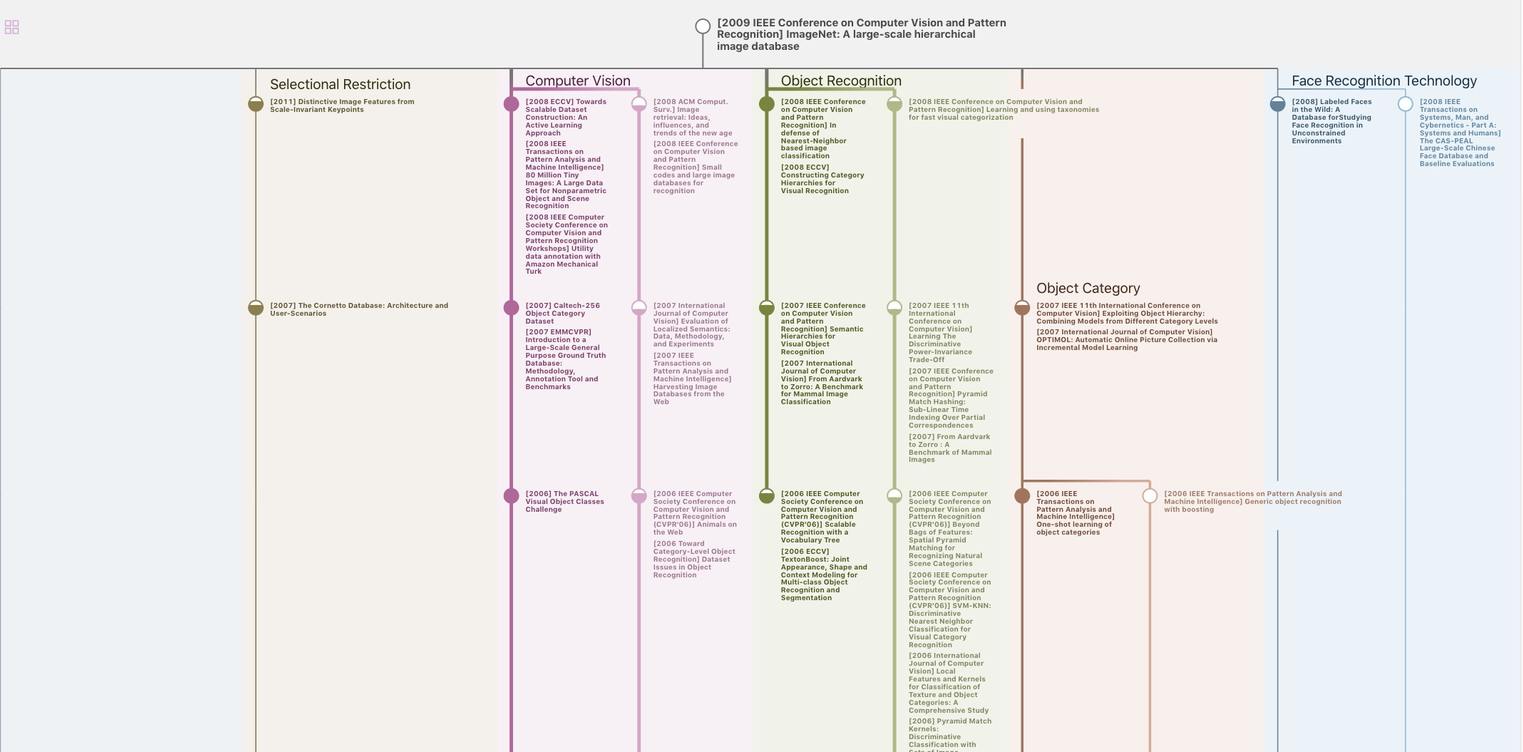
生成溯源树,研究论文发展脉络
Chat Paper
正在生成论文摘要